CCENet: Cascade Class-Aware Enhanced Network for High-Resolution Aerial Imagery Semantic Segmentation
IEEE JOURNAL OF SELECTED TOPICS IN APPLIED EARTH OBSERVATIONS AND REMOTE SENSING(2022)
摘要
Semantic segmentation of high-resolution aerial images is a challenging task on account of interclass homogeneity and intraclass heterogeneity of land cover. Recent works have sought to mitigate this issue by exploiting pixelwise global contextual information using self-attention mechanism. However, the existing attention-based methods usually generate inaccurate object boundary segmentation results, as the self-attention model is embedded in high-level features with low resolution due to prohibitively computational complexity. Moreover, existing attention-based models ignore classwise contextual information from intermediate results, which leads to undesirable feature separability. To obtain discriminative feature as well as generate accurate segmentation boundaries, we present a novel segmentation framework, named cascade class-aware enhanced network (CCENet) for high-resolution aerial imagery. The proposed CCENet predicts segmentation results on multiple stages, and the result of the previous stage is used to refine object boundary details for the latter stage. To exploit the class-aware prior information in previous stage, we propose a lightweight class-aware enhanced module (CaEM) to grab the class-aware contextual dependencies. Specifically, CaEM first extracts a set of class representation of the land covers by global class pooling block and then reconstructs enhanced features using class relation measurement, which alleviates the interclass homogeneity and intraclass heterogeneity of ground objects in feature space. Quantitative and qualitative experimental results on three publicly available datasets demonstrate the superiority of our CCENet over other state-of-the-art methods in the items of high labeling accuracy and computation efficiency.
更多查看译文
关键词
Image segmentation, Semantics, Spatial resolution, Kernel, Feature extraction, Convolutional neural networks, Convolution, Aerial imagery, class context information, deep convolution neural networks, self-attention mechanism, semantic segmentation
AI 理解论文
溯源树
样例
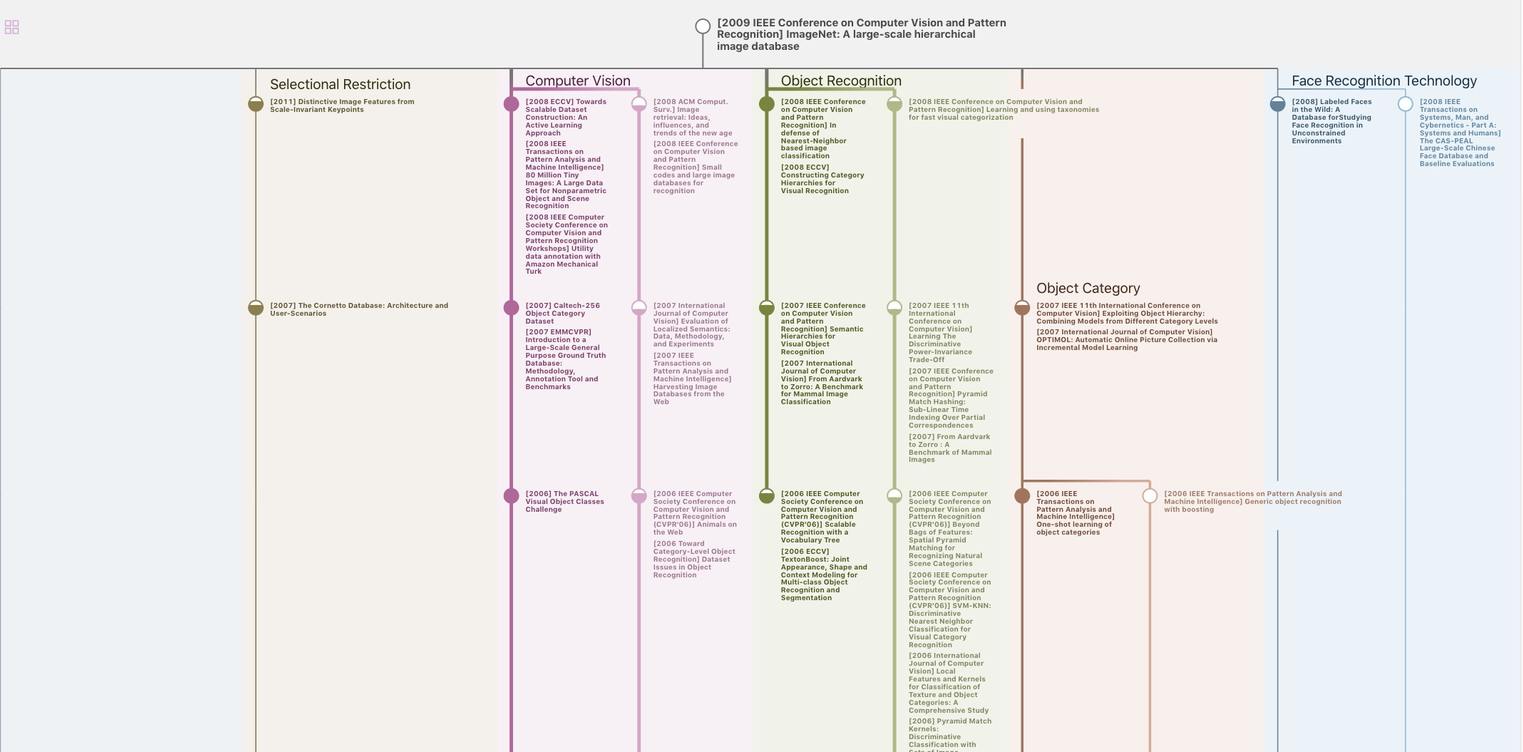
生成溯源树,研究论文发展脉络
Chat Paper
正在生成论文摘要