Multi-branch neural network for hybrid metrology improvement
METROLOGY, INSPECTION, AND PROCESS CONTROL XXXVI(2022)
摘要
In the domain of advanced patterning, and especially at lithography steps achieve very small sizes becomes more and more crucial. This induces measurement challenges and thus requiring the development of new, precise and robust metrology techniques. To overcome the limited constraints of different techniques, one of the most promising approaches is hybrid metrology. It consists in gathering several metrology techniques to measure all the geometrical parameters which are processed them by an algorithm (mainly machine learning algorithm). This work stands out by using for deep learning a multi-branch neural network to increase the precision of predicts. With a particular attention made to the dataset generation and specific settings for each branch, we developed the potential of this approach which increase the precision of predicts.
更多查看译文
关键词
Hybrid dimensional metrology, Critical dimensions, Advanced lithography, Nanoscale characterization, Data fusion, Artificial intelligence, Neural Network, Multi-branch architecture
AI 理解论文
溯源树
样例
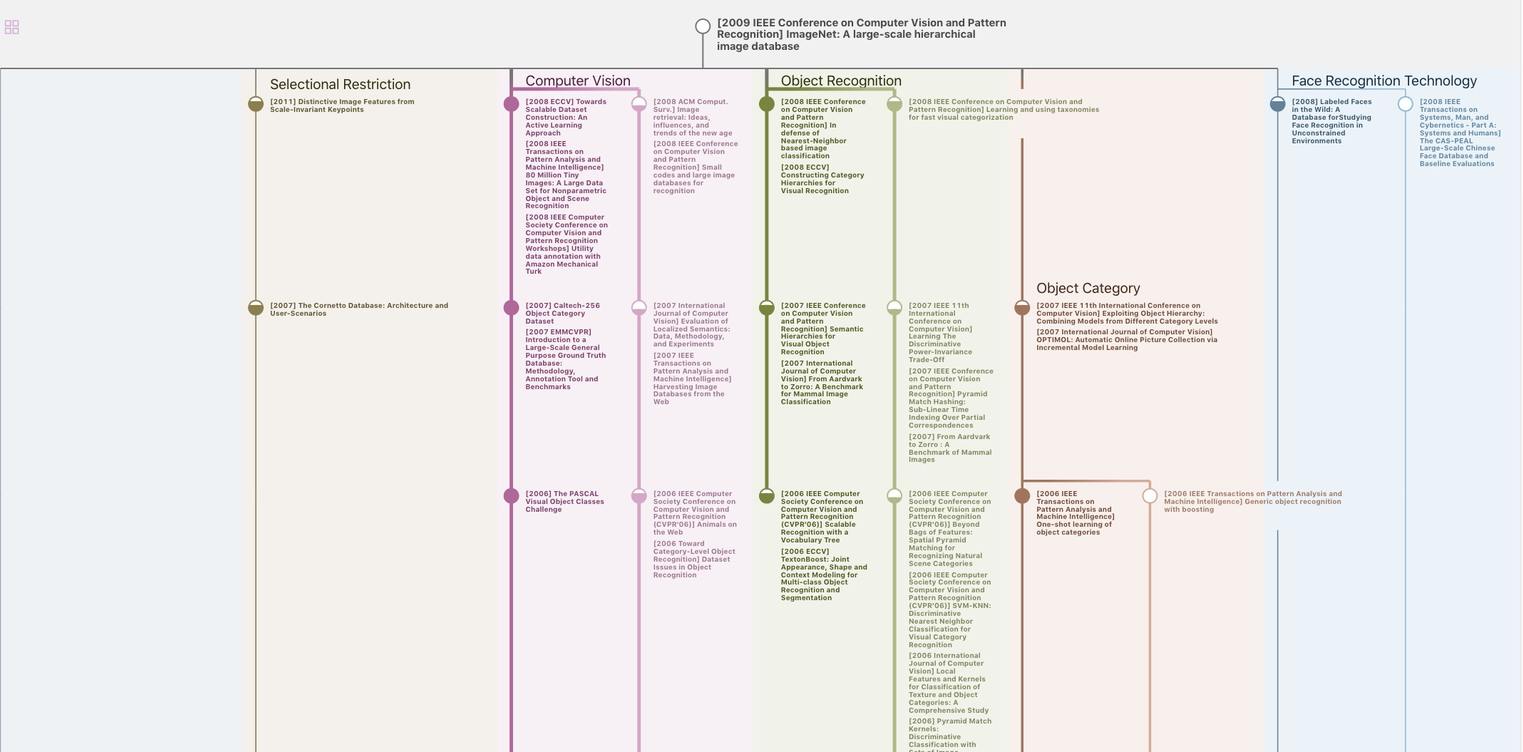
生成溯源树,研究论文发展脉络
Chat Paper
正在生成论文摘要