Inference from Real-World Sparse Measurements
Trans Mach Learn Res(2024)
摘要
Real-world problems often involve complex and unstructured sets ofmeasurements, which occur when sensors are sparsely placed in either space ortime. Being able to model this irregular spatiotemporal data and extractmeaningful forecasts is crucial. Deep learning architectures capable ofprocessing sets of measurements with positions varying from set to set, andextracting readouts anywhere are methodologically difficult. Currentstate-of-the-art models are graph neural networks and require domain-specificknowledge for proper setup. We propose an attention-based model focused on robustness and practicalapplicability, with two key design contributions. First, we adopt a ViT-liketransformer that takes both context points and read-out positions as inputs,eliminating the need for an encoder-decoder structure. Second, we use a unifiedmethod for encoding both context and read-out positions. This approach isintentionally straightforward and integrates well with other systems. Comparedto existing approaches, our model is simpler, requires less specializedknowledge, and does not suffer from a problematic bottleneck effect, all ofwhich contribute to superior performance. We conduct in-depth ablation studies that characterize this problematicbottleneck in the latent representations of alternative models that inhibitinformation utilization and impede training efficiency. We also performexperiments across various problem domains, including high-altitude windnowcasting, two-day weather forecasting, fluid dynamics, and heat diffusion.Our attention-based model consistently outperforms state-of-the-art models inhandling irregularly sampled data. Notably, our model reduces the root meansquare error (RMSE) for wind nowcasting from 9.24 to 7.98 and for heatdiffusion tasks from 0.126 to 0.084.
更多查看译文
AI 理解论文
溯源树
样例
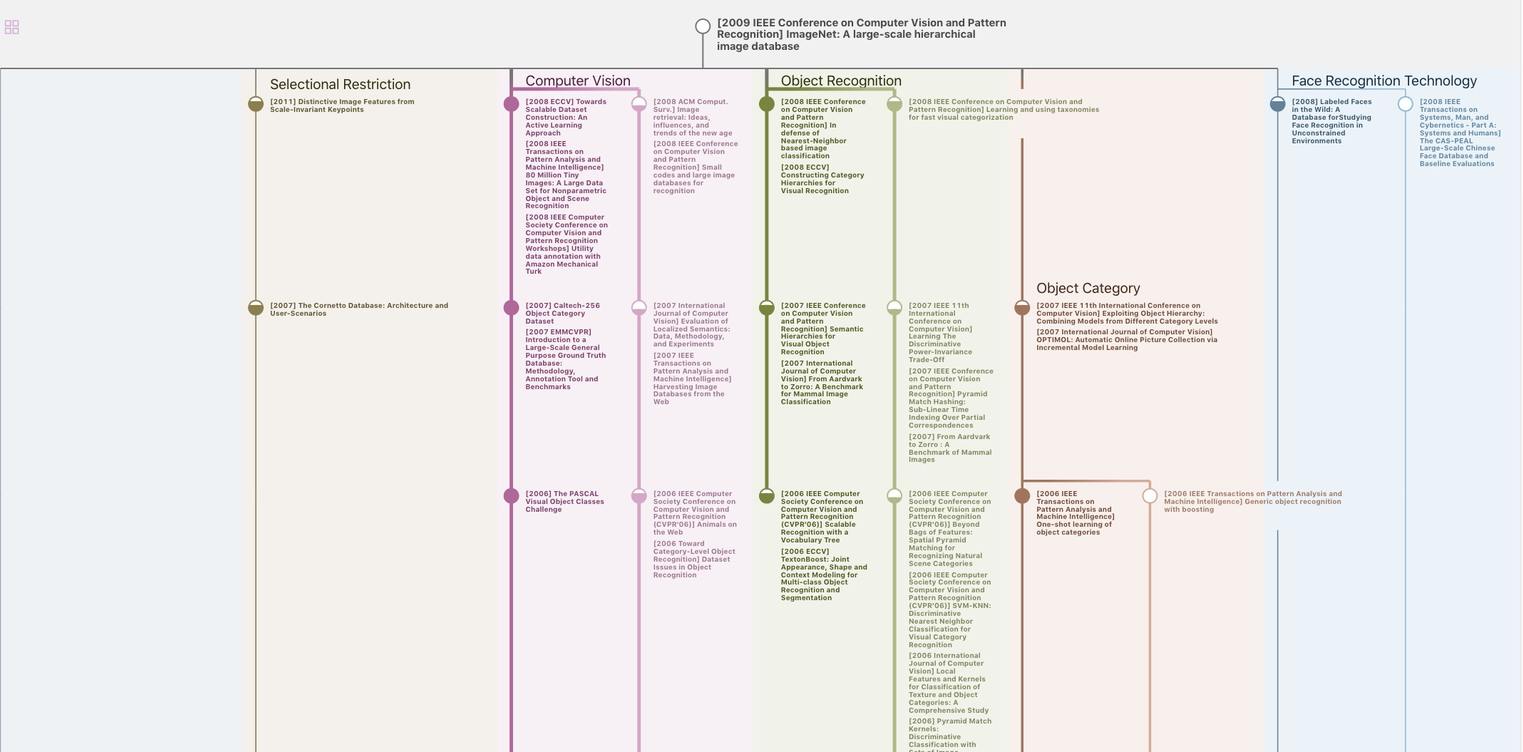
生成溯源树,研究论文发展脉络
Chat Paper
正在生成论文摘要