Classification of Dispersed Patterns of Radiographic Images with COVID-19 by Core-Periphery Network Modeling
COMPLEX NETWORKS & THEIR APPLICATIONS X, VOL 1(2022)
摘要
In real world data classification tasks, we always face the situations where the data samples of the normal cases present a well defined pattern and the features of abnormal data samples vary from one to another, i.e., do not show a regular pattern. Up to now, the general data classification hypothesis requires the data features within each class to present a certain level of similarity. Therefore, such real situations violate the classic classification condition and make it a hard task. In this paper, we present a novel solution for this kind of problems through a network approach. Specifically, we construct a core-periphery network from the training data set in such way that core node set is formed by the normal data samples and peripheral node set contains the abnormal samples of the training data set. The classification is made by checking the coreness of the testing data samples. The proposed method is applied to classify radiographic image for COVID-19 diagnosis. Computer simulations show promising results of the method. The main contribution is to introduce a general scheme to characterize pattern formation of the data "without pattern".
更多查看译文
关键词
Data classification, Core-periphery network, Dispersed class pattern, COVID-19
AI 理解论文
溯源树
样例
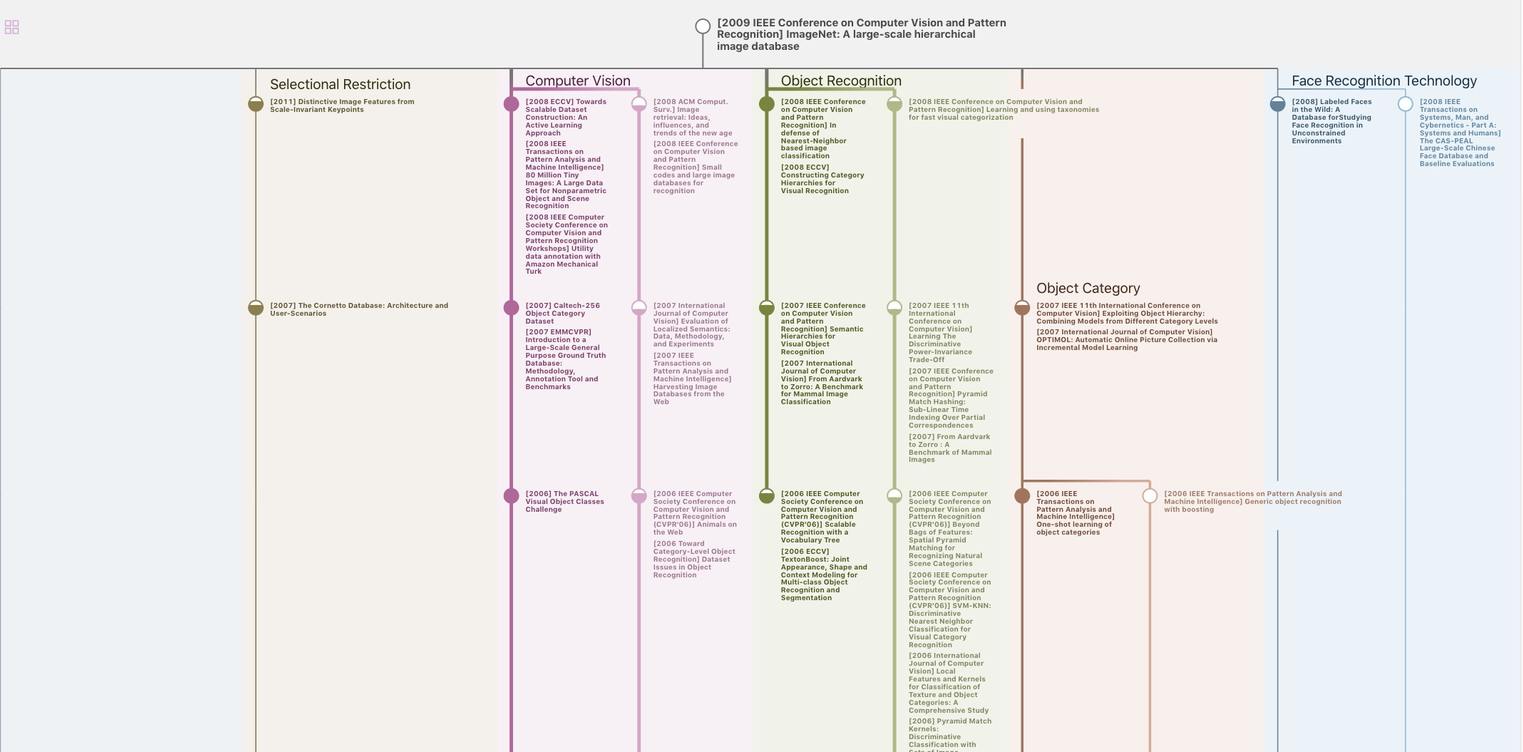
生成溯源树,研究论文发展脉络
Chat Paper
正在生成论文摘要