Triple Attention-based deep convolutional recurrent network for soft sensors
MEASUREMENT(2022)
Abstract
Soft sensors have been widely applied in industrial processes, where exist nonstationary conditions, spatial correlations, and temporal dependencies. Without considering these characteristics, many previous methods failed to adaptively extract the characteristics of process data. To handle the abovementioned issues in soft sensor modeling, a triple attention-based deep convolutional recurrent network (TADCRN) is proposed in this paper. Firstly, multiscale 1d-CNN with scale-wise attention is designed to learn critical features from multiple receptive fields to eliminate the adverse influence of nonstationary characteristics. Secondly, space-wise attention is uti-lized to extract spatial correlations among multiple sensors. Thirdly, BiGRU with time-wise attention is designed to capture temporal dependencies among the consecutively collected data samples. To verify the effectiveness and efficiency of the proposed method, the experiments were conducted on the debutanizer column. The experimental results show that the proposed method outperforms both the conventional machine learning and deep learning methods.
MoreTranslated text
Key words
Soft sensor, Deep learning, One-dimensional convolutional neural network, Bidirectional gated recurrent unit, Triple attention
AI Read Science
Must-Reading Tree
Example
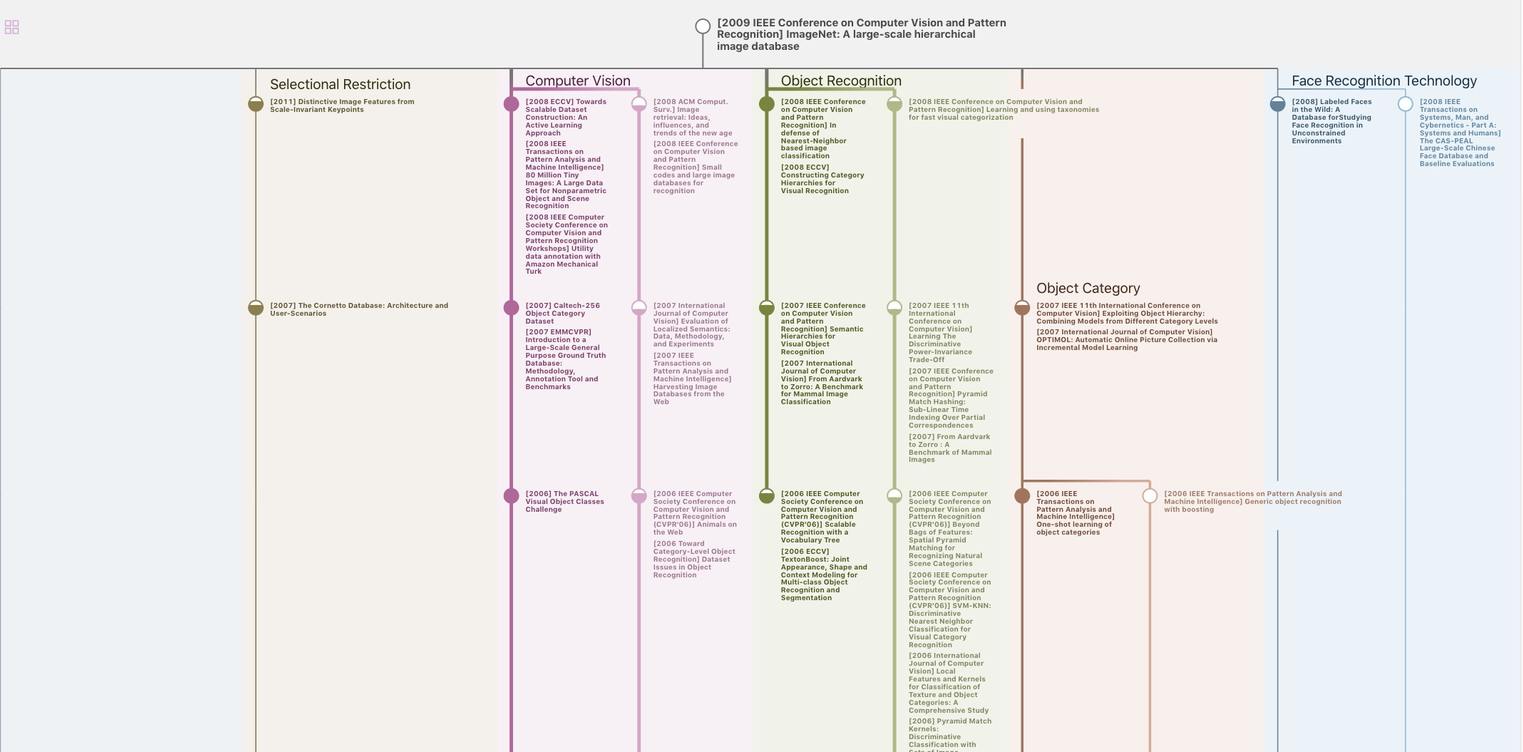
Generate MRT to find the research sequence of this paper
Chat Paper
Summary is being generated by the instructions you defined