Energy efficient spiking neural network neuromorphic processing to enable decentralised service workflow composition in support of multi-domain operations
ARTIFICIAL INTELLIGENCE AND MACHINE LEARNING FOR MULTI-DOMAIN OPERATIONS APPLICATIONS IV(2022)
Abstract
Future Multi-Domain Operations (MDO) will require the coordination of hundreds-even thousands-of devices and component services. This will demand the capability to rapidly discover the distributed devices/services and combine them into different workflow configurations, thereby creating the applications necessary to support changing mission needs. Motivated by neuromorphic processing models, in previous work it was shown that this can be achieved by using hyperdimensional symbolic semantic vector representations of the services/devices and workflows. Using a process of vector exchange the required services are dynamically discovered and inter-connected to achieve the required tasks. In network edge environments, the capability to perform these tasks with minimum energy consumption is critical. This paper describes how emerging spiking neural network (SNN) neuromorphic processing devices can be used to perform the required hyperdimensional vector computation (HDC) with significant energy savings compared to what can be achieved using traditional CMOS implementations.
MoreTranslated text
Key words
AI,Spiking Neural Networks,Service Discovery,Workflow Composition,Hyperdimensional Computing,Vector Symbolic Architecture
AI Read Science
Must-Reading Tree
Example
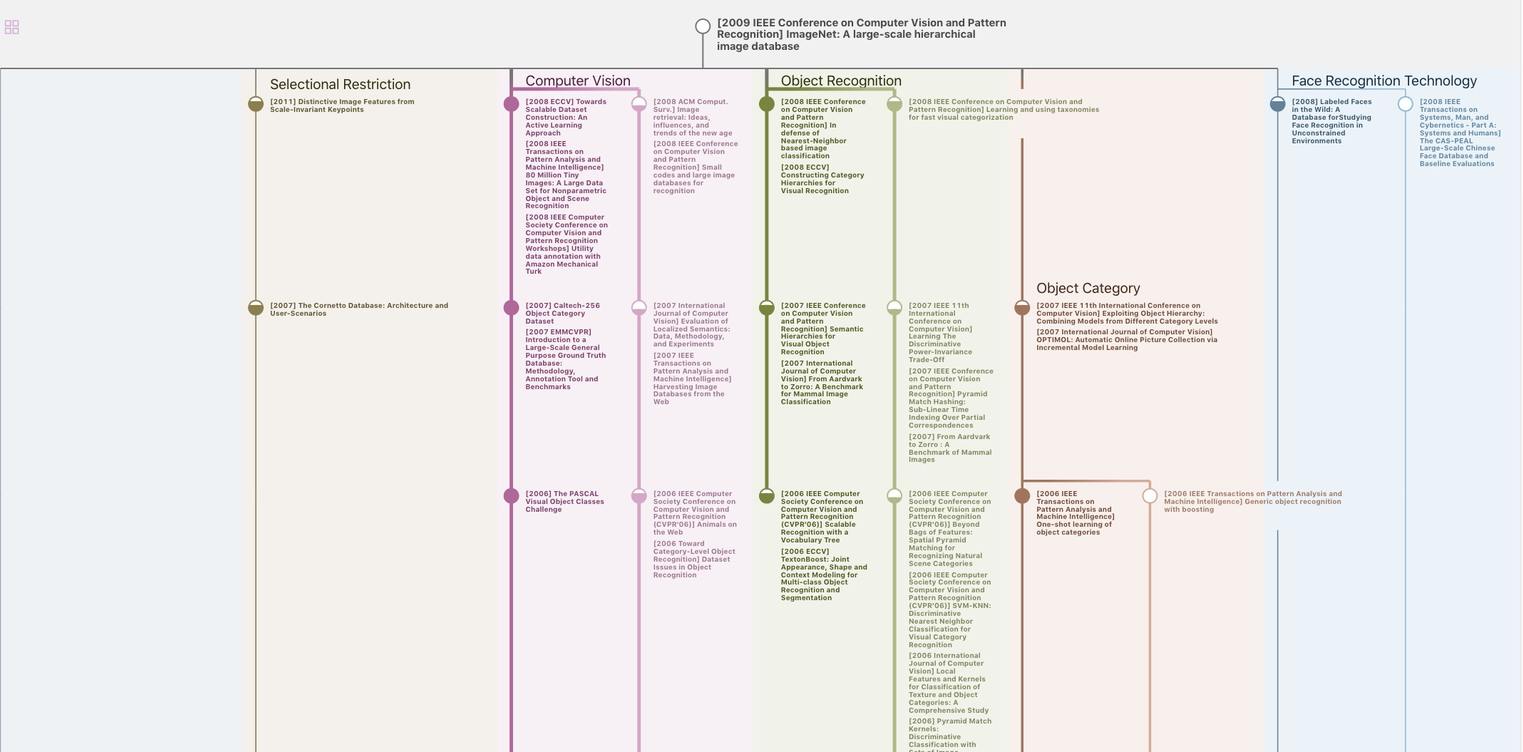
Generate MRT to find the research sequence of this paper
Chat Paper
Summary is being generated by the instructions you defined