Inverse design of high degree of freedom meta-atoms based on machine learning and genetic algorithm methods.
Optics express(2022)
摘要
Since inverse design is an ill-conditioned problem of mapping from low dimensions to high dimensions, inverse design is challenging, especially for design problems with many degrees of freedom (DOFs). Traditional deep learning methods and optimization methods cannot readily calculate the inverse design of meta-atoms with high DOFs. In this paper, a new method combining deep learning and genetic algorithm (GA) methods is proposed to realize the inverse design of meta-atoms with high DOFs. In this method, a predicting neural network (PNN) and a variational autoencoder (VAE) generation model are constructed and trained. The generative model is used to constrain and compress the large design space, so that the GA can jump out of the local optimal solution and find the global optimal solution. The predicting model is used to quickly evaluate the fitness value of each offspring in the GA. With the assistance of these two machine learning models, the GA can find the optimal design of meta-atoms. This approach can realize, on demand, inverse design of meta-atoms, and opens the way for the optimization of procedures in other fields.
更多查看译文
关键词
inverse design,meta-atoms
AI 理解论文
溯源树
样例
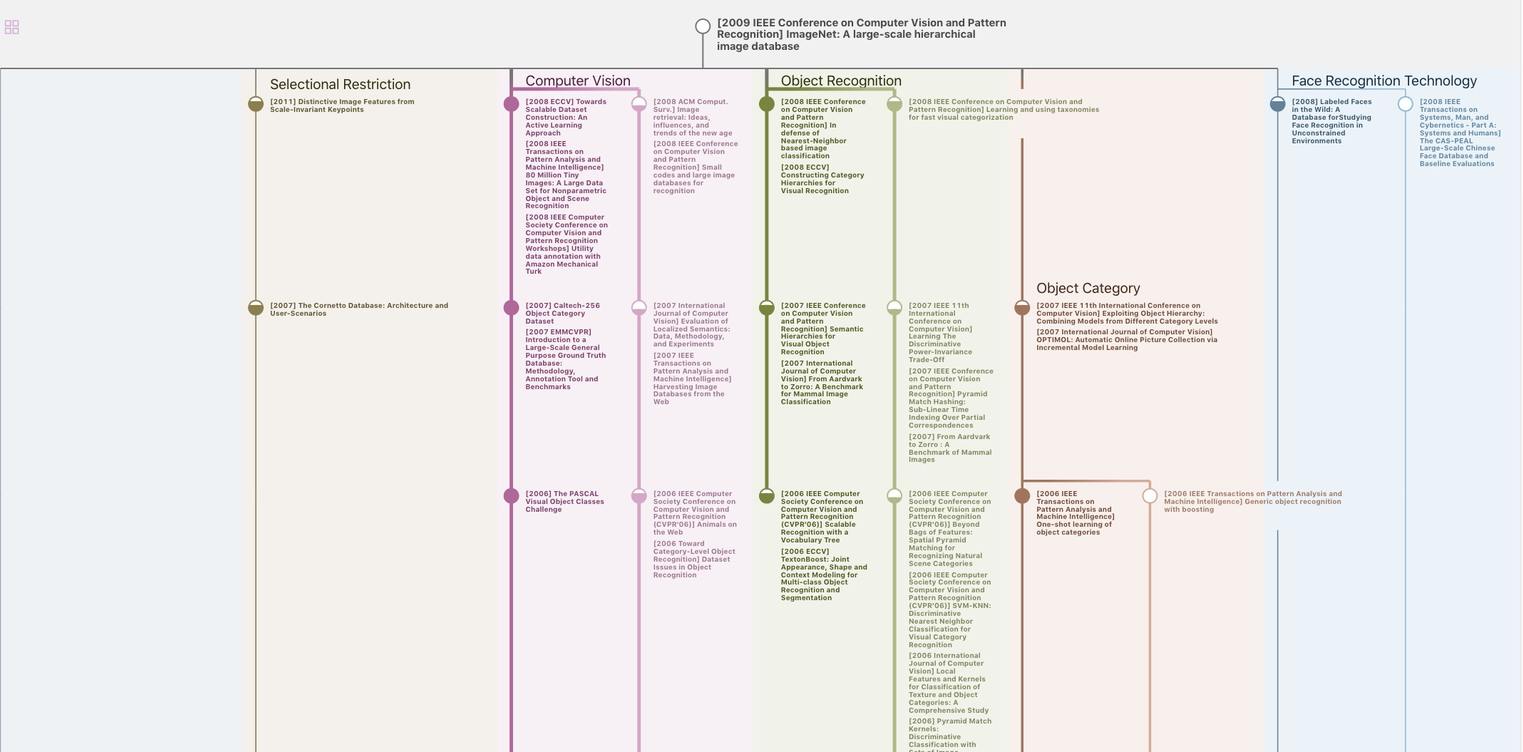
生成溯源树,研究论文发展脉络
Chat Paper
正在生成论文摘要