Can Dynamic Functional Connectivity Be Used to Distinguish Between Resting-State and Motor Imagery in EEG-BCIs?
COMPLEX NETWORKS & THEIR APPLICATIONS X, VOL 2(2022)
摘要
Graph theory has been widely and efficiently applied to characterize brain functioning, ranging from the diagnosis of pathologies (e.g. depression, Alzheimer, schizophrenia, etc.) to investigations of cognitive processes in neuroscience. Recently, the use of graph-based strategies through functional connectivity (FC) analysis has shown to be an interesting option for feature extraction in motor imagery brain-computer interfaces (MI-BCIs) - an alternative communication system that does not require the use of classical biological efferent pathways, mapping brain signals directly to control external assistive devices. Although FC has been used in such context, the dynamics of FC under motor imagery has rarely been taken into account, which outlines an essential requirement for online BCI operation. Therefore, this study aims to evaluate the applicability of dynamic functional connectivity (dFC) to differentiate resting-state and motor imagery in electroencephalography (EEG)-based BCI. We evaluated the classification performance of classical markers, as defined by event-related desynchronization, static FC and the dynamic FC scenario. The analysis includes two different similarity criteria for estimating the FC matrix and four different graph-based metrics applied to a representative EEG dataset with 35 subjects. The obtained results point to the potential and accompanying challenges of using dFC in the context of MI-BCIs, in addition to providing insights concerning the motor network activity organization during motor imagery.
更多查看译文
关键词
Brain-computer interface, Dynamic functional connectivity, Electroencephalography, Motor imagery, Time-varying graphs
AI 理解论文
溯源树
样例
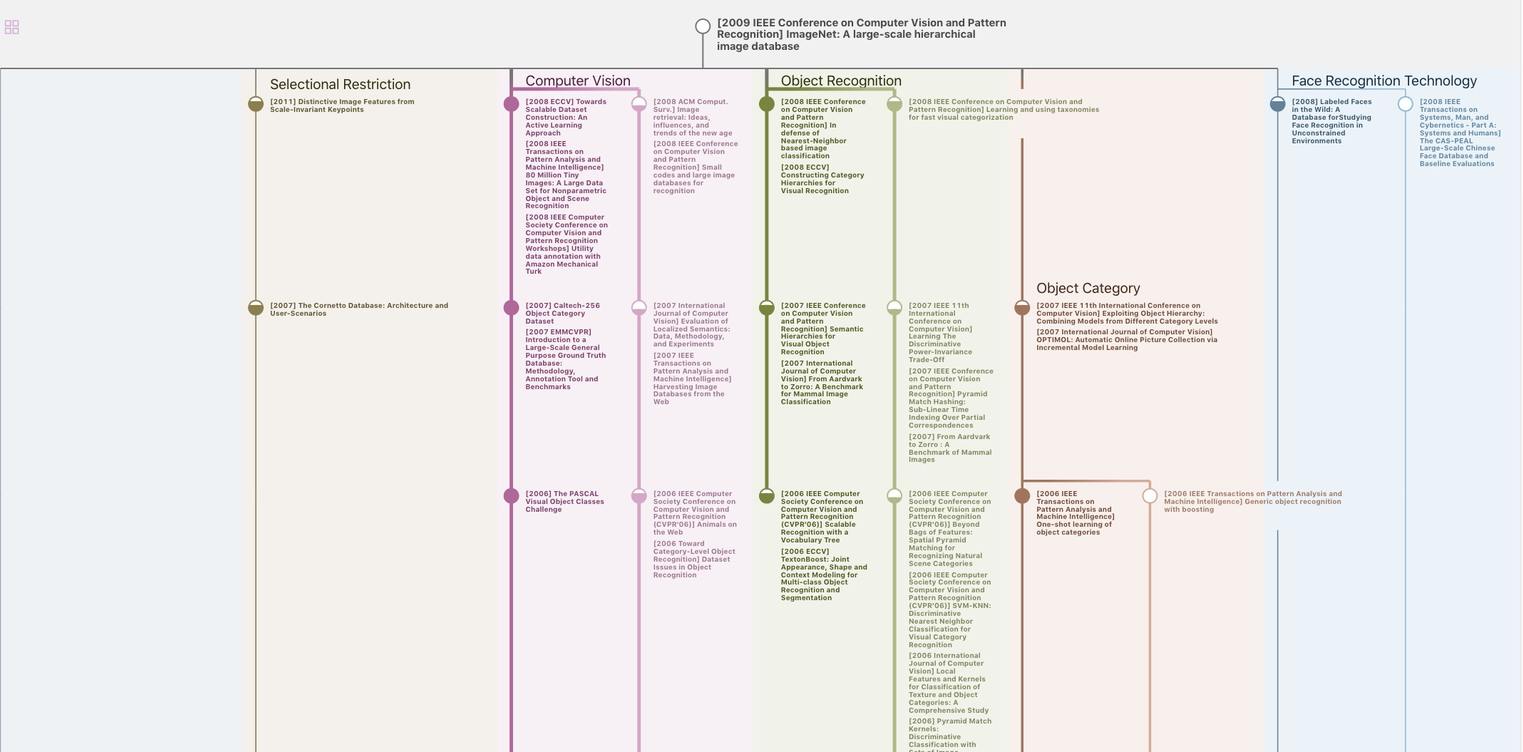
生成溯源树,研究论文发展脉络
Chat Paper
正在生成论文摘要