Multi-modal traffic event detection using shapelets
NEURAL COMPUTING & APPLICATIONS(2022)
摘要
Traffic management continues to be one of the most critical challenges facing smart cities. Timely detection of incidents plays an important role in reducing fatality rates, avoiding congestion and improving traffic conditions. Currently, traditional traffic event detection approaches often rely on one source of data, such as road sensor readings or social media posts. However, there is a need for new approaches that can combine these channels and benefit from the diversity of the collected data for better event detection performance. This paper presents a new framework for near real-time event detection based on the fusion of sensor readings and social media data. The shapelets technique, used for sensor readings, generates sub-sequences of the time series representing distinctive patterns. Each pattern is called a shapelet and is selected based on the maximal differentiation achieved between the different classes of a time series set. In traffic events, shapelets can represent patterns of incidents/congestion as well as normal traffic situations that the framework utilizes to detect the occurrence of events. Similarly, social media posts can be featured as shapelets to enable the combination of both media channels creating a multi-modal solution. In the proposed framework, two pipelines are defined : sensor readings detection pipeline and social media detection pipeline . In addition, two multi-modal fusion techniques based on Shapelet Transform are suggested and compared, namely early fusion and late fusion . They help in combining the two pipelines either at the data level or at the decision level. Validation using the M25 London Circular road data shows that early fusion of both sources has better detection rate and better performance over late fusion.
更多查看译文
关键词
Event detection, Multi-modal, Automatic incident detection, Traffic, Shapelet transform, Time series classification
AI 理解论文
溯源树
样例
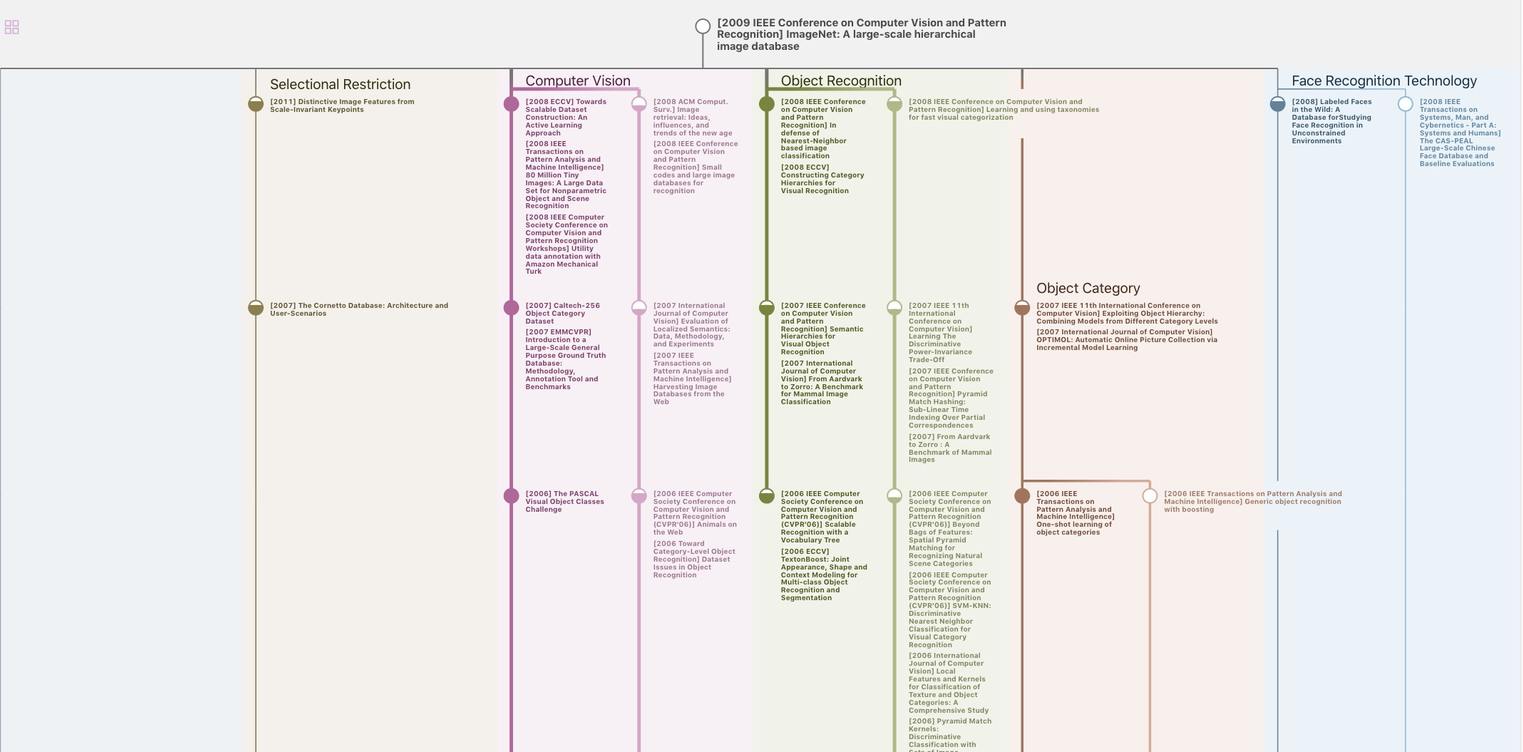
生成溯源树,研究论文发展脉络
Chat Paper
正在生成论文摘要