Feature redundancy assessment framework for subject matter experts
Engineering Applications of Artificial Intelligence(2023)
摘要
Traditional feature removal techniques focus on showing how well the selected subset of features can perform in terms of model accuracy while neglecting the aspect of eliminating redundant features and incorporating Subject Matter Experts’ (SME) prior knowledge. This is important so that SMEs can leverage their prior knowledge to incorporate actionable or controllable features to build a downstream model with confidence and practical application. Furthermore, feature removal should include evidence on how similar the redundant features are with the selected features. We proposed a framework that incorporates SME prior knowledge to assess/augment the relevancy of the features with respect to the domain-specific problem. First, we rely on the Variance Inflation Factor (VIF) to iteratively remove the redundant features and measure their information loss. The quantifying of information loss will assist the SME in determining the number of features to be selected. Next, Partitions Around Medoids (PAM) is used to cluster redundant features to the closest selected feature. These clusters guide the SME in the augmentation process where the SME can retain, add, or swap the preferred features with those deemed non-redundant by the algorithm. We compared our result based on four commonly used benchmark datasets (Alate Adelges, Sonar, Wisconsin Diagnostic Breast Cancer, and Wine) with the features selected by domain experts, how they are being grouped, and the possible options to perform feature swaps. Our results show the similarity features between redundant features and their corresponding selected features. Also, we have demonstrated that our framework is able to maintain comparable retained information with those supervised feature selection methods, and demonstrate overall higher retained information of up to 3%.
更多查看译文
关键词
Feature redundancy,Feature selection,Clustering,Guided feature,Feature swap assessment,Retained information,Unsupervised task,Human-in-the-loop,Subject matter expert in the loop
AI 理解论文
溯源树
样例
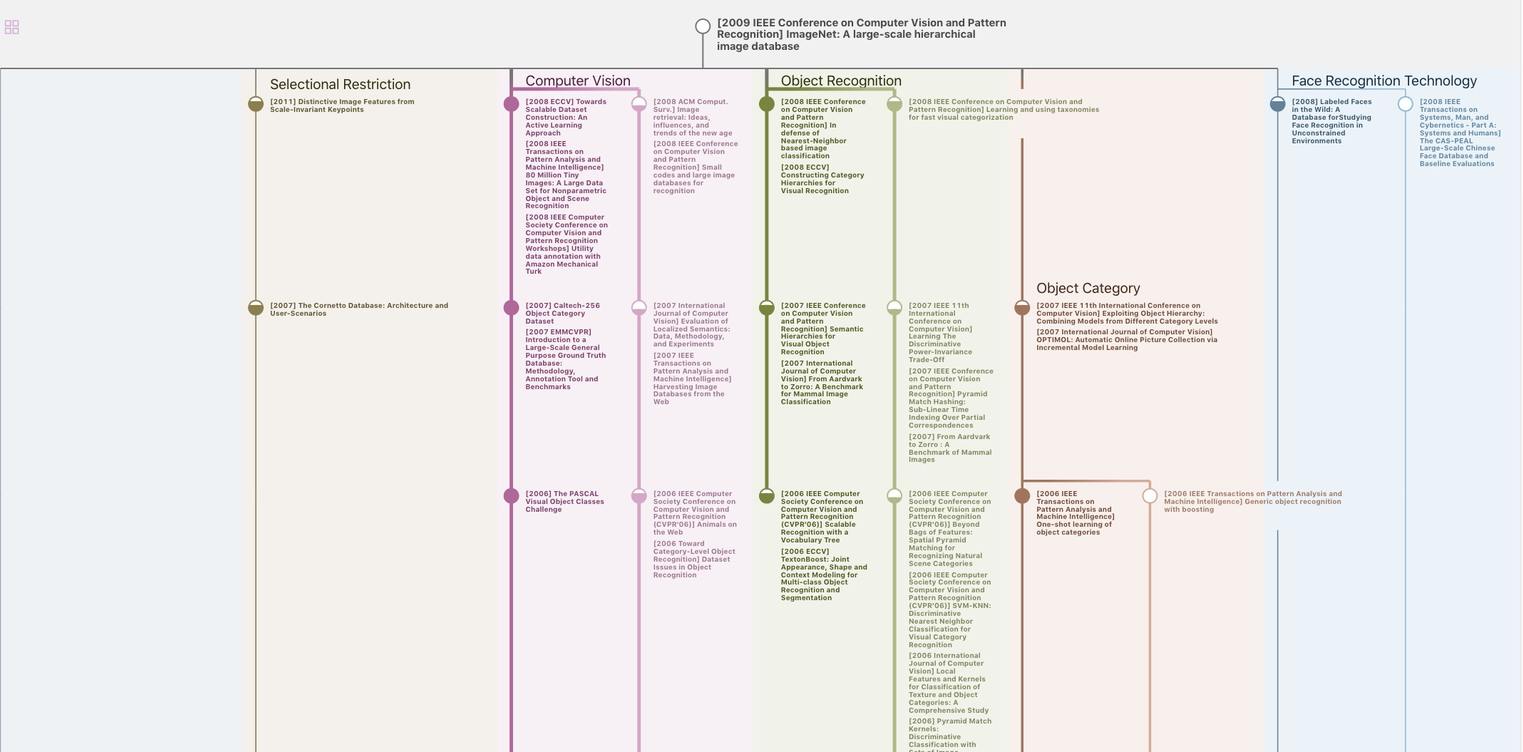
生成溯源树,研究论文发展脉络
Chat Paper
正在生成论文摘要