Modelling the Effects of Self-learning and Social Influence on the Diversity of Knowledge
COMPLEX NETWORKS & THEIR APPLICATIONS X, VOL 2(2022)
摘要
This paper presents a computational model of acquiring new knowledge through self-learning (e.g. a Wikipedia "rabbit hole") or social influence (e.g. recommendations through friends). This is set up in a bipartite network between a static social network (agents) and a static knowledge network (topics). For simplicity, the learning process is singly parameterized by alpha as the probability of self-learning, leaving 1 - alpha as the socially-influenced discovery probability. Numerical simulations show a tradeoff of alpha on the diversity of knowledge when examined at the population level (e.g. number of distinct topics) and at the individual level (e.g. the average distance between topics for an agent), consistent across different intralayer configurations. In particular, higher values of alpha, or increased self-learning tendency, lead to higher population diversity and robustness. However, lower values of alpha, where learning/discovery is more easily influenced by social inputs, expand individual knowledge diversity more readily. These numerical results might provide some basic insights into how social influences can affect the diversity of human knowledge, especially in the age of information and social media.
更多查看译文
关键词
Topic diversity, Knowledge discovery, Bipartite network, Social influence, Self-learning
AI 理解论文
溯源树
样例
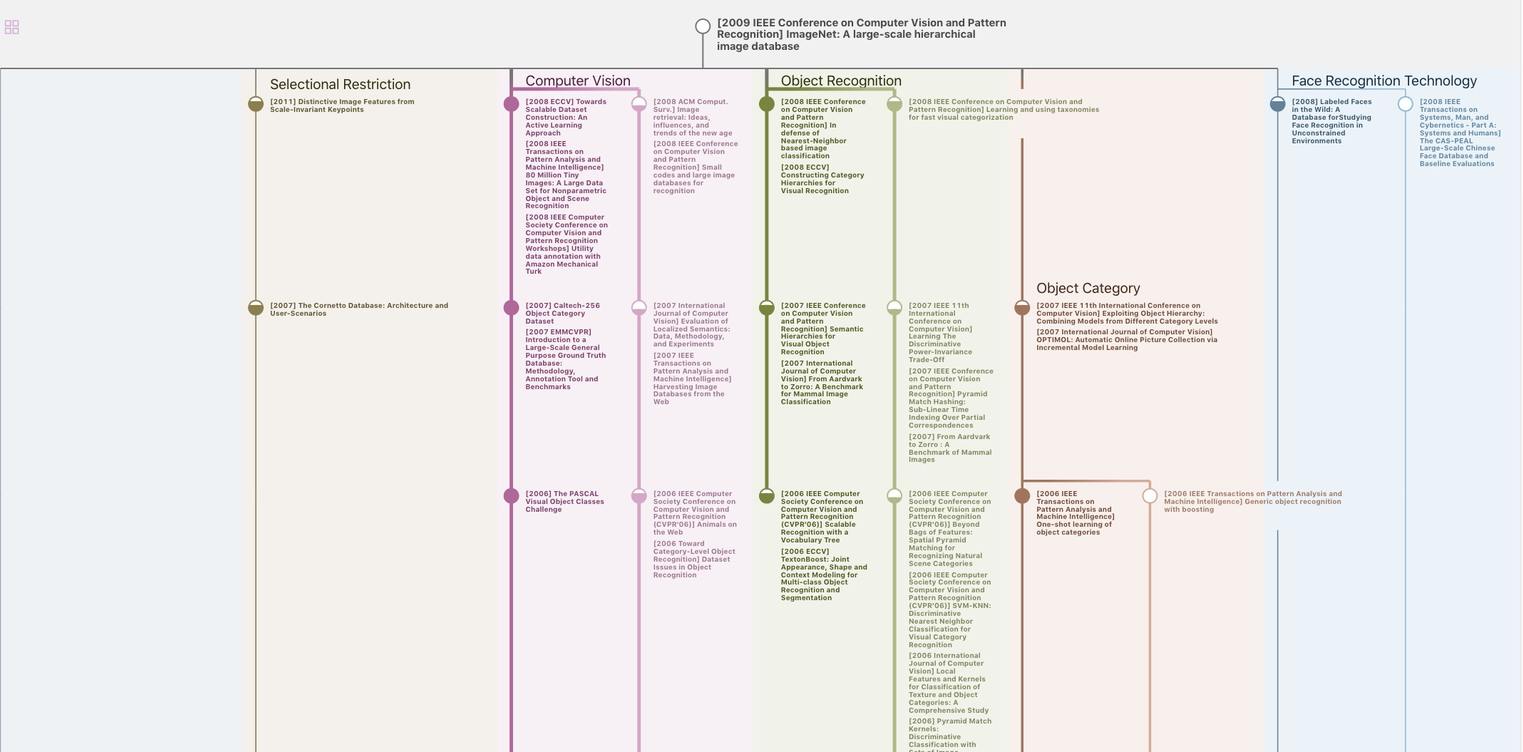
生成溯源树,研究论文发展脉络
Chat Paper
正在生成论文摘要