A Two-Sided Matching Decision-Making Approach with Correlative Criteria Under Probabilistic Linguistic Environment
INTERNATIONAL JOURNAL OF INFORMATION TECHNOLOGY & DECISION MAKING(2023)
摘要
Two-sided matching decision-making (TSMDM) problems widely exist in human being's life. In practical TSMDM problems, subjects who are being matched with usually tend to provide linguistic evaluations for convenience. Owing to the fuzziness of decision-making environment, subjects may provide several linguistic terms associated with their probabilities as the evaluations, which can be described as the probabilistic linguistic term sets (PLTSs). To model such scenarios, this paper proposes a TSMDM approach with probabilistic linguistic evaluations. The probabilistic linguistic evaluations are firstly normalized with the preservation of ignored probabilities. The normalized evaluations are then aggregated into the comprehensive evaluations by using the defined Choquet integral-based probabilistic linguistic aggregation (CIPLA) operator. Thereafter, the satisfaction degrees of two sides of subjects are calculated based on the closeness coefficient in TOPSIS method. On the basis of this, a multi-objective TSMDM model aiming to maximize the comprehensive satisfaction degree is built and transformed into a single-objective TSMDM model considering the weights of two sides. To solve the single-objective TSMDM model is to determine the optimal matching result. An illustrative example of matching enterprises and knowledge-sender universities is introduced to validate the proposed approach and compare it with other existing TSMDM approaches. The results illustrate that the proposed approach can not only avoid information loss, but also effectively integrate PLTSs with correlative criteria.
更多查看译文
关键词
Probabilistic linguistic term sets, two-sided matching decision-making, information fusion, optimal model
AI 理解论文
溯源树
样例
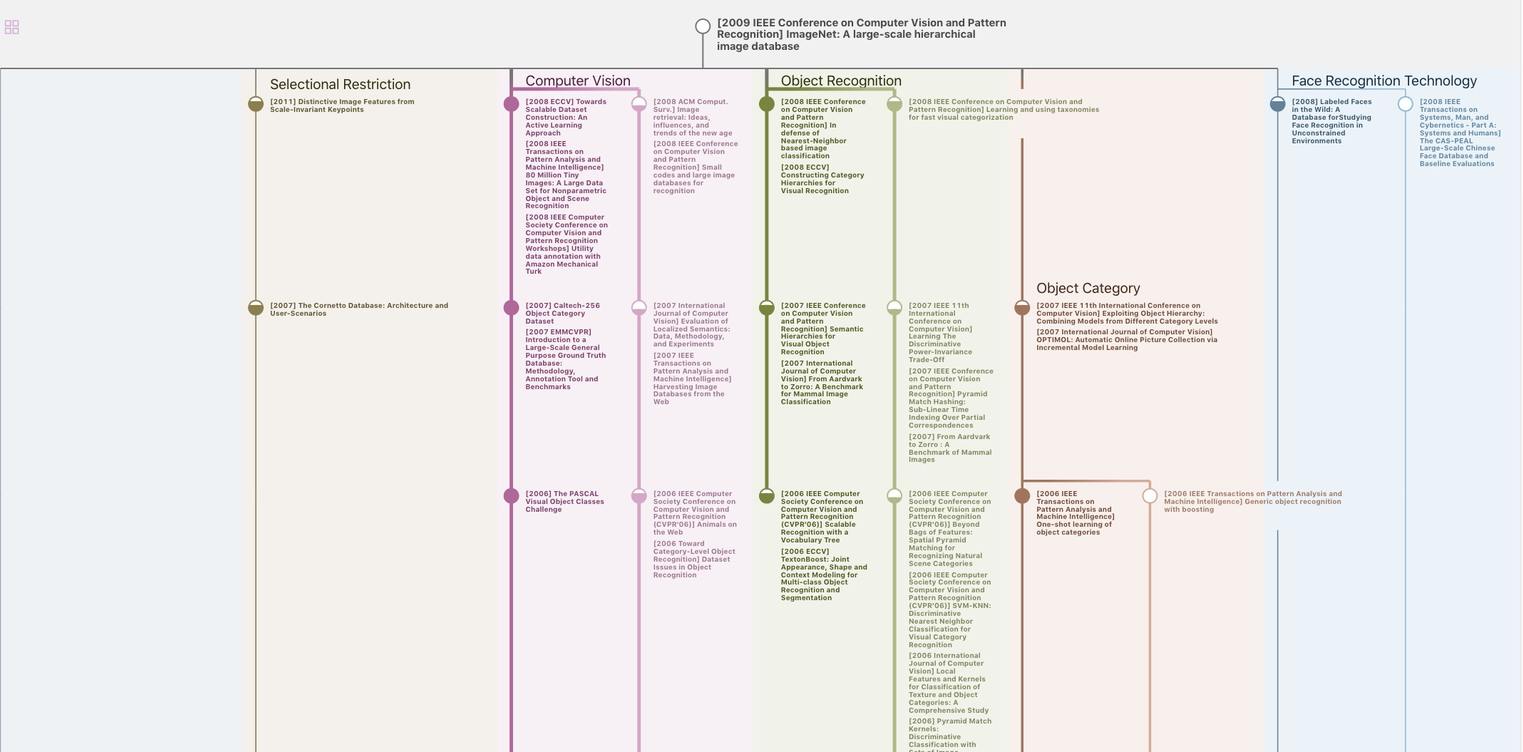
生成溯源树,研究论文发展脉络
Chat Paper
正在生成论文摘要