Uncertainty-Based Spatial-Temporal Attention for Online Action Detection.
European Conference on Computer Vision(2022)
摘要
Online action detection aims at detecting the ongoing action in a streaming video. In this paper, we proposed an uncertainty-based spatial-temporal attention for online action detection. By explicitly modeling the distribution of model parameters, we extend the baseline models in a probabilistic manner. Then we quantify the predictive uncertainty and use it to generate spatial-temporal attention that focus on large mutual information regions and frames. For inference, we introduce a two-stream framework that combines the baseline model and the probabilistic model based on the input uncertainty. We validate the effectiveness of our method on three benchmark datasets: THUMOS-14, TVSeries, and HDD. Furthermore, we demonstrate that our method generalizes better under different views and occlusions, and is more robust when training with small-scale data.
更多查看译文
关键词
Online action detection,Spatial-temporal attention,Uncertainty modeling,Generalization,Robustness
AI 理解论文
溯源树
样例
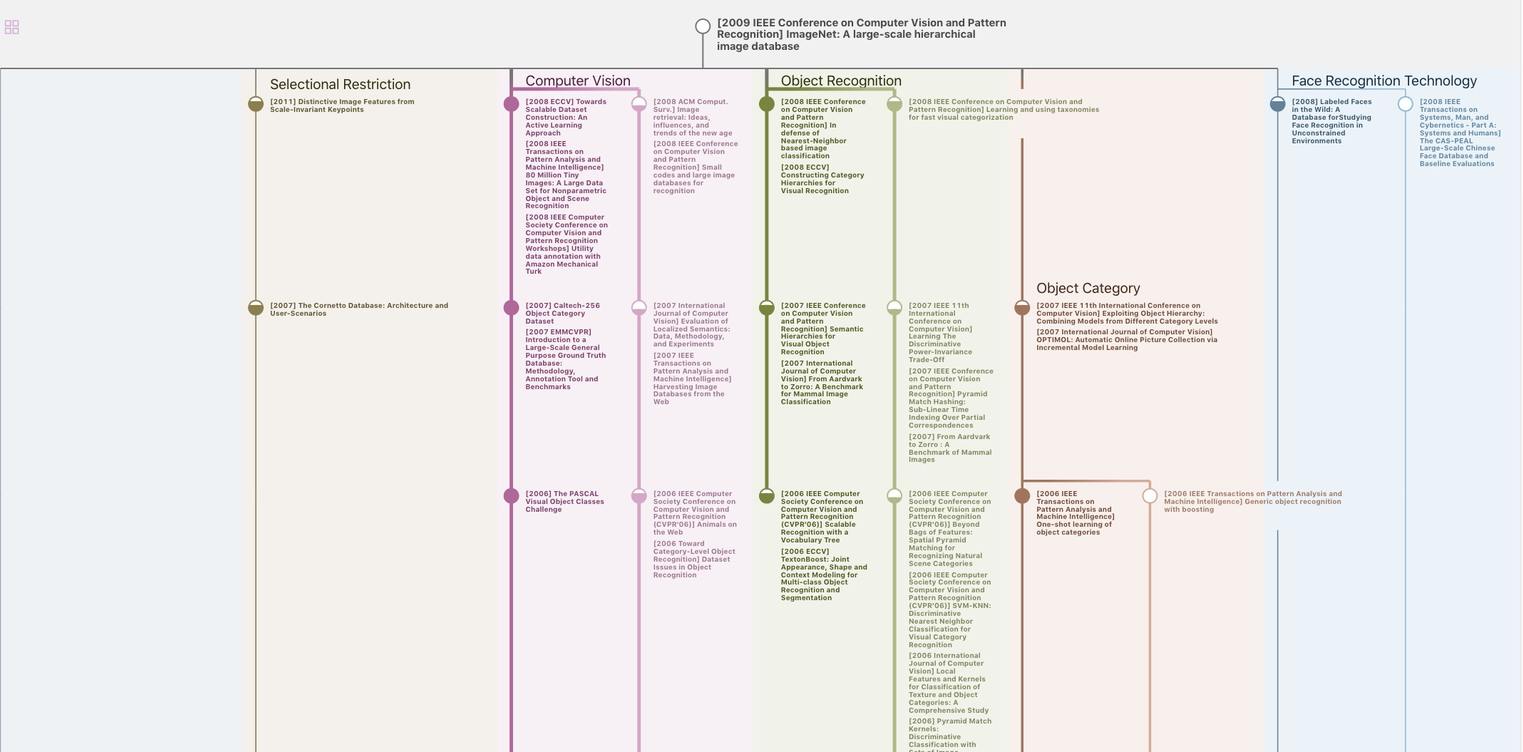
生成溯源树,研究论文发展脉络
Chat Paper
正在生成论文摘要