Fant\^omas: Evaluating Reversibility of Face Anonymizations Using a General Deep Learning Attacker
arxiv(2022)
摘要
Biometric data is a rich source of information that can be used to identify individuals and infer private information about them. To mitigate this privacy risk, anonymization techniques employ transformations on clear data to obfuscate sensitive information, all while retaining some utility of the data. Albeit published with impressive claims, they sometimes are not evaluated with convincing methodology. We hence are interested to which extent recently suggested anonymization techniques for obfuscating facial images are effective. More specifically, we test how easily they can be automatically reverted, to estimate the privacy they can provide. Our approach is agnostic to the anonymization technique as we learn a machine learning model on the clear and corresponding anonymized data. We find that 10 out of 14 tested face anonymization techniques are at least partially reversible, and six of them are at least highly reversible.
更多查看译文
AI 理解论文
溯源树
样例
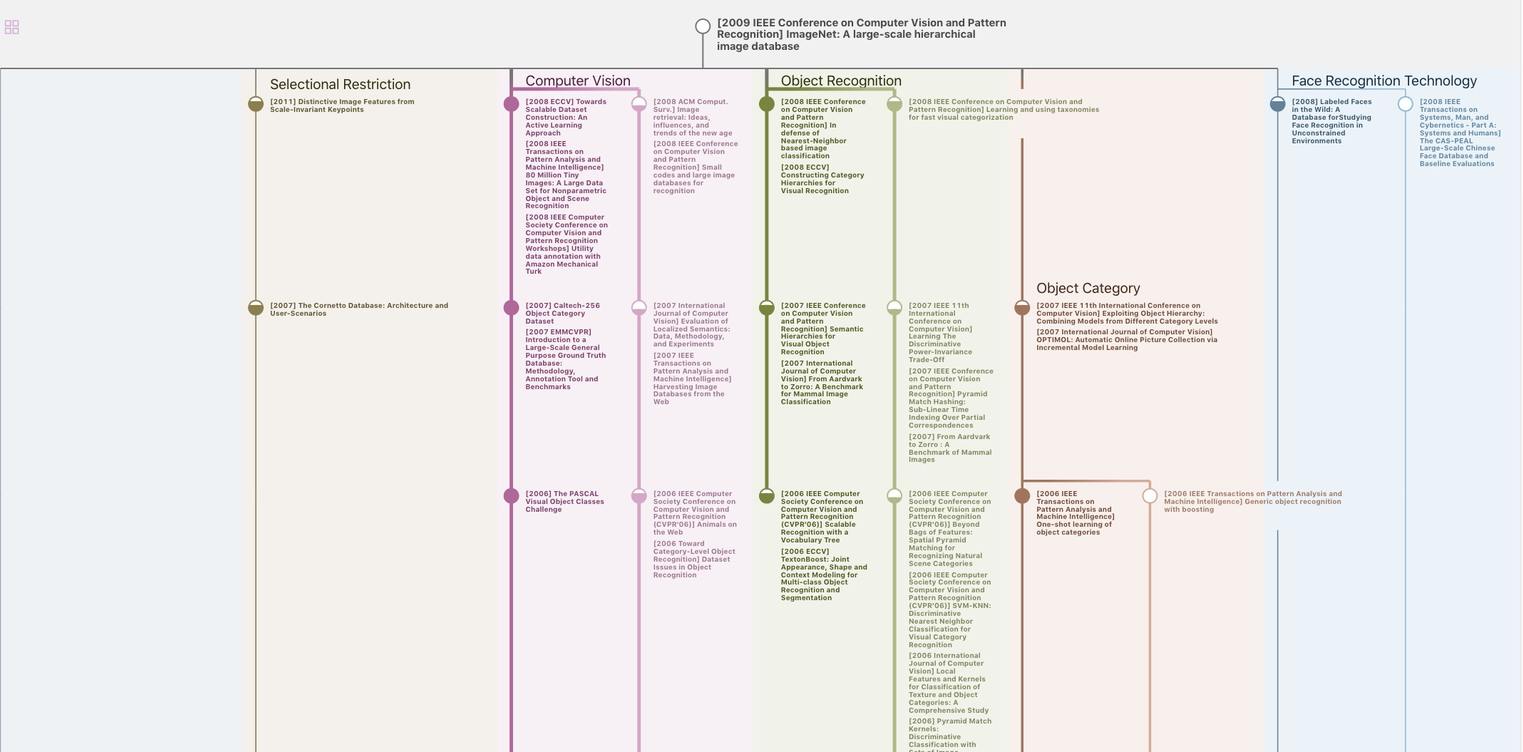
生成溯源树,研究论文发展脉络
Chat Paper
正在生成论文摘要