Solving task scheduling problems in cloud manufacturing via attention mechanism and deep reinforcement learning
Journal of Manufacturing Systems(2022)
摘要
Cloud manufacturing (CMfg) offers a cloud platform for both consumers and providers, and allocating consumer tasks to service providers requires many-to-many scheduling. Deep reinforcement learning (DRL) has been gradually employed to solve CMfg scheduling problems and has achieved satisfactory performance in dynamic and uncertain cloud environments. Nonetheless, we need better scheduling algorithms and more accessible modeling methods to enable practical implementation. In this study, an end-to-end scheduling algorithm is proposed to address task scheduling problems in CMfg. The proposal extracts intercorrelations in enterprise–enterprise and enterprise–task with the multi-head attention mechanism and is trained by DRL. Our proposal has extremely low time-response compared to heuristic algorithms and can generate a scheduling solution in seconds. In contrast to other DRL algorithms, our proposal can achieve better scheduling performances and uses a more accessible modeling method: the objective function alone is sufficient to train the model stably, without the need for a step-based reward function. Applying multi-head attention and DRL to scheduling problems is an exploratory attempt to achieve positive results. Experimental results on a case of automobile structure part processing in CMfg indicated that our proposal showed competitive scheduling performances and running time compared to eight DRL algorithms, two heuristic algorithms, and two priority dispatching rules. Besides, the results proved that our proposal’s generalizability and scalability were better than the other eight DRL algorithms.
更多查看译文
关键词
Scheduling problem,Deep reinforcement learning,Transformer,Multi-head attention,Cloud manufacturing
AI 理解论文
溯源树
样例
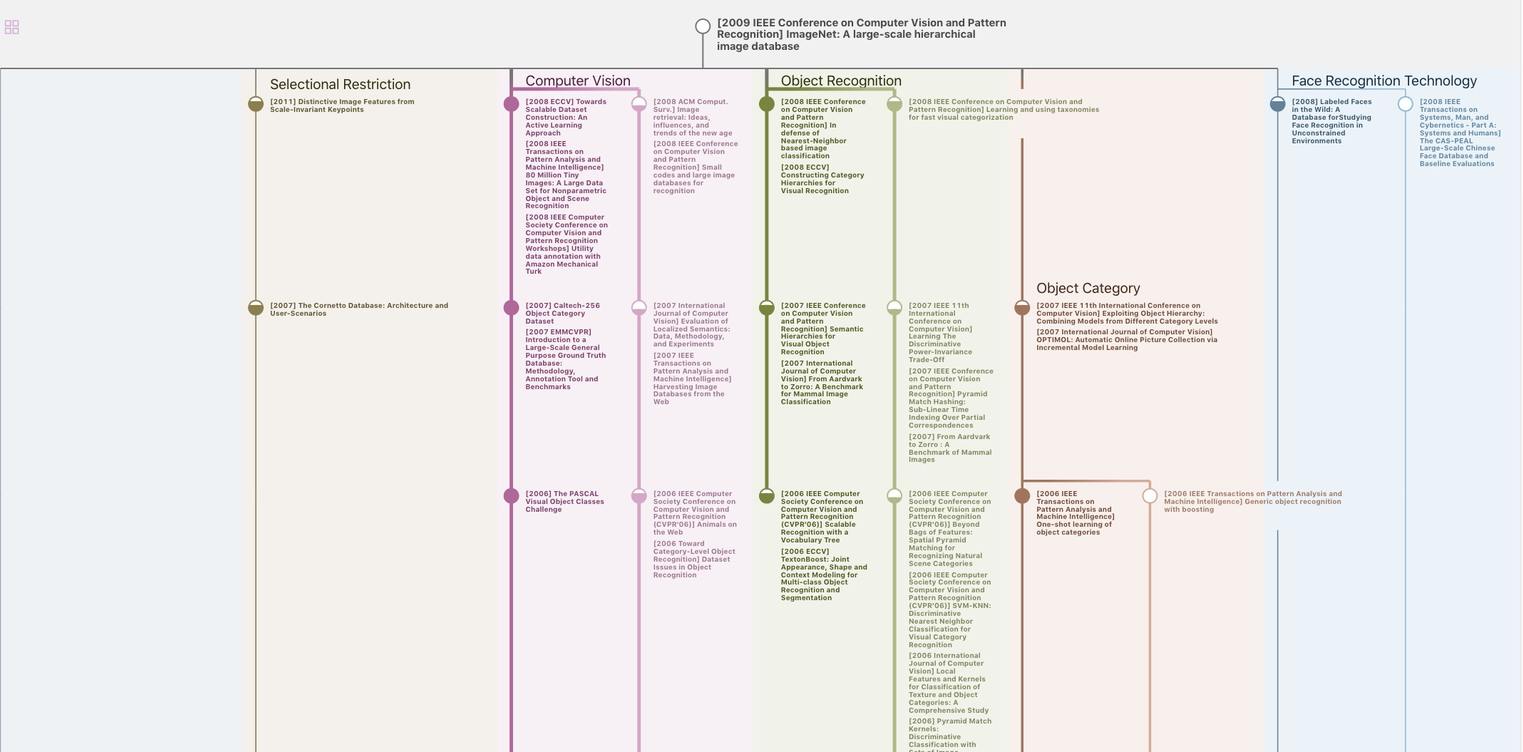
生成溯源树,研究论文发展脉络
Chat Paper
正在生成论文摘要