ONE DAY AHEAD PREDICTION OF PM2.5 SPATIAL DISTRIBUTION USING MODIS 3 KM AOD AND SPATIOTEMPORAL MODEL OVER BEIJING-TIANJIN-HEBEI, CHINA
XXIV ISPRS CONGRESS: IMAGING TODAY, FORESEEING TOMORROW, COMMISSION III(2022)
摘要
Accurate prediction of PM2.5 concentration is the premise and guarantees to effectively control PM2.5 concentration and avoid the adverse effects of high PM2.5 concentration on human health. However, given the existing statistical models can only predict the pollutant concentration at the monitoring sites, the spatial distribution of PM2.5 concentration cannot be predicted, which greatly limits the application of the model in PM2.5 concentration prediction. This study combined the PM2.5 spatial distribution data predicted using the Moderate Resolution Imaging Spectroradiometer 3 km aerosol optical depth (AOD) and meteorological factors into a spatiotemporal autoregressive (STAR) model to predict the regional PM2.5 concentration and quantify the short-term spatial distribution change of PM2.5 in Beijing-Tianjin-Hebei region (JingJinJi) one day in advance. Five simulation functions were used to simulate the STAR model, and the 2014 data of JingJinJi were used to verify its accuracy. Results showed that the STAR model had the best prediction performance when gradient boosting decision tree was used as the simulation function compared with other simulation functions. The coefficient of determination (R-2), root mean square prediction error (RMSE), index of agreement (IA), and mean absolute error (MAE) of the STAR model were 0.85, 27.08 mu g/m(3), 0.96, and 20 mu g/m(3), respectively. The spatial distribution prediction results of PM2.5 showed that the +1-day PM2.5 spatial distribution prediction results were in good agreement with the PM2.5 spatial distribution results predicted by AOD to provide accurate spatiotemporal distribution data for reducing air pollution and air pollution early warning.
更多查看译文
关键词
MODIS AOD, PM2.5, PM2.5 spatiotemporal distribution, Spatiotemporal autoregressive model, Machine learning model
AI 理解论文
溯源树
样例
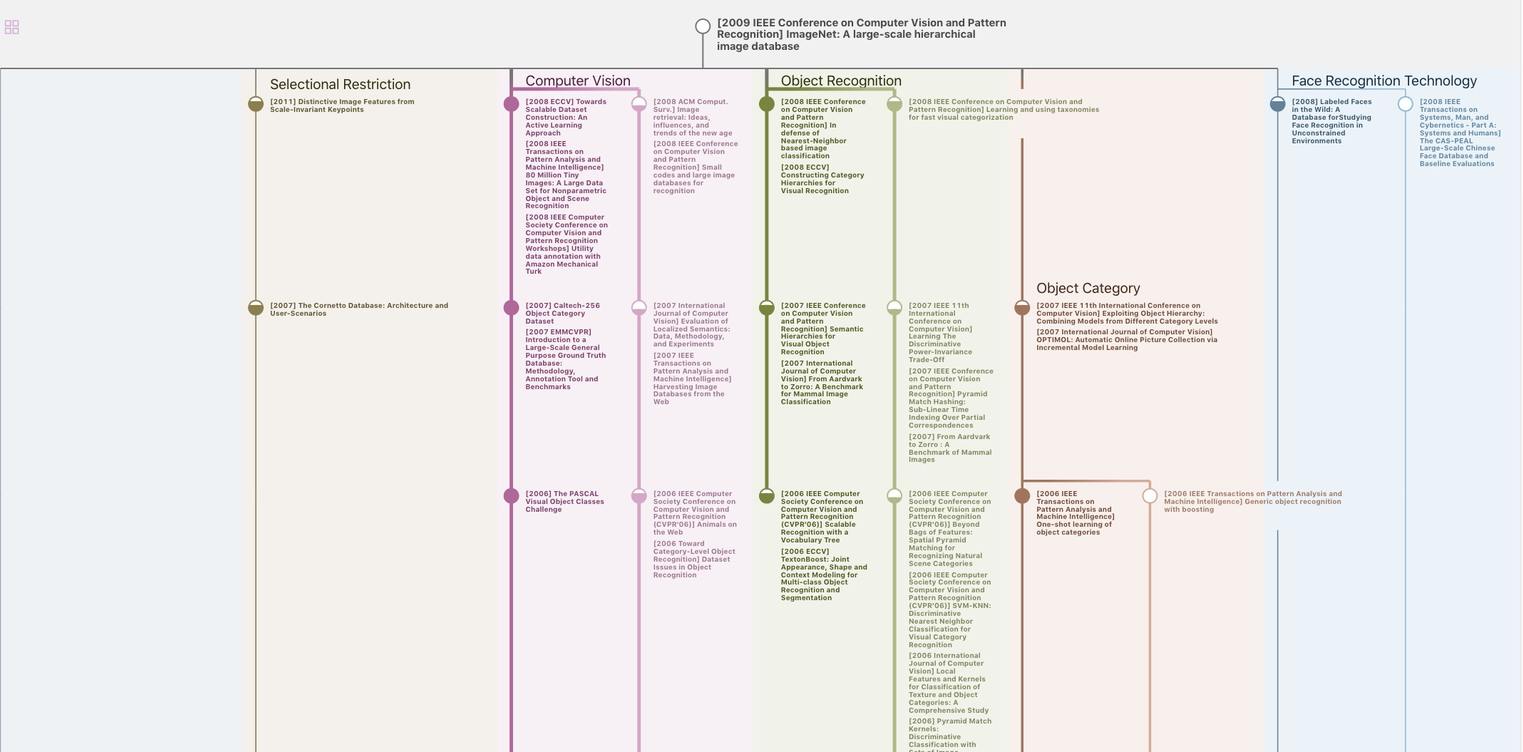
生成溯源树,研究论文发展脉络
Chat Paper
正在生成论文摘要