Forging Multiple Training Objectives for Pre-trained Language Models via Meta-Learning
arxiv(2022)
摘要
Multiple pre-training objectives fill the vacancy of the understanding capability of single-objective language modeling, which serves the ultimate purpose of pre-trained language models (PrLMs), generalizing well on a mass of scenarios. However, learning multiple training objectives in a single model is challenging due to the unknown relative significance as well as the potential contrariety between them. Empirical studies have shown that the current objective sampling in an ad-hoc manual setting makes the learned language representation barely converge to the desired optimum. Thus, we propose \textit{MOMETAS}, a novel adaptive sampler based on meta-learning, which learns the latent sampling pattern on arbitrary pre-training objectives. Such a design is lightweight with negligible additional training overhead. To validate our approach, we adopt five objectives and conduct continual pre-training with BERT-base and BERT-large models, where MOMETAS demonstrates universal performance gain over other rule-based sampling strategies on 14 natural language processing tasks.
更多查看译文
关键词
multiple training objectives,language models,pre-trained,meta-learning
AI 理解论文
溯源树
样例
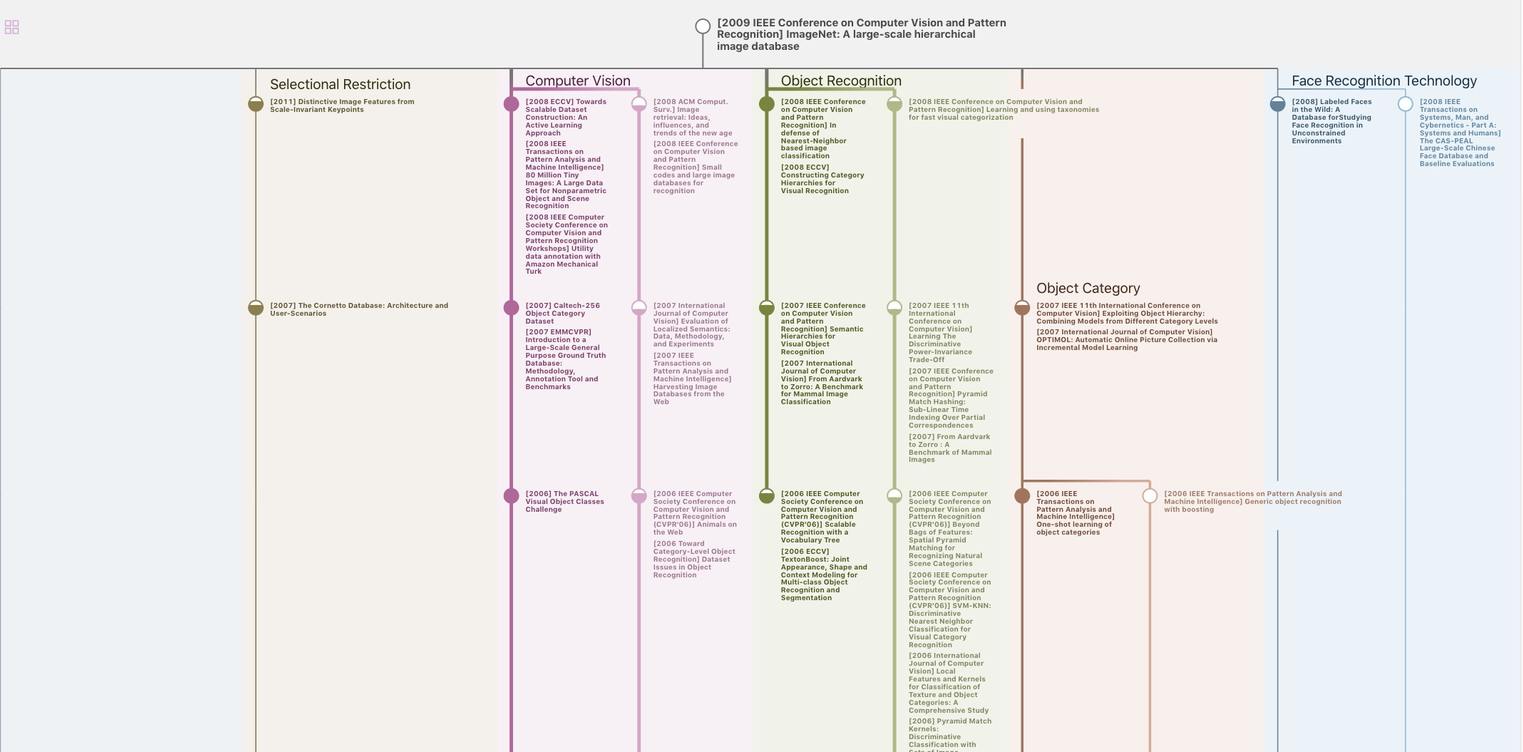
生成溯源树,研究论文发展脉络
Chat Paper
正在生成论文摘要