Generative Meta-Adversarial Network for Unseen Object Navigation.
European Conference on Computer Vision(2022)
摘要
Object navigation is a task to let the agent navigate to a target object. Prevailing works attempt to expand navigation ability in new environments and achieve reasonable performance on the seen object categories that have been observed in training environments. However, this setting is somewhat limited in real world scenario, where navigating to unseen object categories is generally unavoidable. In this paper, we focus on the problem of navigating to unseen objects in new environments only based on limited training knowledge. Same as the common ObjectNav tasks, our agent still gets the egocentric observation and target object category as the input and does not require any extra inputs. Our solution is to let the agent "imagine" the unseen object by synthesizing features of the target object. We propose a generative meta-adversarial network (GMAN), which is mainly composed of a feature generator and an environmental meta discriminator, aiming to generate features for unseen objects and new environments in two steps. The former generates the initial features of the unseen objects based on the semantic embedding of the object category. The latter enables the generator to further learn the background characteristics of the new environment, progressively adapting the generated features to approximate the real features of the target object. The adapted features serve as a more specific representation of the target to guide the agent. Moreover, to fast update the generator with a few observations, the entire adversarial framework is learned in the gradient-based meta-learning manner. The experimental results on AI2THOR and RoboTHOR simulators demonstrate the effectiveness of the proposed method in navigating to unseen object categories. The code is available at https://github.com/sx-zhang/GMAN.git.
更多查看译文
关键词
Object navigation, Unseen object, Adversarial learning
AI 理解论文
溯源树
样例
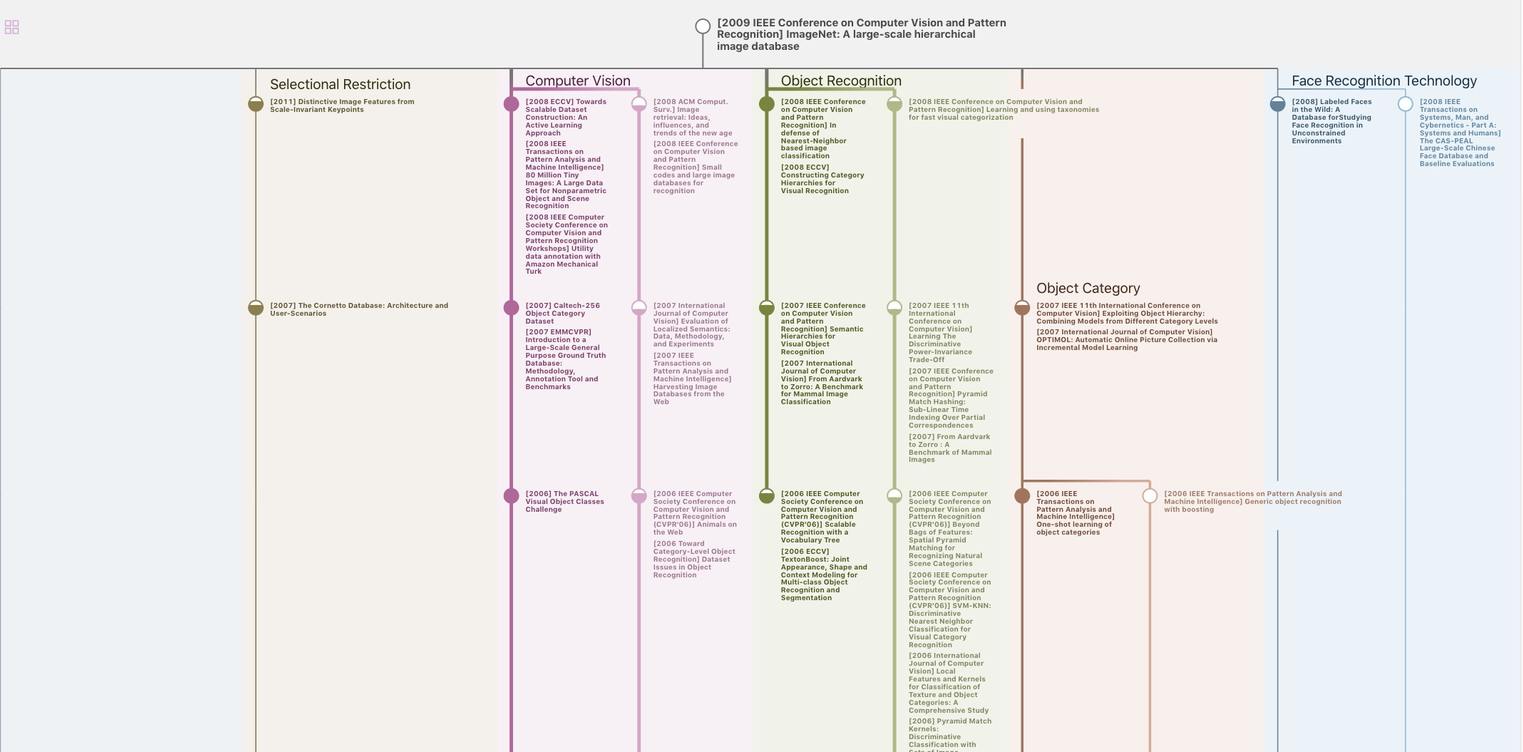
生成溯源树,研究论文发展脉络
Chat Paper
正在生成论文摘要