Deep reinforcement learning oriented for real world dynamic scenarios
CoRR(2022)
摘要
Autonomous navigation in dynamic environments is a complex but essential task for autonomous robots. Recent deep reinforcement learning approaches show promising results to solve the problem, but it is not solved yet, as they typically assume no robot kinodynamic restrictions, holonomic movement or perfect environment knowledge. Moreover, most algorithms fail in the real world due to the inability to generate real-world training data for the huge variability of possible scenarios. In this work, we present a novel planner, DQN-DOVS, that uses deep reinforcement learning on a descriptive robocentric velocity space model to navigate in highly dynamic environments. It is trained using a smart curriculum learning approach on a simulator that faithfully reproduces the real world, reducing the gap between the reality and simulation. We test the resulting algorithm in scenarios with different number of obstacles and compare it with many state-of-the-art approaches, obtaining a better performance. Finally, we try the algorithm in a ground robot, using the same setup as in the simulation experiments.
更多查看译文
关键词
deep reinforcement learning,dynamic scenarios,real world
AI 理解论文
溯源树
样例
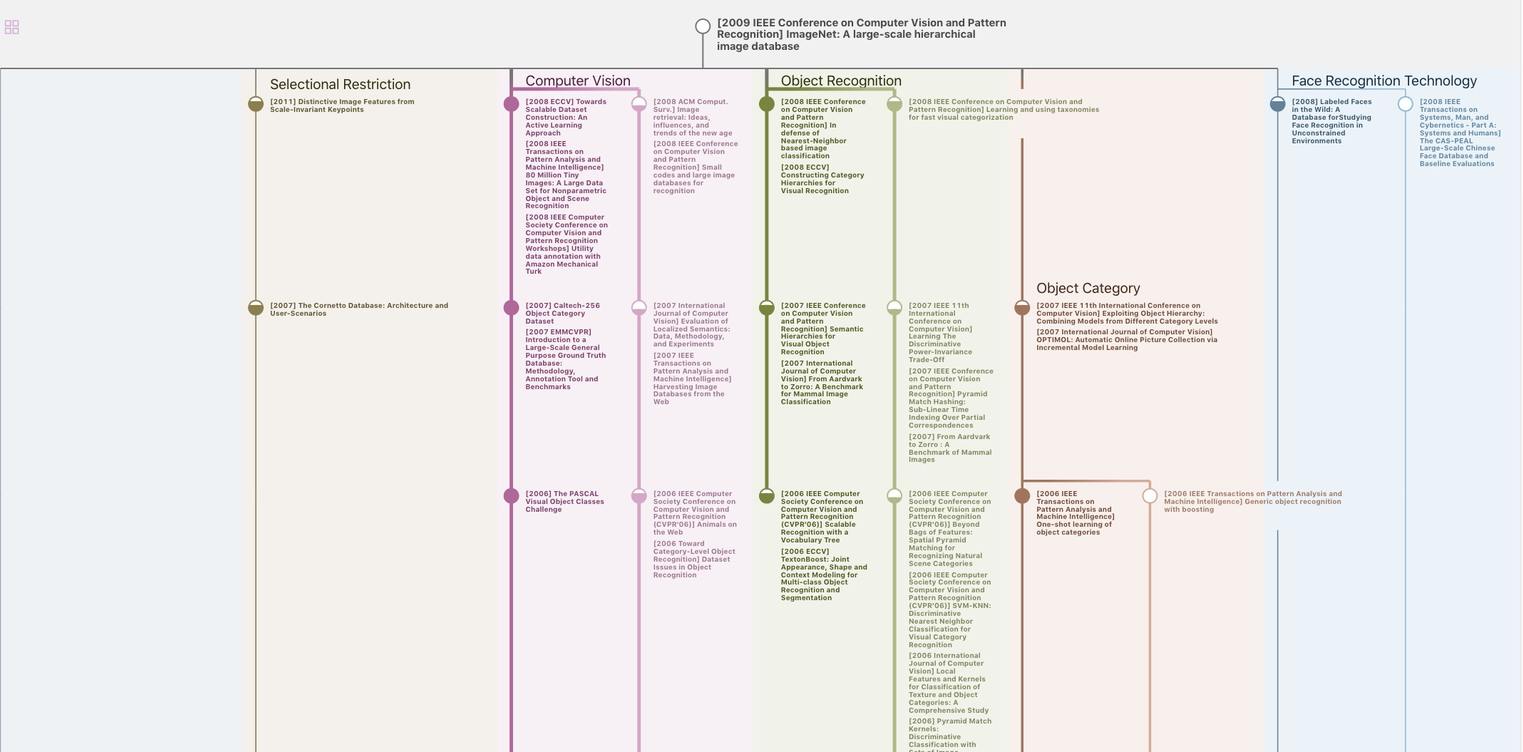
生成溯源树,研究论文发展脉络
Chat Paper
正在生成论文摘要