On convergence of a q-random coordinate constrained algorithm for non-convex problems
arXiv (Cornell University)(2022)
摘要
We propose a random coordinate descent algorithm for optimizing a non-convex
objective function subject to one linear constraint and simple bounds on the
variables. Although it is common use to update only two random coordinates
simultaneously in each iteration of a coordinate descent algorithm, our
algorithm allows updating arbitrary number of coordinates. We provide a proof
of convergence of the algorithm. The convergence rate of the algorithm improves
when we update more coordinates per iteration. Numerical experiments on large
scale instances of different optimization problems show the benefit of updating
many coordinates simultaneously.
更多查看译文
关键词
non-convex
AI 理解论文
溯源树
样例
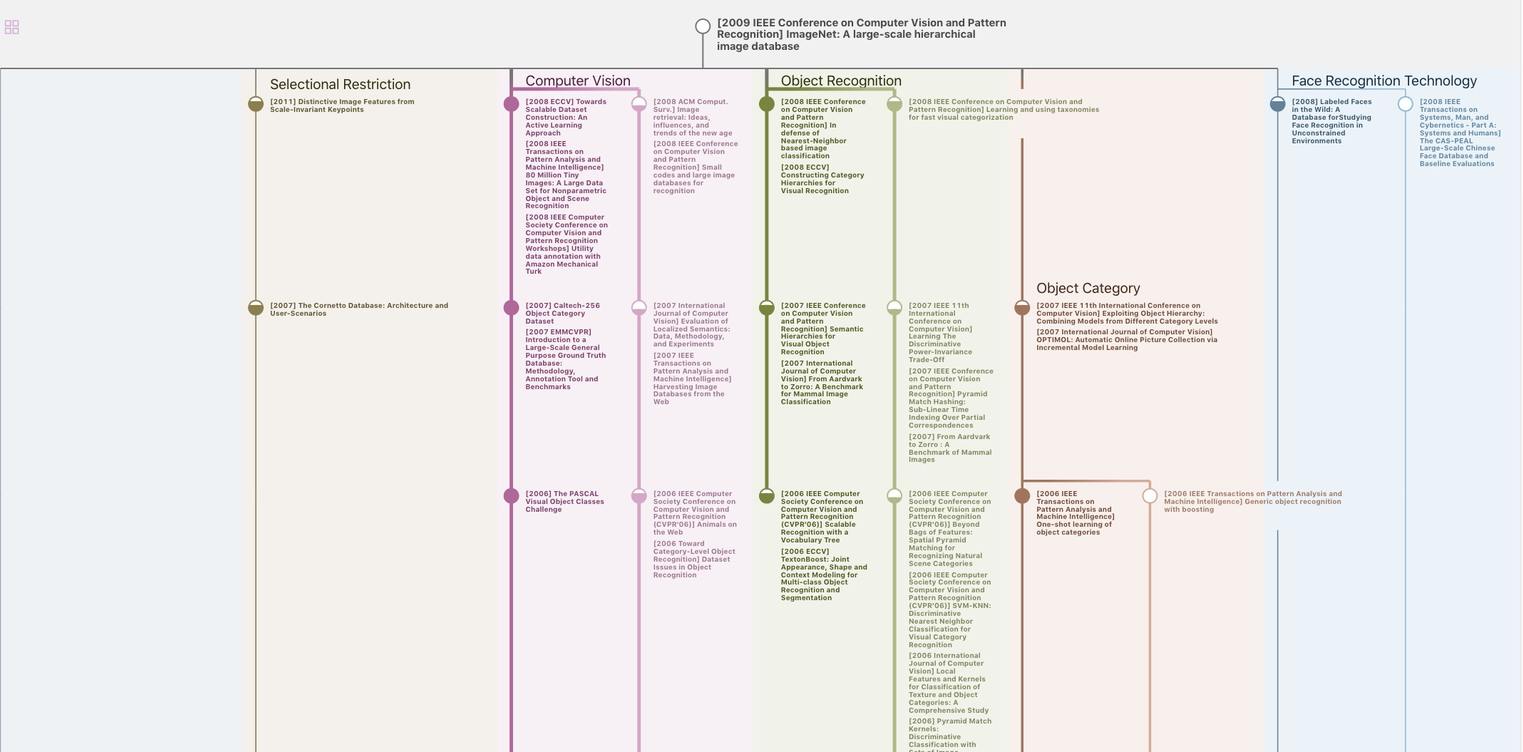
生成溯源树,研究论文发展脉络
Chat Paper
正在生成论文摘要