Machine Learning for analysis of speckle dynamics: quantification and outlier detection
PHYSICAL REVIEW RESEARCH(2022)
摘要
X-ray photon correlation spectroscopy (XPCS) provides an understanding of complex dynamics in materials that are tied to their synthesis, properties, and behaviors. Analysis of XPCS data for dynamics that are far from equilibrium is labor intense and often can impede the discovery process, especially in experiments with high collection rates. Moreover, binning and averaging, involved in the analysis for alleviating poor signal-tonoise ratio, leads to a loss of temporal resolution and the accumulation of systematic error for the parameters quantifying the dynamics. Here, we integrate a denoising autoencoder model into workflows for the analysis of nonequilibrium two-time intensity-intensity correlation functions. Noise reduction allows for extracting the parameters that characterize the sample's dynamics with the temporal resolution limited only by frame rates. Not only does it improve the quantitative usage of the data, but it also creates the potential for automating the analytical workflow, which is a key to high-throughput or autonomous XPCS experiments. Various approaches for the uncertainty quantification and extension of the model for anomalies' detection are discussed.
更多查看译文
AI 理解论文
溯源树
样例
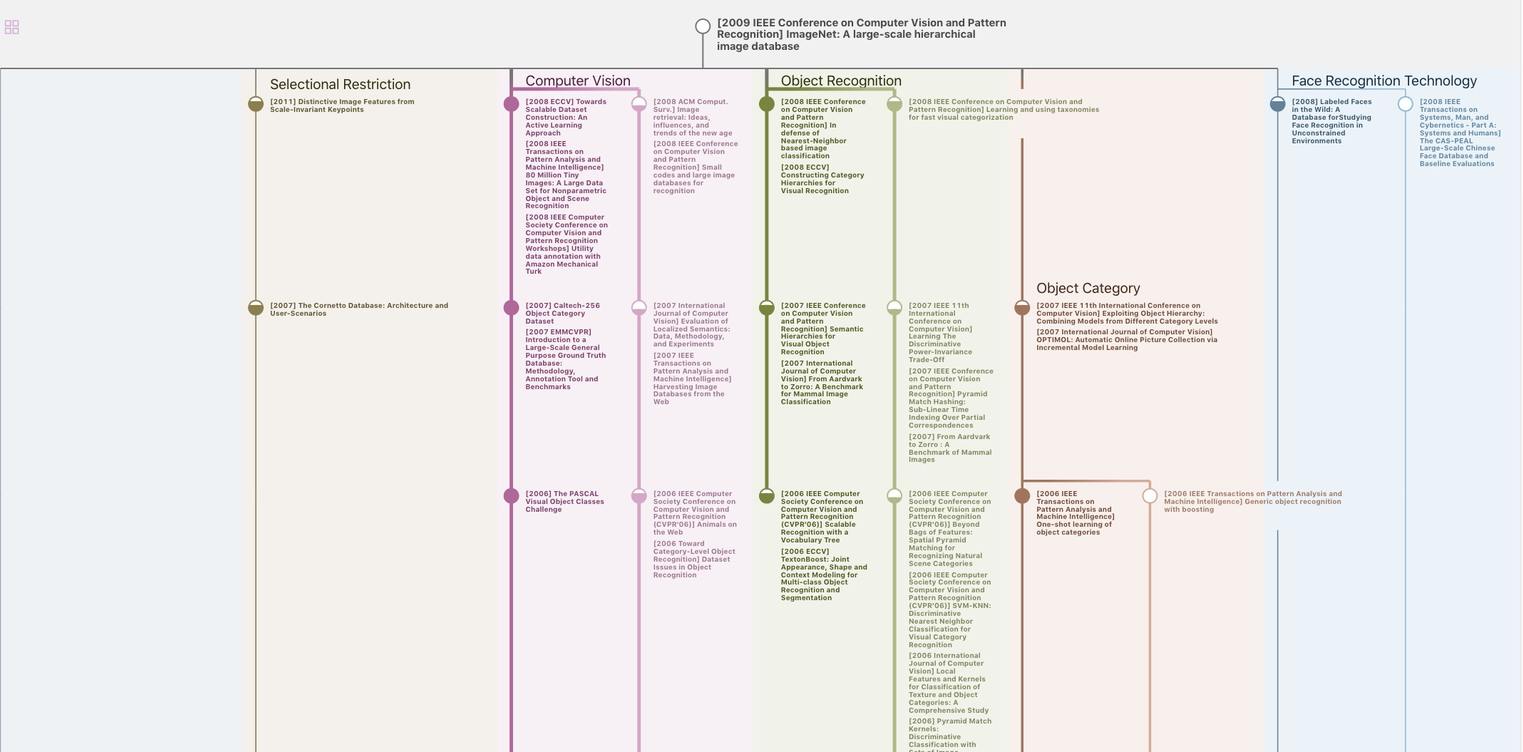
生成溯源树,研究论文发展脉络
Chat Paper
正在生成论文摘要