Wavelet-based fuzzy clustering of interval time series
International Journal of Approximate Reasoning(2022)
摘要
We investigate the fuzzy clustering of interval time series using wavelet variances and covariances; in particular, we use a fuzzy c-medoids clustering algorithm. Traditional hierarchical and non-hierarchical clustering methods lead to the identification of mutually exclusive clusters whereas fuzzy clustering methods enable the identification of overlapping clusters, implying that one or more series could belong to more than one cluster simultaneously. An interval time series (ITS) which arises when interval-valued observations are recorded over time is able to capture the variability of values within each interval at each time point. This is in contrast to single-point information available in a classical time series. Our main contribution is that by combining wavelet analysis, interval data analysis and fuzzy clustering, we are able to capture information which would otherwise have not been contemplated by the use of traditional crisp clustering methods on classical time series for which just a single value is recorded at each time point. Through simulation studies, we show that under some circumstances fuzzy c-medoids clustering performs better when applied to ITS than when it is applied to the corresponding traditional time series. Applications to exchange rates ITS and sea-level ITS show that the fuzzy clustering method reveals different and more meaningful results than when applied to associated single-point time series.
更多查看译文
关键词
Interval time series,Wavelet variance,Wavelet covariances,Fuzzy clustering,Partitioning around medoids
AI 理解论文
溯源树
样例
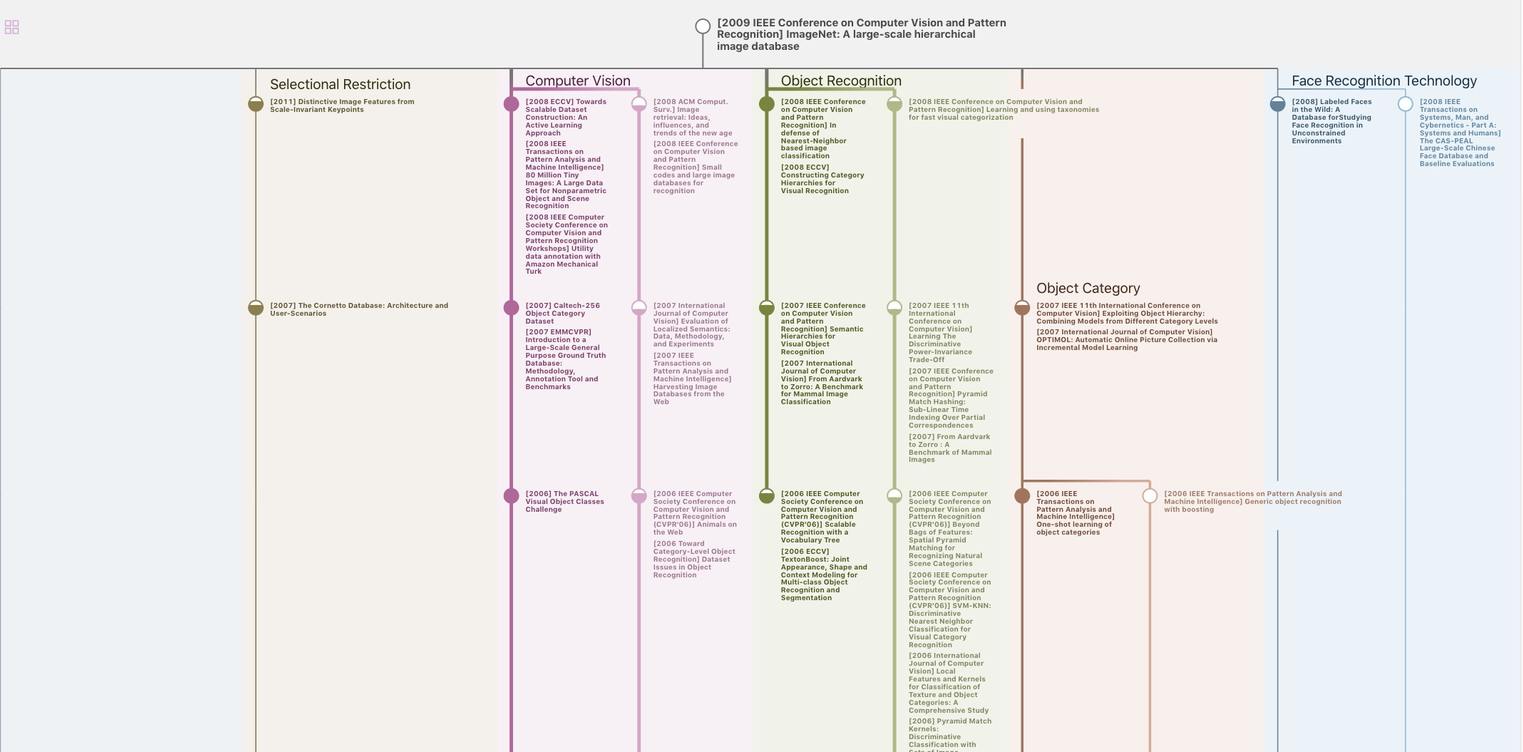
生成溯源树,研究论文发展脉络
Chat Paper
正在生成论文摘要