A compact and robust perceptual hashing function through self-supervised learning
2022 11th Mediterranean Conference on Embedded Computing (MECO)(2022)
摘要
Thanks to the Internet, it is easy to share and redis-tribute multimedia items for different purposes. However, to avoid malicious usage of sensitive data, it is desirable to have efficient ways to track and identify relevant items. A hash-based content identification system uses a robust perceptual hashing function to generate a short binary string to match query multimedia items with a set of relevant assets in a database. In low consumption systems, such as in IoT applications, the properties of this function are crucial to reducing the network bandwidth consumption to communicate with a server and efficiently compute the binary identifier with limited computing power. Moreover, it still requires to be adaptive to new scenarios. In this work, we propose to exploit the feature learning capabilities of convolutional neural networks to learn automatically a perceptual hashing function invariant against a set of expected manipulations. To achieve this, we define a compact and efficient architecture and a self-supervised learning pretext task that enforces a perceptual hashing algorithm's robustness and discrimination properties. We evaluate this approach against 15 manipulations with a comprehensive set of intensities in different datasets, achieving high robustness and identification performance.
更多查看译文
关键词
perceptual hashing,content identification,self-supervised learning,deep learning,computer vision
AI 理解论文
溯源树
样例
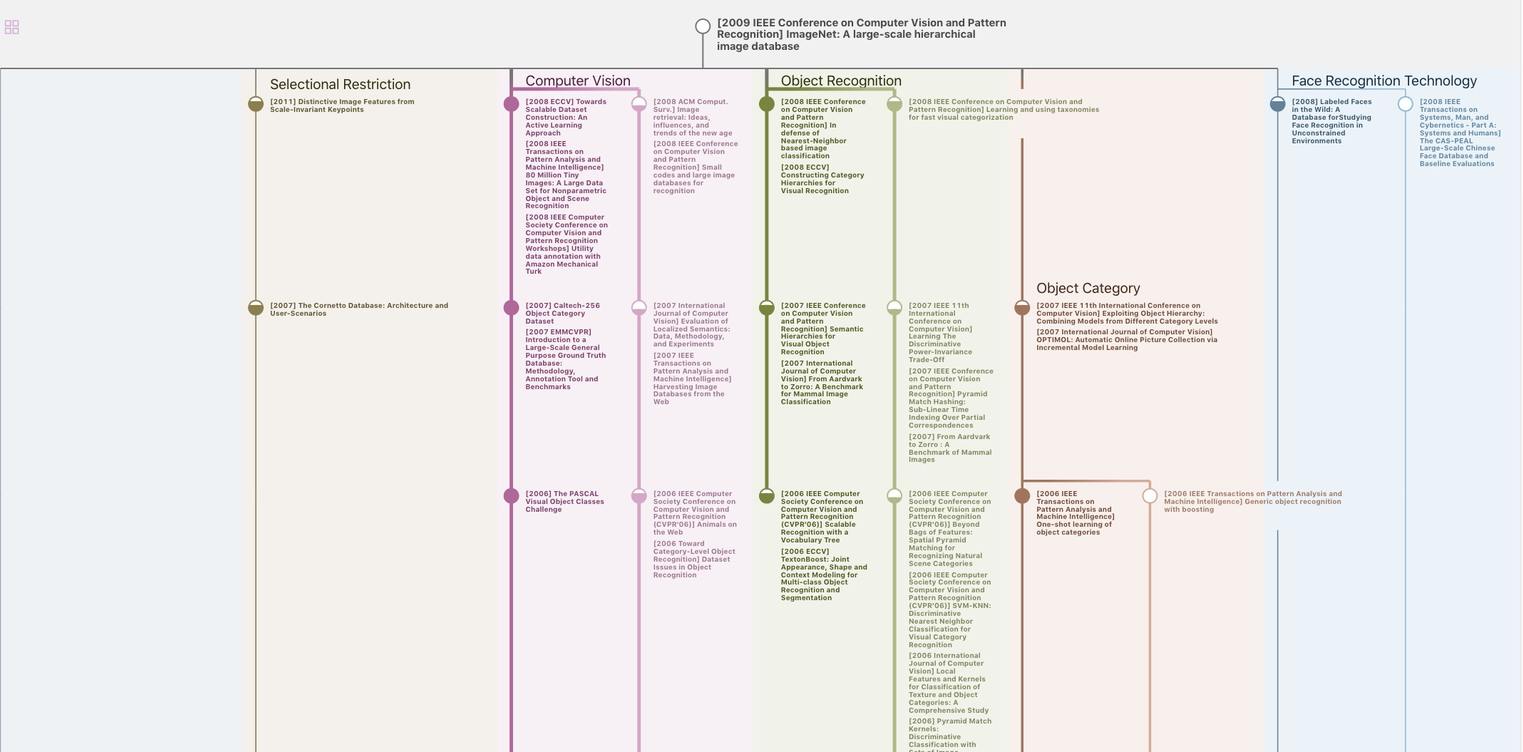
生成溯源树,研究论文发展脉络
Chat Paper
正在生成论文摘要