Competitive Online Truthful Time-Sensitive-Valued Data Auction
arxiv(2022)
摘要
In this work, we investigate online mechanisms for trading time-sensitive valued data. We adopt a continuous function $d(t)$ to represent the data value fluctuation over time $t$. Our objective is to design an \emph{online} mechanism achieving \emph{truthfulness} and \emph{revenue-competitiveness}. We first prove several lower bounds on the revenue competitive ratios under various assumptions. We then propose several online truthful auction mechanisms for various adversarial models, such as a randomized observe-then-select mechanism $\mathcal{M}_1$ and prove that it is \textit{truthful} and $\Theta(\log n)$-competitive under some assumptions. Then we present an effective truthful weighted-selection mechanism $\mathcal{M'}_W$ by relaxing the assumptions on the sizes of the discount-classes. We prove that it achieves a competitive ratio $\Theta(n\log n)$ for any known non-decreasing discount function $d(t)$, and the number of buyers in each discount class $n_c \ge 2$. When the optimum expected revenue $OPT_1$ can be estimated within a constant factor, i.e. $c_0 \cdot OPT_1 \le Z \le OPT_1 $ for some constant $c_0 \in(0,1)$, we propose a truthful online posted-price mechanism that achieves a constant competitive ratio $\frac{4}{c_0}$. Our extensive numerical evaluations demonstrate that our mechanisms perform very well in most cases.
更多查看译文
关键词
competitive online,data,time-sensitive-valued
AI 理解论文
溯源树
样例
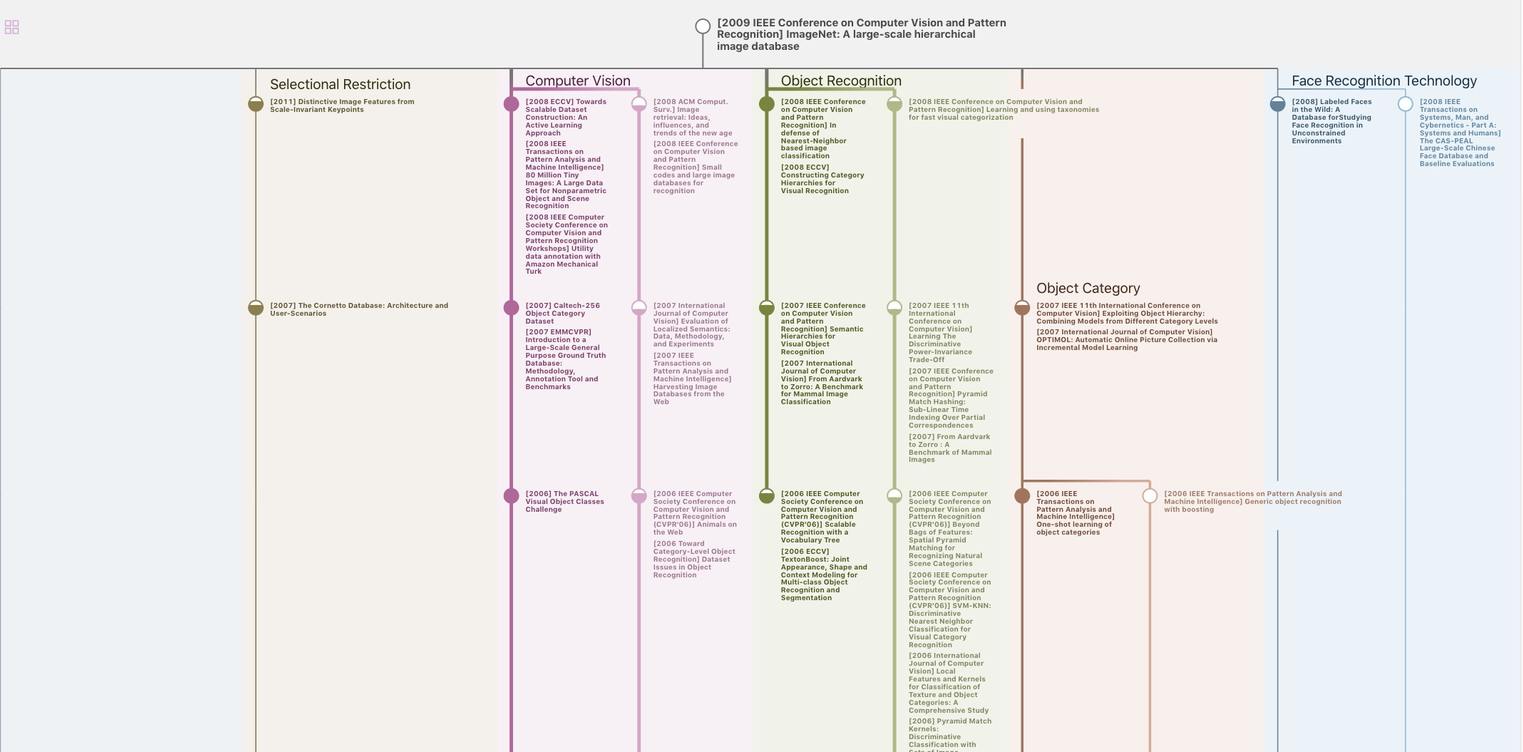
生成溯源树,研究论文发展脉络
Chat Paper
正在生成论文摘要