Bearing Fault Diagnosis for Time-Varying System Using Vibration–Speed Fusion Network Based on Self-Attention and Sparse Feature Extraction
Machines(2022)
摘要
Nowadays, most deep-learning-based bearing fault diagnosis methods are studied under the condition of steady speed, while the performance of these models cannot be fully played under time-varying conditions. Therefore, in order to facilitate the practical application of a deep learning model in bearing fault diagnosis, a vibration–speed fusion network is proposed, which utilizes a transformer with a self-attention module to extract vibration features and utilizes a sparse autoencoder (SAE) network to extract sparse features from speed pulse signal. The vibration–speed fusion network enables the efficient fusion of different signals in a high-dimensional vector space with a high degree of model interpretability, without additional signal processing steps. After tuning the hyperparameters of the network, the key segments of the bearing’s time-domain vibration signals can be optimally extracted, the network performance is much better than traditional deep learning methods, and the classification accuracy can reach 95.18% and 99.85% on the two public bearing datasets from the Xi’an Jiaotong University and the University of Ottawa.
更多查看译文
关键词
intelligent fault diagnosis,transformer,time-varying rotational speed,feature visualization
AI 理解论文
溯源树
样例
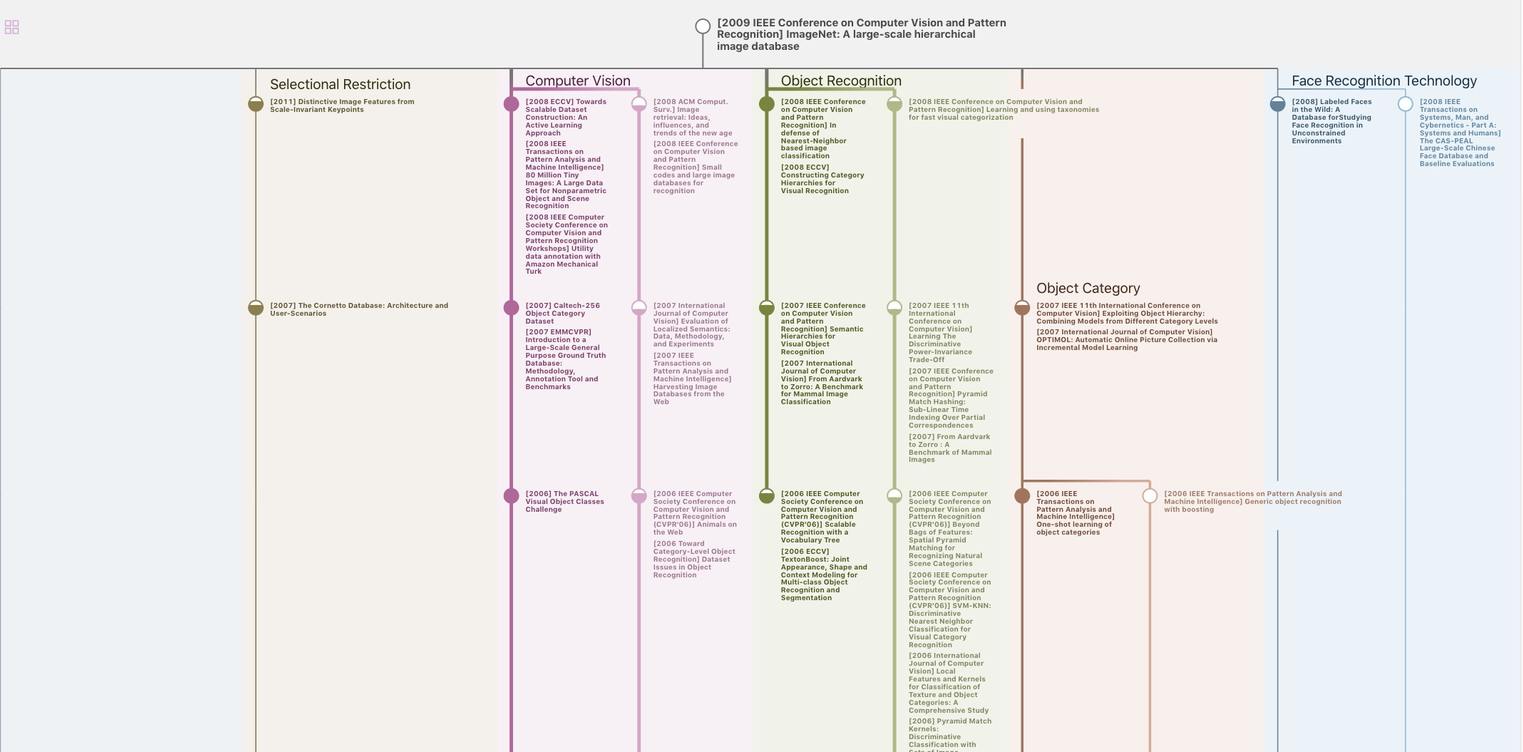
生成溯源树,研究论文发展脉络
Chat Paper
正在生成论文摘要