Machine learning modeling using process context and the exposure data for overlay prediction
METROLOGY, INSPECTION, AND PROCESS CONTROL XXXVI(2022)
摘要
Overlay is one of the critical parameters and directly impacts yield. Due to high metrology cost, only a small number of wafers are measured per lot. To this end, virtual metrology (VM) aims to provide valuable information about the nonmeasured wafers with little to no additional cost. VM leverages historical per-wafer measurements from exposure tools and processing equipment collected at previous process steps to report overlay on every wafer. As data-driven approaches gain more adoption in the semiconductor manufacturing, machine learning (ML) is a natural choice to tackle this task. In this paper, we present the strategies of learning overlay prediction models from exposure and process context data as well as the steps for achieving desired prediction performance, including data preparation, feature selection, best modeling methods, hyperparameters tuning and objective. We demonstrate our methodology on a large HVM dataset under stable APC conditions.
更多查看译文
关键词
Virtual metrology (VM), machine learning (ML), overlay prediction, Advanced Process Control (APC), exposure, process context
AI 理解论文
溯源树
样例
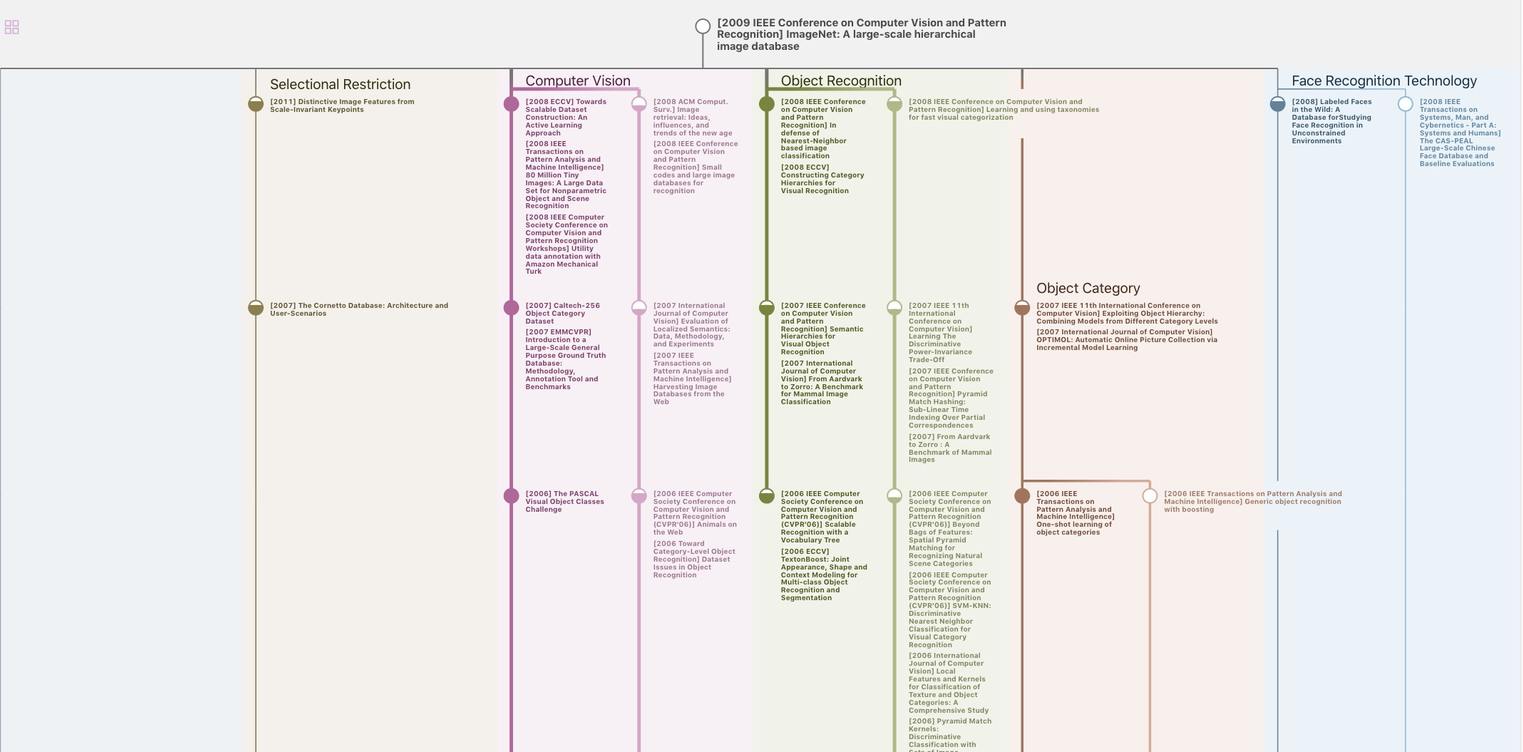
生成溯源树,研究论文发展脉络
Chat Paper
正在生成论文摘要