Predicting Melt Pool Dimensions for Wire-Feed Directed Energy Deposition Process
Integrating Materials and Manufacturing Innovation(2022)
摘要
Additive manufacturing (AM) is gaining attention because of its ability to design complex geometries. Direct energy deposition (DED), one of the AM processes, is widely used nowadays for its high deposition rate. When using DED process in manufacturing or repairing, it is important to know the melt pool dimensions as a function of processing parameters to obtain high deposition rate and avoid defects such as lack of fusion. In this study, we used the random forest (RF) algorithm to predict melt pool dimensions and compared the results against existing physics based lumped model by Doumanidis et al. [ 1 ]. The results show that RF model works well to predict the DED melt pool dimensions, where energy density and material volume deposited govern the dimensions. Further, we tested the ubiquitous semi-ellipsoidal shape assumption for DED cross section against the circular shape and found semi-ellipsoidal shape to be fair when deposition process is stable and free of defects. Overall, this study highlights the applicability of machine learning algorithms for small AM datasets.
更多查看译文
关键词
Regression analysis,Directed energy deposition,Ti-6Al-4V,Melt pool shape
AI 理解论文
溯源树
样例
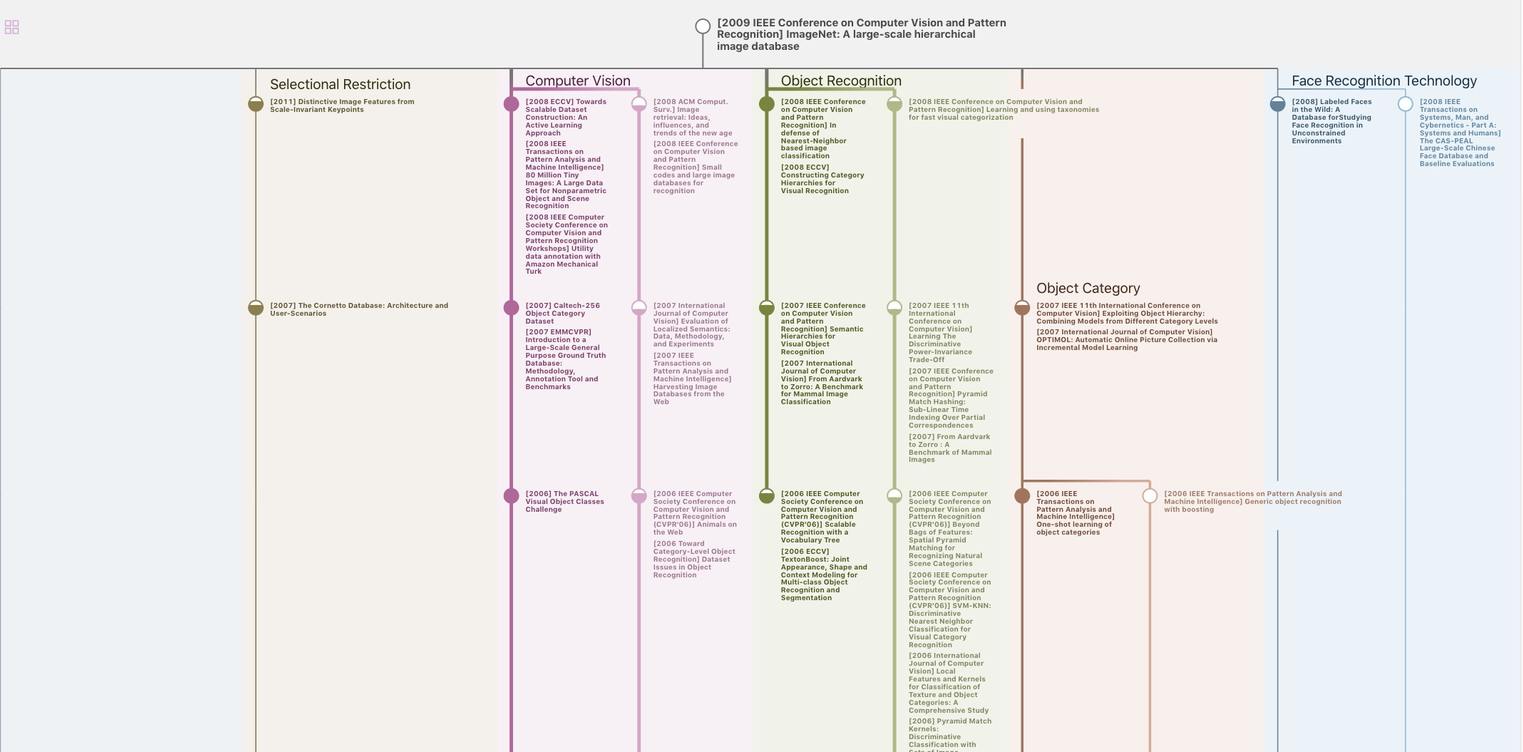
生成溯源树,研究论文发展脉络
Chat Paper
正在生成论文摘要