One-Shot Object Detection in Heterogeneous Artwork Datasets
2022 Eleventh International Conference on Image Processing Theory, Tools and Applications (IPTA)(2022)
摘要
Christian archeologists face many challenges in understanding visual narration through artwork images. This understanding is essential to access underlying semantic in-formation. Therefore, narrative elements (objects) need to be labeled, compared, and contextualized by experts, which takes an enormous amount of time and effort. Our work aims to reduce labeling costs by using one-shot object detection to generate a labeled database from unannotated images. Novel object categories can be defined broadly and annotated using visual examples of narrative elements without training exclusively for such objects. In this work, we propose two ways of using contextual information as data augmentation to improve the detection performance. Furthermore, we introduce a multi-relation detector to our framework, which extracts global, local, and patch-based relations of the image. Additionally, we evaluate the use of contrastive learning. We use data from Christian archeology (CHA) and art history - IconArt-v2 (IA). Our context encoding approach improves the typical fine-tuning approach in terms of mean average precision (mAP) by about 3.5 % (4 %) at 0.25 intersection over union (IoU) for UnSeen categories, and 6 % (1.5 %) for Seen categories in CHA (IA). To the best of our knowledge, our work is the first to explore few shot object detection on heterogeneous artistic data by investigating evaluation methods and data augmentation strategies. We will release the code and models after acceptance of the work.
更多查看译文
关键词
one-shot,object detection,digital humanities,computational humanities,data augmentation
AI 理解论文
溯源树
样例
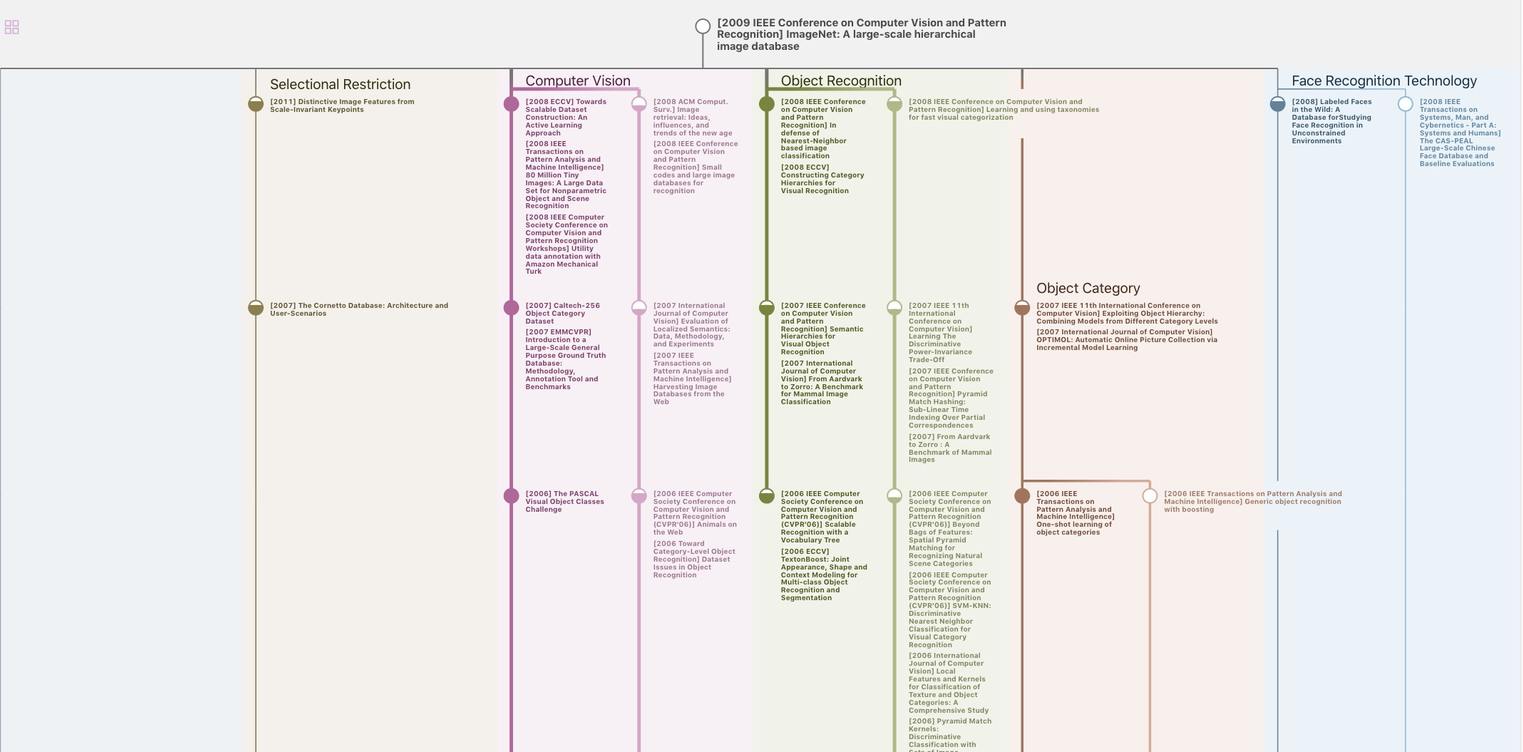
生成溯源树,研究论文发展脉络
Chat Paper
正在生成论文摘要