Triggers and Tweets: Implicit Aspect-Based Sentiment and Emotion Analysis of Community Chatter Relevant to Education Post-COVID-19
BIG DATA AND COGNITIVE COMPUTING(2022)
摘要
This research proposes a well-being analytical framework using social media chatter data. The proposed framework infers analytics and provides insights into the public's well-being relevant to education throughout and post the COVID-19 pandemic through a comprehensive Emotion and Aspect-based Sentiment Analysis (ABSA). Moreover, this research aims to examine the variability in emotions of students, parents, and faculty toward the e-learning process over time and across different locations. The proposed framework curates Twitter chatter data relevant to the education sector, identifies tweets with the sentiment, and then identifies the exact emotion and emotional triggers associated with those feelings through implicit ABSA. The produced analytics are then factored by location and time to provide more comprehensive insights that aim to assist the decision-makers and personnel in the educational sector enhance and adapt the educational process during and following the pandemic and looking toward the future. The experimental results for emotion classification show that the Linear Support Vector Classifier (SVC) outperformed other classifiers in terms of overall accuracy, precision, recall, and F-measure of 91%. Moreover, the Logistic Regression classifier outperformed all other classifiers in terms of overall accuracy, recall, an F-measure of 81%, and precision of 83% for aspect classification. In online experiments using UAE COVID-19 education-related data, the analytics show high relevance with the public concerns around the education process that were reported during the experiment's timeframe.
更多查看译文
关键词
public well-being, education process, sentiment analysis, emotion analysis, aspect-based sentiment analysis, Twitter
AI 理解论文
溯源树
样例
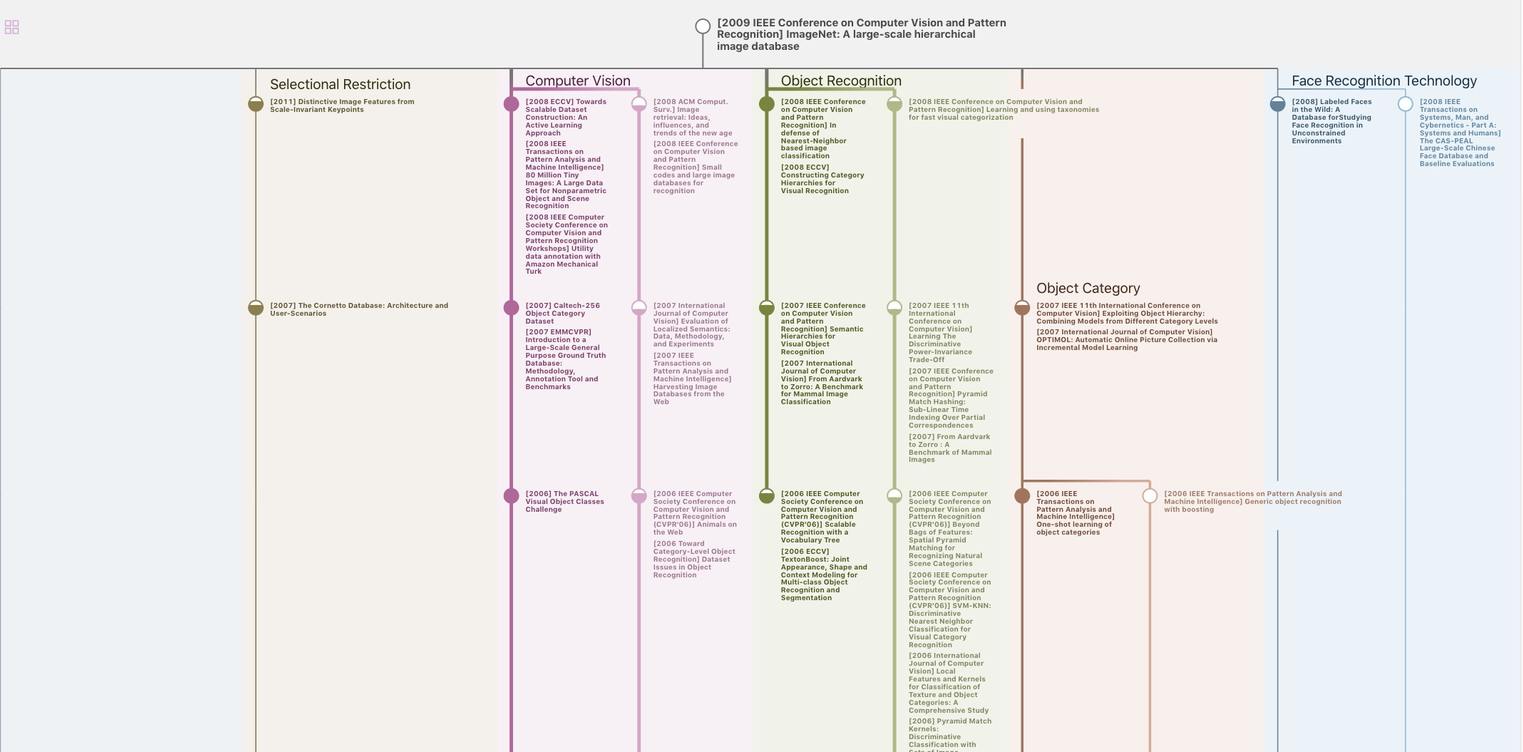
生成溯源树,研究论文发展脉络
Chat Paper
正在生成论文摘要