CPS-MEBR: Click Feedback-Aware Web Page Summarization for Multi-Embedding-Based Retrieval
arxiv(2022)
摘要
Embedding-based retrieval (EBR) is a technique to use embeddings to represent query and document, and then convert the retrieval problem into a nearest neighbor search problem in the embedding space. Some previous works have mainly focused on representing the web page with a single embedding, but in real web search scenarios, it is difficult to represent all the information of a long and complex structured web page as a single embedding. To address this issue, we design a click feedback-aware web page summarization for multi-embedding-based retrieval (CPS-MEBR) framework which is able to generate multiple embeddings for web pages to match different potential queries. Specifically, we use the click data of users in search logs to train a summary model to extract those sentences in web pages that are frequently clicked by users, which are more likely to answer those potential queries. Meanwhile, we introduce sentence-level semantic interaction to design a multi-embedding-based retrieval (MEBR) model, which can generate multiple embeddings to deal with different potential queries by using frequently clicked sentences in web pages. Offline experiments show that it can perform high quality candidate retrieval compared to single-embedding-based retrieval (SEBR) model.
更多查看译文
关键词
retrieval,cps-mebr,feedback-aware,multi-embedding-based
AI 理解论文
溯源树
样例
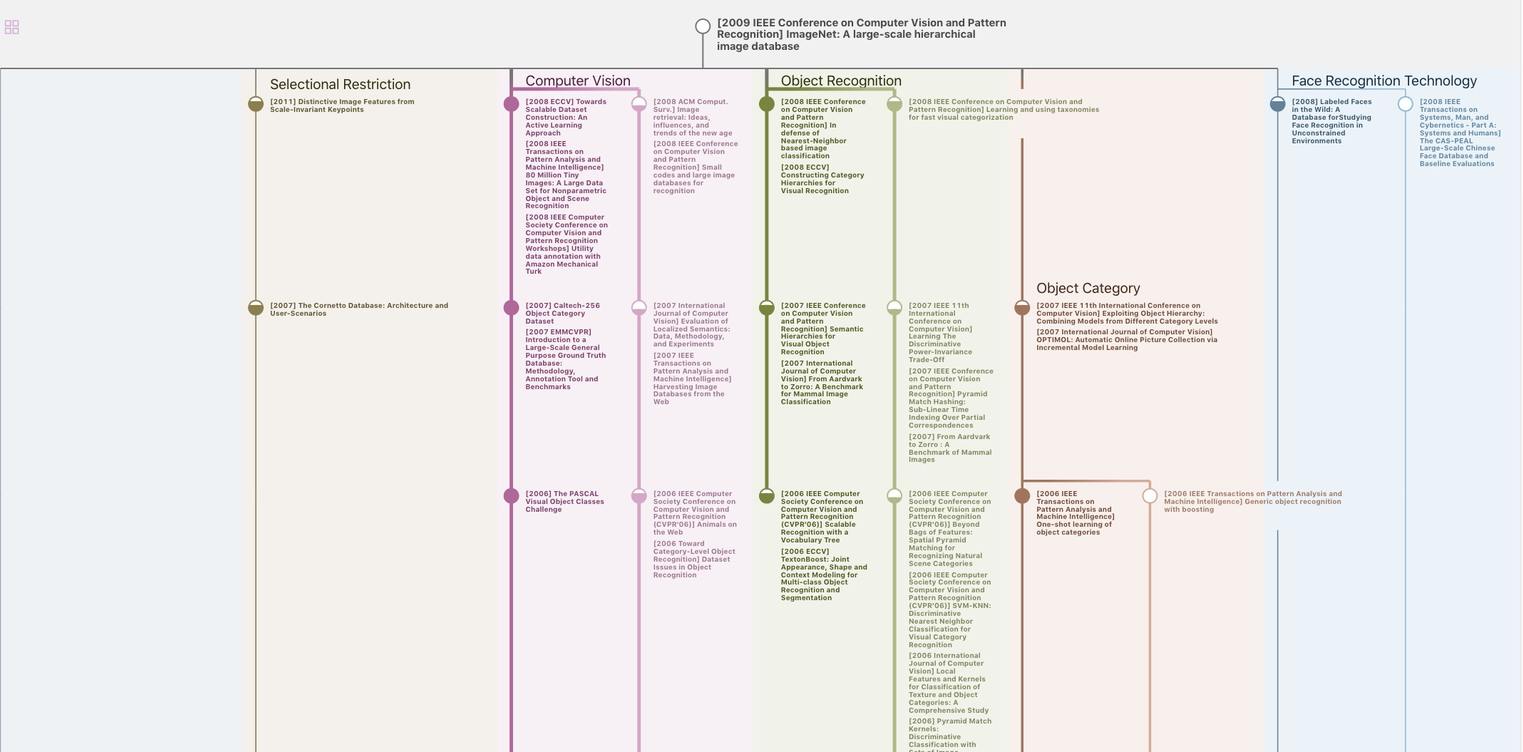
生成溯源树,研究论文发展脉络
Chat Paper
正在生成论文摘要