Dymola-Enabled Reinforcement Learning for Real-time Generator Set-point Optimization
2022 IEEE Power & Energy Society Innovative Smart Grid Technologies Conference (ISGT)(2022)
摘要
This paper introduces a reinforcement learning framework which determines real-time generator set-points in a dynamically changing environment in order to optimize a chosen objective. This is performed within the high-fidelity simulation environment Dymola, which utilizes the Modelica programming language to model complex systems. A case study is created using the OpenIPSL IEEE 9-bus dynamic model, with the objective of minimizing voltage deviations across the network. The reinforcement learning agent shows improvement in minimizing voltage deviations versus the default droop controlled governors without any explicit knowledge of the topology of the system or relative location of the controllers. The results indicate that reinforcement learning may be a useful tool for applications in model-free, real-time power systems dynamics and control. An open-source Python package is provided for the proposed framework with the present case-study as an example.
更多查看译文
关键词
Modelica,voltage regulation,unsupervised learning
AI 理解论文
溯源树
样例
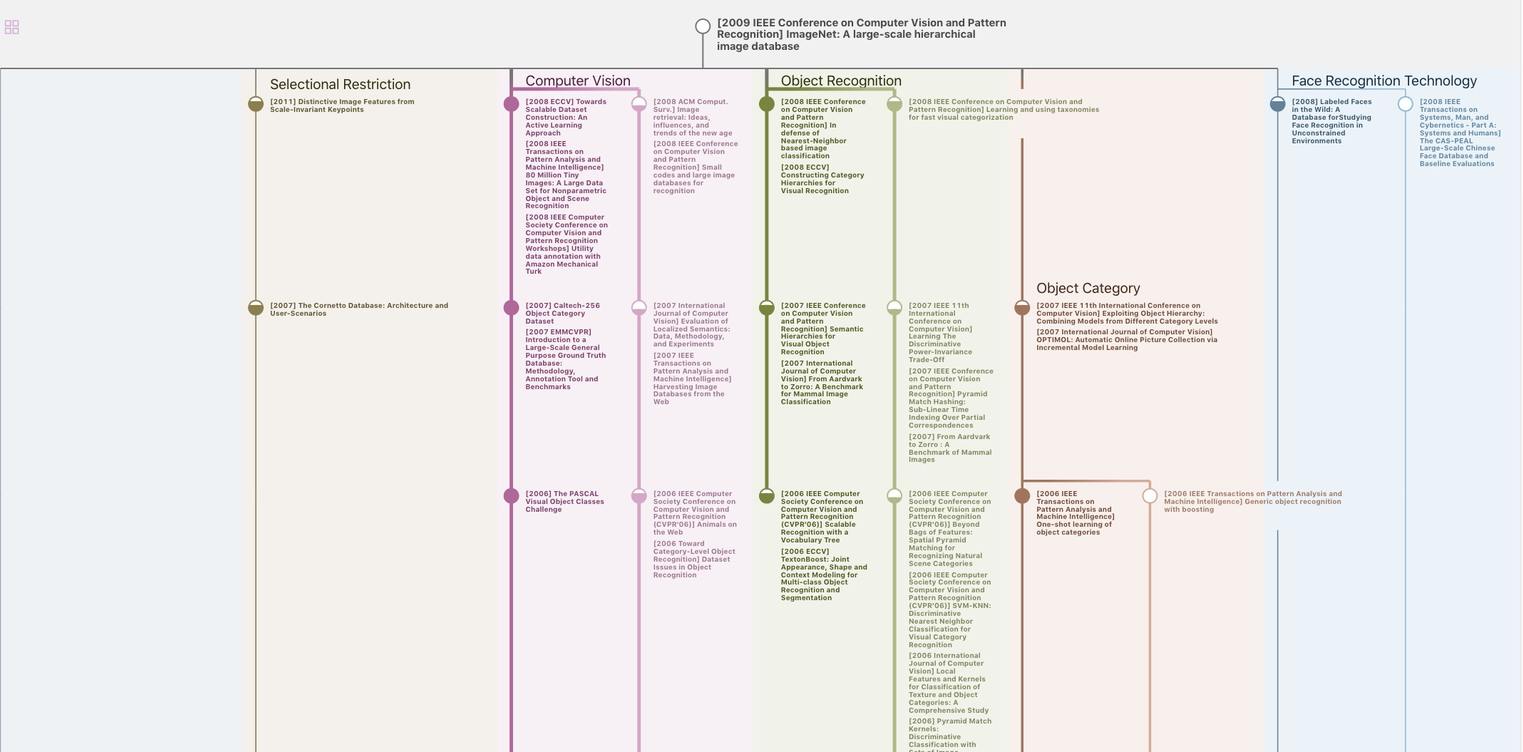
生成溯源树,研究论文发展脉络
Chat Paper
正在生成论文摘要