Semantic Masking: A Novel Technique to Mitigate the Class-Imbalance Problem in Real-Time Semantic Segmentation
2022 National Conference on Communications (NCC)(2022)
摘要
In the field of computer vision and scene under-standing, semantic segmentation is considered to be one of the most challenging task. This is due to the fact that it has to solve all the three standard vision problems, multi-class classification, object detection and image segmentation. One of the most promising areas of application of semantic segmentation is autonomous driving. The advent of deep-learning and the availability of large-scale datasets has enabled the research com-munity to reach to unprecedented performance heights compared to traditional machine learning algorithms. However, despite all the progress, existing learning methods still face the problem of class-imbalance because of which large classes get more attention and consequently the network becomes biased towards them. The problem of class-imbalance is particularly more prominent in urban road-scene datasets. This is because the layout of the scene captured by the camera mounted on a fixed location, naturally causes certain less important classes to occupy more area in the dataset. Trees, sky and buildings are some of the examples of large classes which frequently occur and occupy large areas despite the fact that they are less important with regards to driving related decision making. To tackle this problem, in this work, we have done the statistical analysis of the famous Cityscapes dataset to uncover the hidden patterns that large and small classes follow. Based on these patterns, we propose a semantic masking technique, that enables our proposed network MaskNet to pay more attention to regions where the smaller classes are more likely to occur. In this way, we see a significant performance increase with regards to smaller classes and the problem of class-imbalance is mitigated to a good extent.
更多查看译文
关键词
Semantic Segmentation,Class Imbalance,Deep Learning,Autonomous Driving
AI 理解论文
溯源树
样例
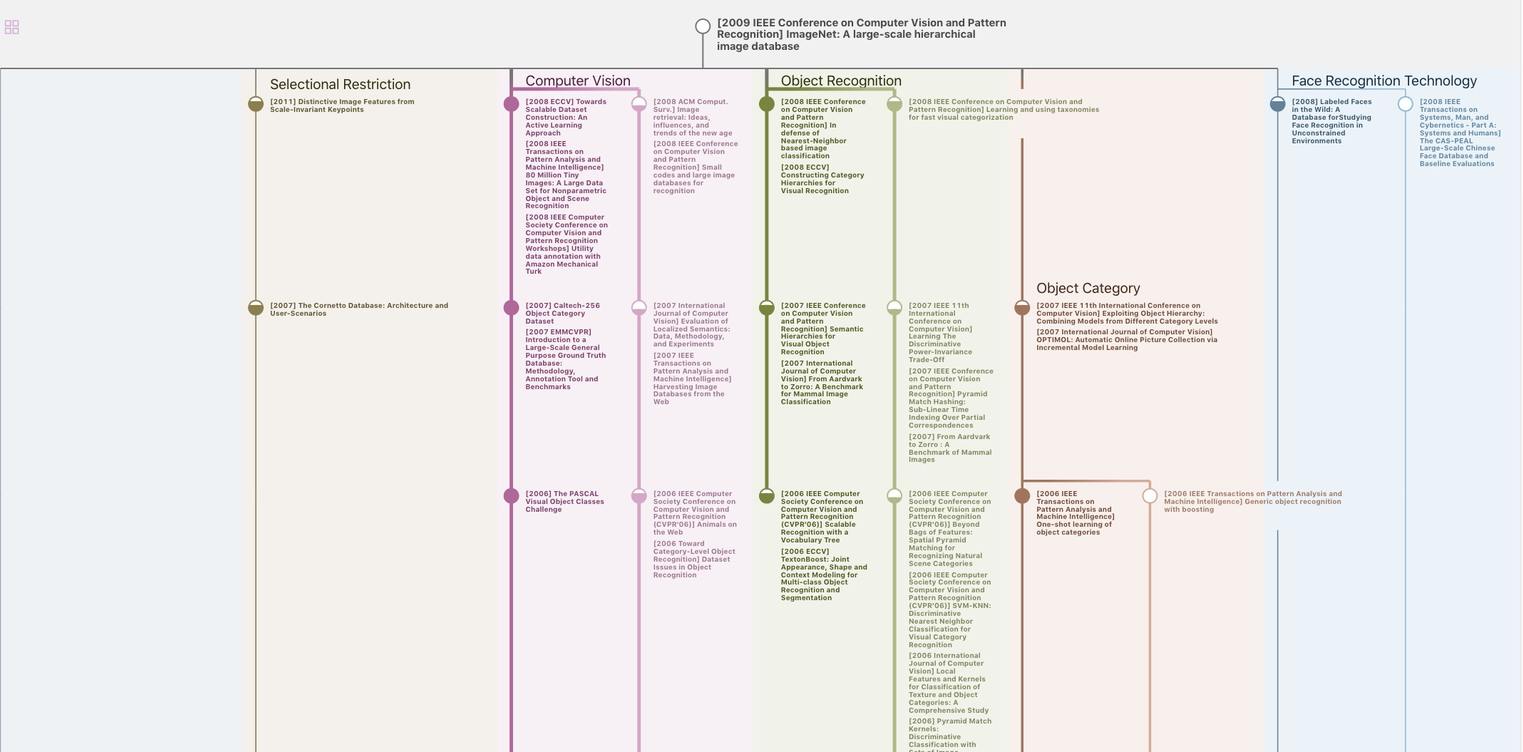
生成溯源树,研究论文发展脉络
Chat Paper
正在生成论文摘要