Digital Human Interactive Recommendation Decision-Making Based on Reinforcement Learning
arxiv(2022)
摘要
Digital human recommendation system has been developed to help customers find their favorite products and is playing an active role in various recommendation contexts. How to timely catch and learn the dynamics of the preferences of the customers, while meeting their exact requirements, becomes crucial in the digital human recommendation domain. We design a novel practical digital human interactive recommendation agent framework based on Reinforcement Learning(RL) to improve the efficiency of the interactive recommendation decision-making by leveraging both the digital human features and the superior flexibility of RL. Our proposed framework learns through real-time interactions between the digital human and customers dynamically through the state-of-art RL algorithms, combined with multimodal embedding and graph embedding, to improve the accuracy of personalization and thus enable the digital human agent to timely catch the attention of the customer. Experiments on real business data demonstrate that our framework can provide better personalized customer engagement and better customer experiences.
更多查看译文
AI 理解论文
溯源树
样例
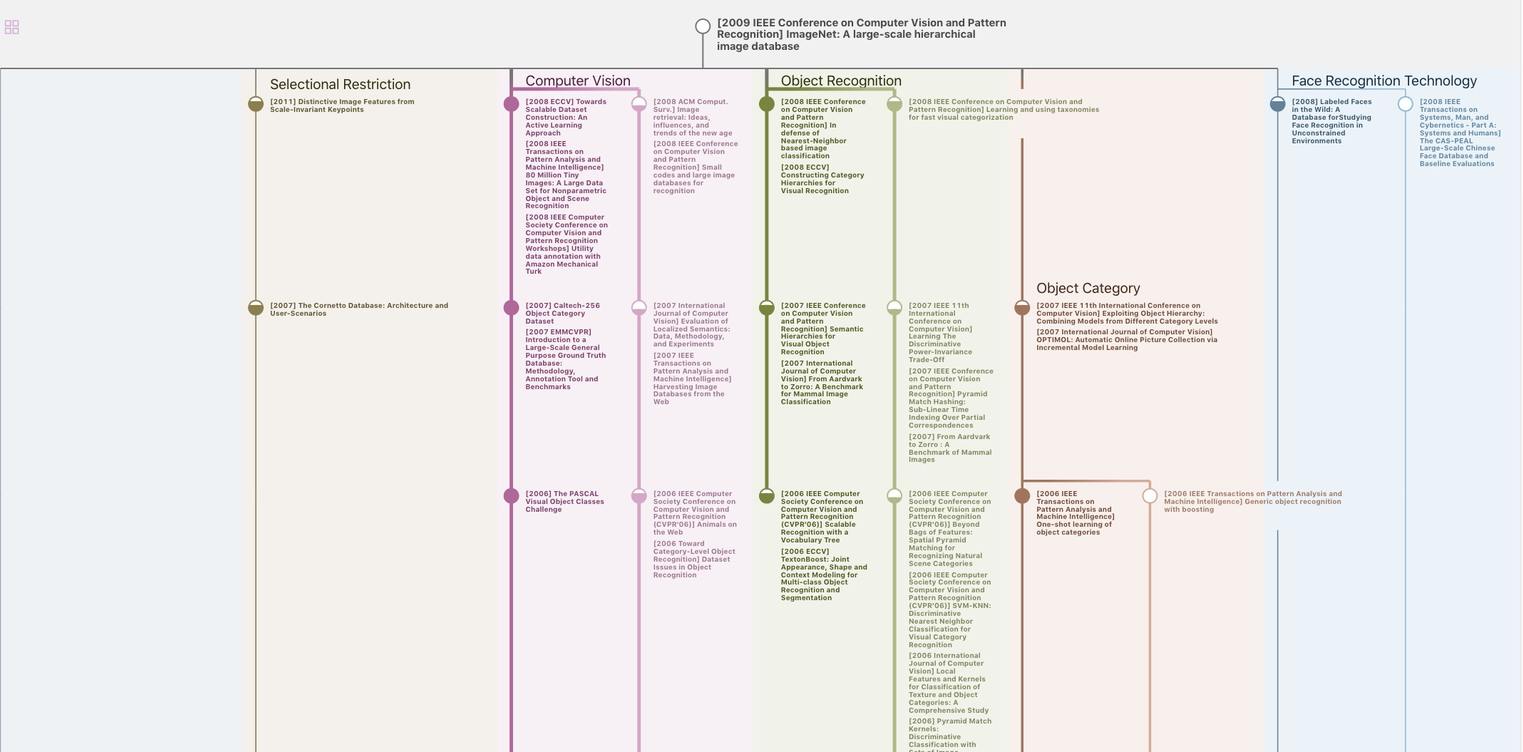
生成溯源树,研究论文发展脉络
Chat Paper
正在生成论文摘要