Residual Policy Learning Facilitates Efficient Model-Free Autonomous Racing
IEEE ROBOTICS AND AUTOMATION LETTERS(2022)
摘要
Motion planning for autonomous racing is a challenging task due to the safety requirement while driving aggressively. Most previous solutions utilize the prior information or depend on complex dynamics modeling. Classical model-free reinforcement learning methods are based on random sampling, which severely increases the training consumption and undermines the exploration efficiency. In this letter, we propose an efficient residual policy learning method for high-speed autonomous racing named ResRace, which leverages only the real-time raw observation of LiDAR and IMU for low-latency obstacle avoiding and navigation. We first design a controller based on the modified artificial potential field (MAPF) to generate a policy for navigation. Besides, we utilize the deep reinforcement learning (DRL) algorithm to generate a residual policy as a supplement to obtain the optimal policy. Concurrently, the MAPF policy effectively guides the exploration and increases the update efficiency. This complementary property contributes to the fast convergence and few required resources of our method. We also provide extensive experiments to illustrate our method outperforms the leading algorithms and reaches the comparable level of professional human players on the five F1Tenth tracks.
更多查看译文
关键词
Autonomous vehicle navigation, motion and path planning, reinforcement learning
AI 理解论文
溯源树
样例
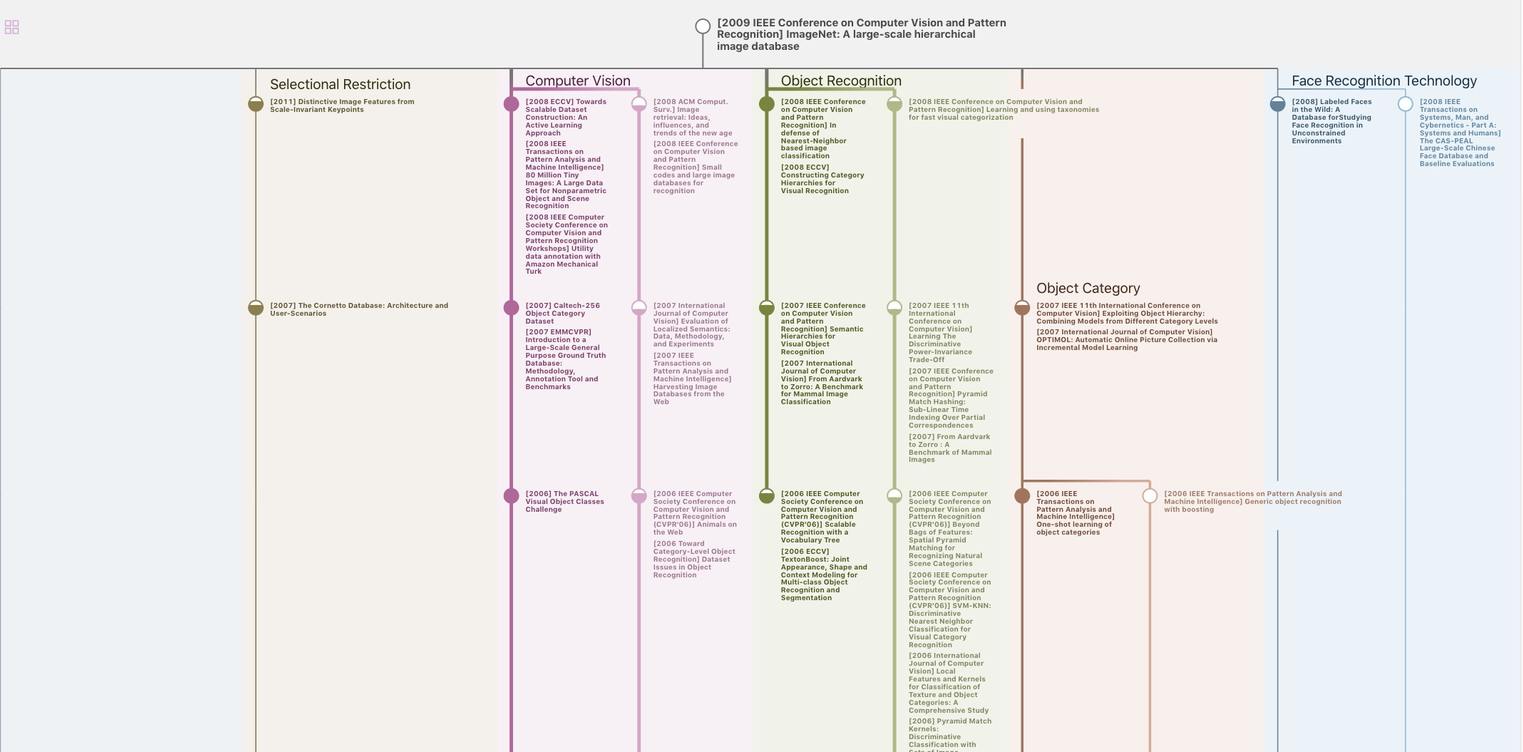
生成溯源树,研究论文发展脉络
Chat Paper
正在生成论文摘要