A Dynamic Deep-learning Model for Generating a Magnetogram Sequence from an SDO/AIA EUV Image Sequence
ASTROPHYSICAL JOURNAL SUPPLEMENT SERIES(2022)
摘要
The solar magnetic field dominates solar activities in the solar atmosphere, such as solar flares and coronal mass ejections (CMEs). The Helioseismic and Magnetic Imager (HMI) on board the Solar Dynamics Observatory (SDO) has been in operation from 2010, providing a full-disk photospheric magnetogram. However, with a single view of observation, SDO/HMI cannot provide a global view of the Sun at the same time, so the farside of the Sun is blind to us. The Solar Terrestrial Relations Observatory (STEREO) provides two different views of the Sun with complementary viewing angles relative to SDO/HMI. However, it did not carry a magnetograph, but an extreme-ultraviolet (EUV) imager. Fortunately, deep learning has been proved to generate a solar farside magnetogram from STEREO farside EUV observation. Although a single generated magnetogram is morphologically very similar to ground truth, the sequence of the generated magnetogram has noticeable magnetic field fluctuation, which cannot be ignored when it is displayed as a time series, especially at an active region. This fluctuation is represented by sudden magnetic polarity reversal and drifting of magnetic field distribution. To mitigate this problem, a novel dynamic deep-learning model by integrating a convolutional gated recurrent units (convGRU) model into a pix2pix baseline is proposed in this paper. It can generate a sequence of a magnetogram with smooth transition among consecutive magnetograms by exploring spatio-temporal information of an input EUV image sequence. From both quantitative and qualitative comparisons, the proposed model can generate a magnetogram sequence more close to real observation.
更多查看译文
关键词
sdo/aia euv image sequence,magnetogram sequence,deep-learning deep-learning,dynamic
AI 理解论文
溯源树
样例
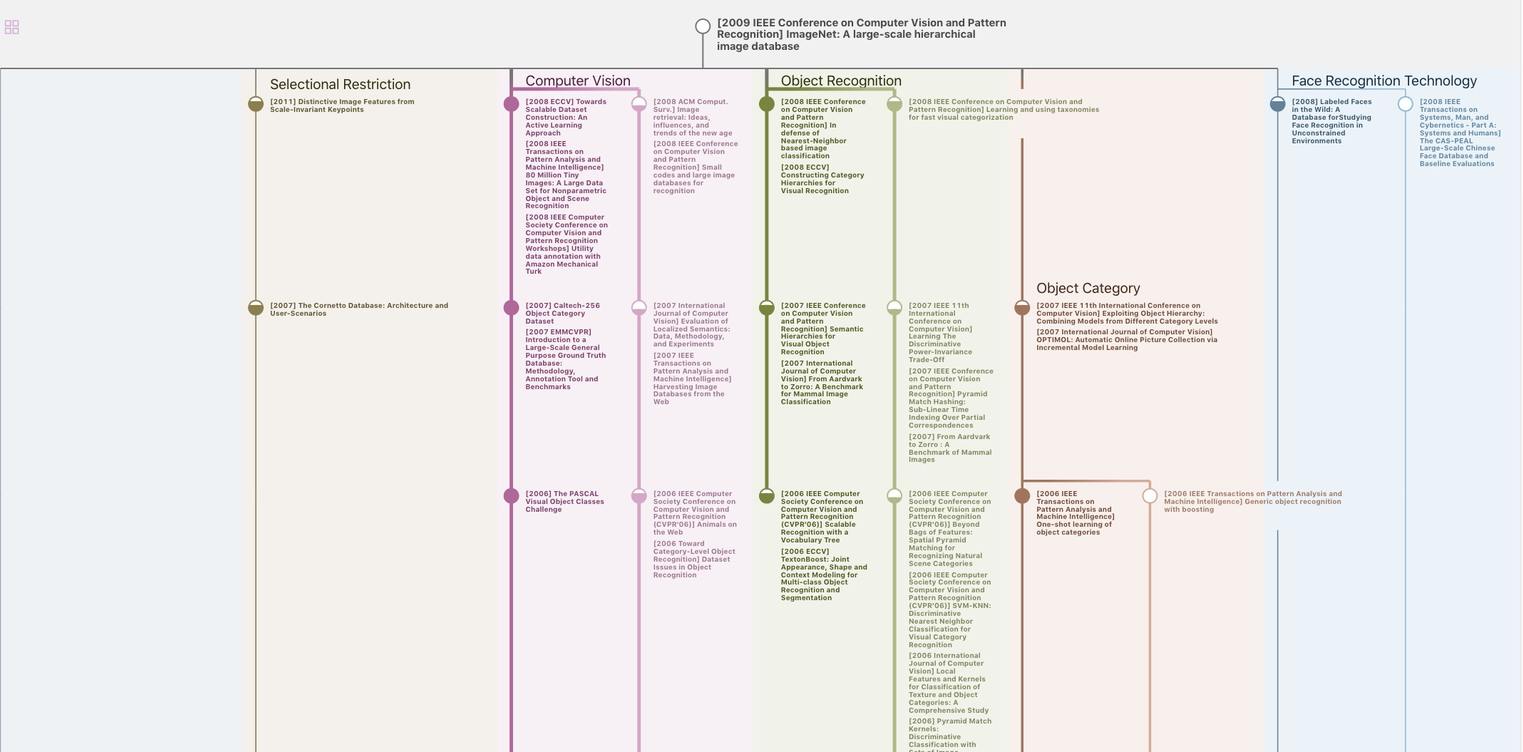
生成溯源树,研究论文发展脉络
Chat Paper
正在生成论文摘要