Augmenting Deep Classifiers with Polynomial Neural Networks.
European Conference on Computer Vision(2022)
摘要
Deep neural networks have been the driving force behind the success in classification tasks, e.g., object and audio recognition. Impressive results and generalization have been achieved by a variety of recently proposed architectures, the majority of which are seemingly disconnected. In this work, we cast the study of deep classifiers under a unifying framework. In particular, we express state-of-the-art architectures (e.g., residual and non-local networks) in the form of different degree polynomials of the input. Our framework provides insights on the inductive biases of each model and enables natural extensions building upon their polynomial nature. The efficacy of the proposed models is evaluated on standard image and audio classification benchmarks. The expressivity of the proposed models is highlighted both in terms of increased model performance as well as model compression. Lastly, the extensions allowed by this taxonomy showcase benefits in the presence of limited data and long-tailed data distributions. We expect this taxonomy to provide links between existing domain-specific architectures. The source code is available at https://github.com/grigorisg9gr/polynomials-for-augmenting-NNs.
更多查看译文
关键词
Polynomial neural networks, Tensor decompositions, Polynomial expansions, Classification
AI 理解论文
溯源树
样例
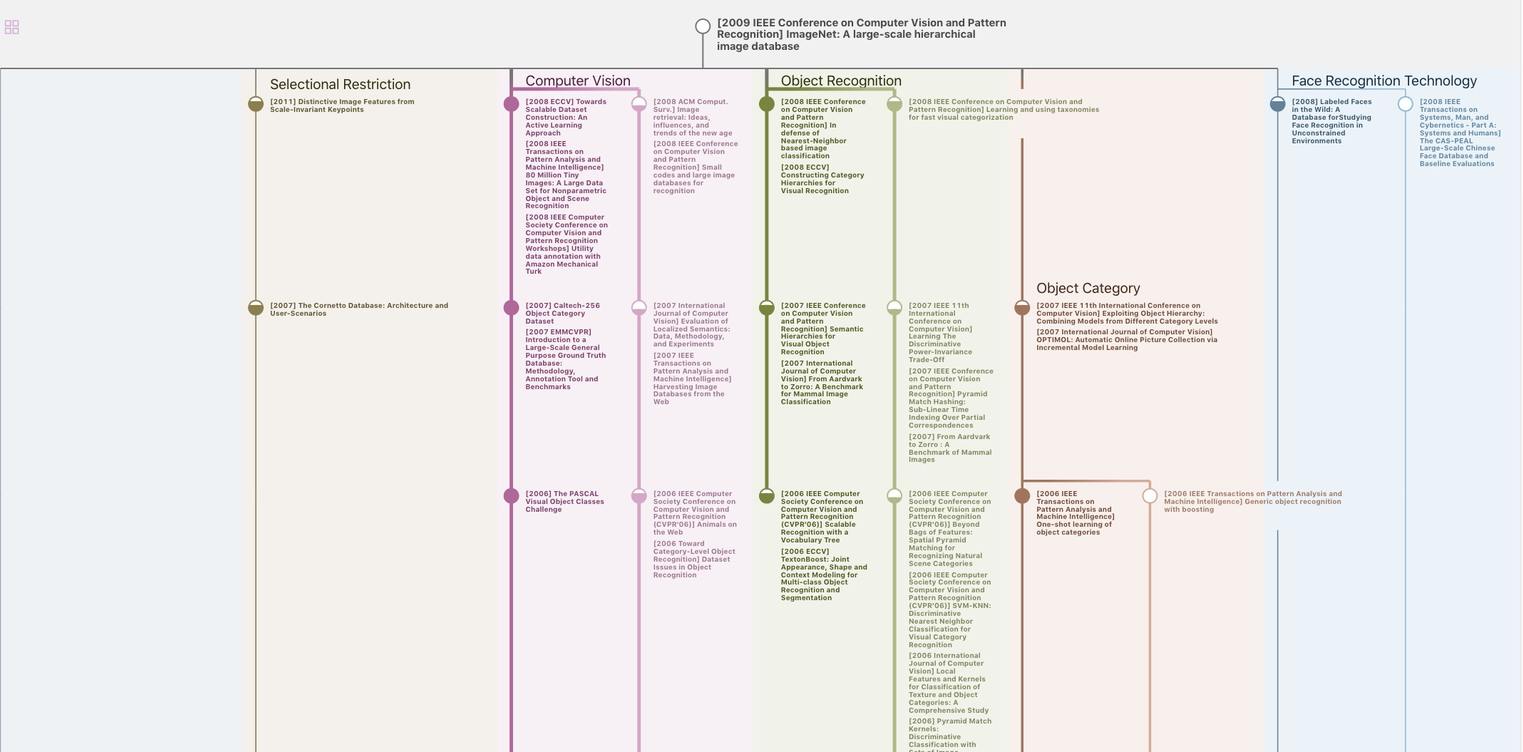
生成溯源树,研究论文发展脉络
Chat Paper
正在生成论文摘要