Soil Spectroscopy with the Gaussian Pyramid Scale Space
Geoderma(2022)
摘要
Soil visible-near infrared (vis–NIR) spectra are complex and modeling soil properties can be challenging. They can suffer from additive and multiplicative noise, they are hyper-dimensional and highly collinear, making their analyses and interpretation sometimes difficult. Here, we introduce the Gaussian pyramid scale space as a multi-resolution approach for denoising spectra, reducing dimensionality, and improving the interpretability and accuracy of spectroscopic machine learning. We also used the approach to analyse contextual interactions between different resolutions and the stability of feature importance across different resolutions. Using an Australian data set and the German data in the LUCAS spectral database we found that with a single Gaussian scale that represents a relatively coarse spectral resolution, we could estimate organic carbon, clay and pH as accurately as with multiple Gaussian scales, or using all resolutions. This indicates that, in the vis–NIR range, there are no relevant interactions between resolutions, which simplifies interpretations. We conclude that the Gaussian pyramid scale space can help to model soil properties with spectral machine learning, improving both accuracy and interpretability. Because the Gaussian pyramid approach is computationally efficient, it can also be used for preprocessing and knowledge discovery before more elaborate modeling is applied.
更多查看译文
关键词
Gaussian pyramid scale space,Soil spectroscopy,Feature engineering,Feature importance,Interpretable machine learning
AI 理解论文
溯源树
样例
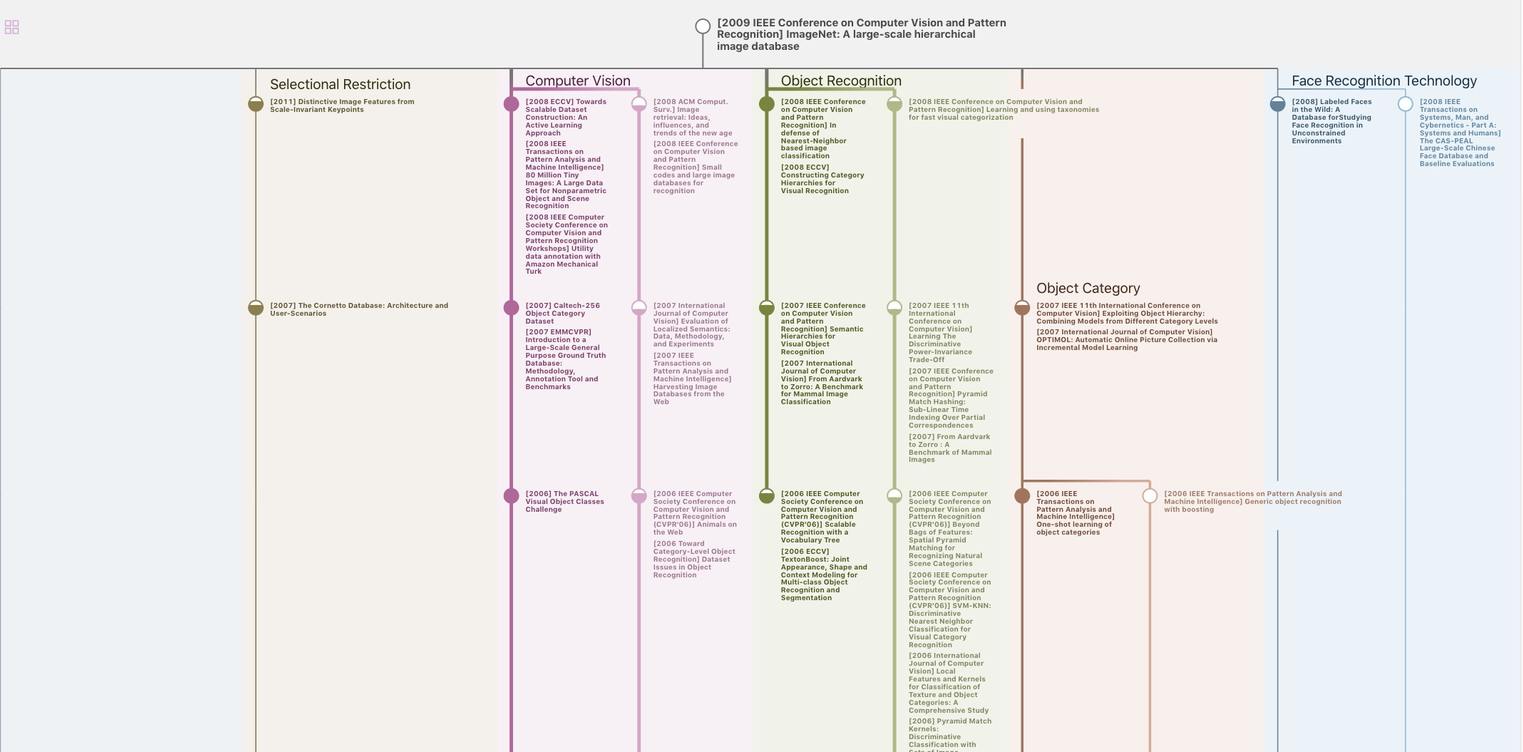
生成溯源树,研究论文发展脉络
Chat Paper
正在生成论文摘要