Multiscale feature pyramid network based on activity level weight selection for infrared and visible image fusion
Journal of the Optical Society of America A(2022)
摘要
At present, deep-learning-based infrared and visible image fusion methods have the problem of extracting insufficient source image features, causing imbalanced infrared and visible information in fused images. To solve the problem, a multiscale feature pyramid network based on activity level weight selection (MFPN-AWS) with a complete downsampling-upsampling structure is proposed. The network consists of three parts: a downsampling convolutional network, an AWS fusion layer, and an upsampling convolutional network. First, multiscale deep features are extracted by downsampling convolutional networks, obtaining rich information of intermediate layers. Second, AWS highlights the advantages of the l1-norm and global pooling dual fusion strategy to describe the characteristics of target saliency and texture detail, and effectively balances the multiscale infrared and visible features. Finally, multiscale fused features are reconstructed by the upsampling convolutional network to obtain fused images. Compared with nine state-of-the-art methods via the publicly available experimental datasets TNO and VIFB, MFPN-AWS reaches more natural and balanced fusion results, such as better overall clarity and salient targets, and achieves optimal values on two metrics: mutual information and visual fidelity. (c) 2022 Optica Publishing Group
更多查看译文
关键词
multiscale feature pyramid network,activity level weight selection,fusion
AI 理解论文
溯源树
样例
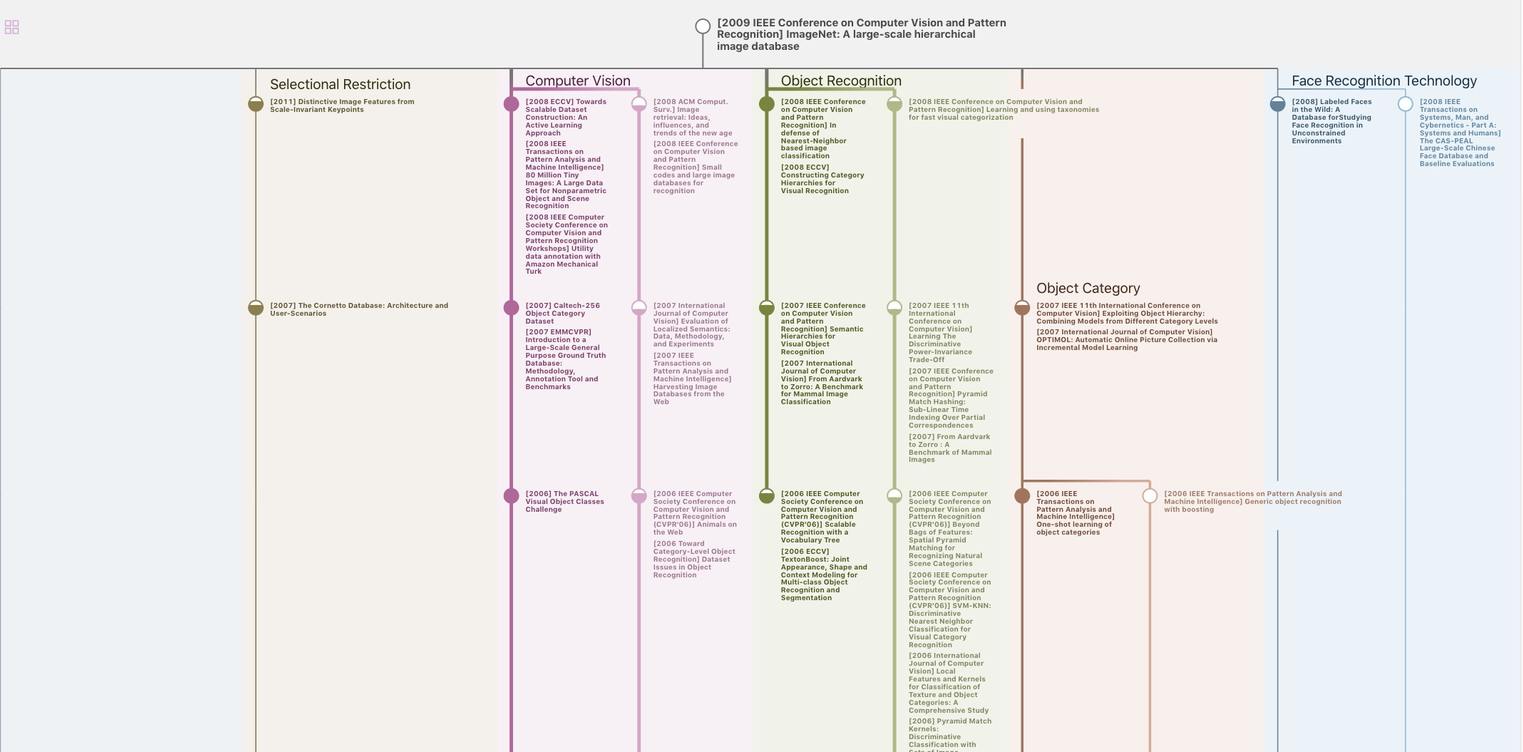
生成溯源树,研究论文发展脉络
Chat Paper
正在生成论文摘要