Robust semi-supervised data representation and imputation by correntropy based constraint nonnegative matrix factorization
Applied Intelligence(2022)
摘要
Many methods have been proposed recently for high-dimensional data representation to reduce the dimensionality of the data. Matrix Factorization (MF) as an efficient dimension-reduction method is increasingly used in a wide range of applications. However, these methods are often unable to handle data with missing entries. In a Semi-Supervised Learning (SSL) scenario, many commonly used missing value imputation methods, e.g., KNN imputation, cannot utilize the existing information on the labels, which is one of the most discriminative information in the data. Considering the outliers in the observed entries, in this paper, we propose an algorithm called Correntropy based Constraint Nonnegative Matrix Factorization Completion (CCNMF) for simultaneous construction of robust representation and imputation of high-dimensional data in an SSL scenario. Specifically, the Maximum Correntropy Criterion (MCC) is used to construct the model of the CCNMF method to alleviate the negative effects of non-Gaussian noise and outliers in the data. To solve the optimization problem, an iterative algorithm based on a Fenchel Conjugate (FC) and Block Coordinate Update (BCU) framework is proposed. We show that the proposed algorithm can satisfy not only objective sequential convergence but also iterate sequence convergence. The experiments are conducted on the real-world image dataset and community health dataset. In many cases, it is shown that the proposed method outperforms several state-of-the-art methods for both representation and imputation.
更多查看译文
关键词
Data representation, Missing data, Non-negative matrix factorization, Maximum correntropy criterion, Semi-supervised learning
AI 理解论文
溯源树
样例
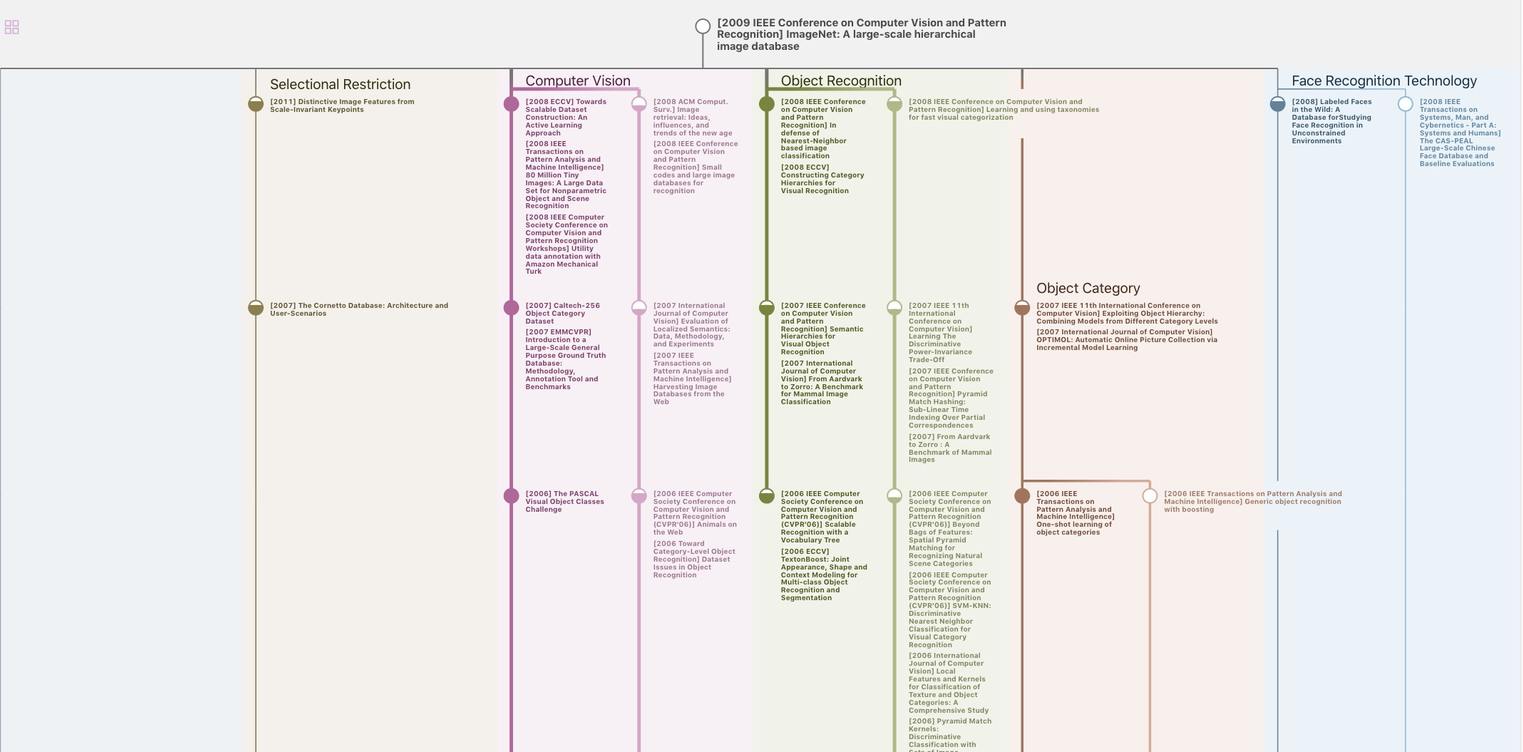
生成溯源树,研究论文发展脉络
Chat Paper
正在生成论文摘要