Progressive, Extrapolative Machine Learning for Near-Wall Turbulence Modeling
Physical review fluids(2022)
摘要
Conventional empirical turbulence modeling is progressive: one begins by modeling simple flows and progressively works towards more complex ones. The outcome is a series of nested models, with the next, more complex model accounting for some additional physics relative to the previous, less complex model. The above, however, is not the philosophy of data-enabled turbulence modeling. Data-enabled modeling is one stop: one trains against a group of data, which contains simple and complex flows. The resulting model is the best fit of the training data but does not closely reproduce any particular flow. The differences between the two modeling approaches have left data-enabled models open to criticism: machine learned models do not fully preserve, e.g., the law of the wall (among other empirical facts), and they do not generalize to, e.g., high Reynolds numbers (among other conditions). The purpose of this paper is to respond to and resolve some of these criticisms: we intend to show that the conventional progressive modeling is compatible with data-enabled modeling. The paper hinges on the extrapolation theorem and the neutral neural network theorem. The extrapolation theorem allows us to control a network's behavior when extrapolating and the neutral neural network theorem allows us to augment a network without "catastrophic forgetting." For demonstration purposes, we successively model the flow in the constant stress layer, which is simple; the flow in a channel and a boundary layer, which is more complex; and wall-bounded flow with system rotation, which is even more complex. We show that the more complex models respect the less complex models, and that the models preserve the known empiricism.
更多查看译文
AI 理解论文
溯源树
样例
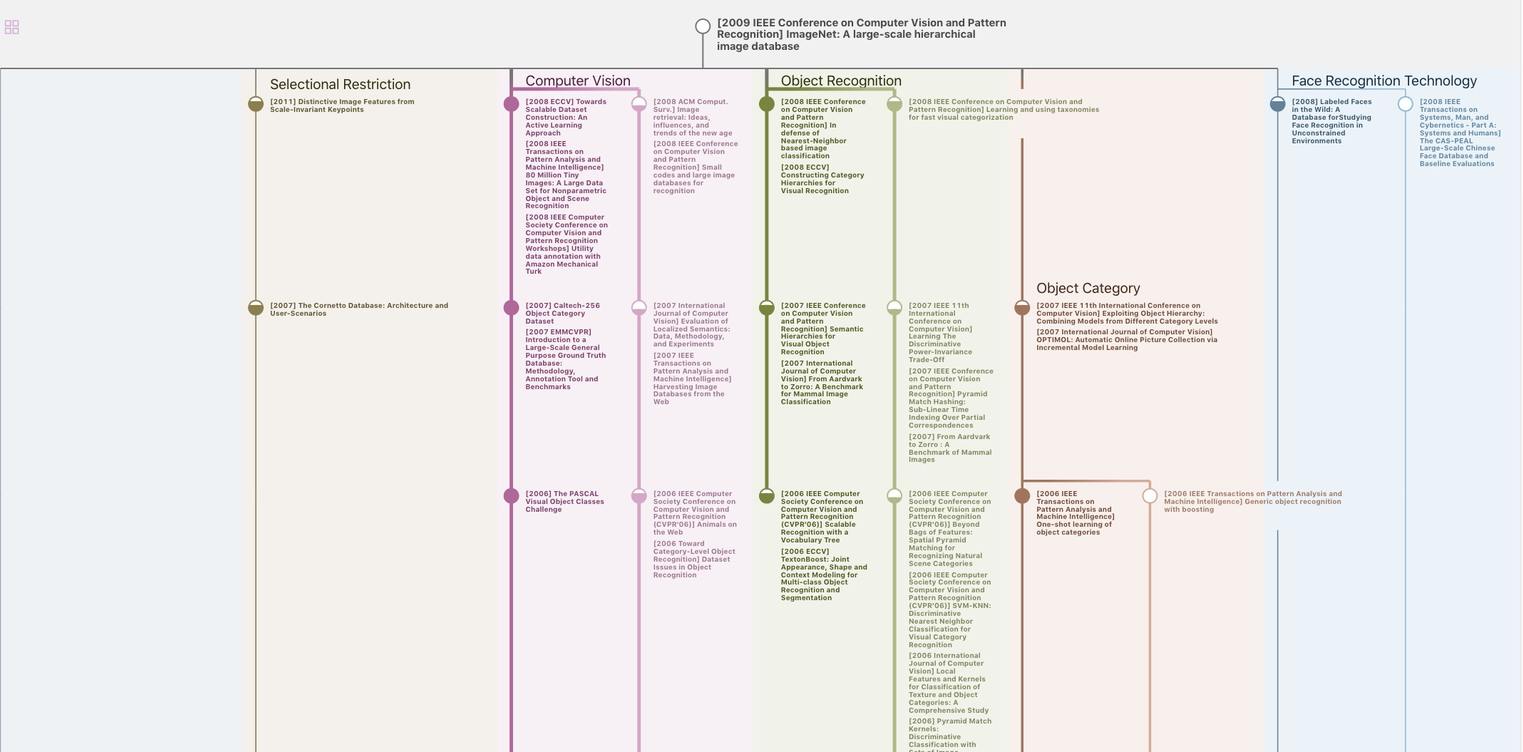
生成溯源树,研究论文发展脉络
Chat Paper
正在生成论文摘要