ORAD: a new framework of offline Reinforcement Learning with Q-value regularization
EVOLUTIONARY INTELLIGENCE(2024)
摘要
Offline Reinforcement Learning (RL) defines a framework for learning from previously collected static buffer. However, offline RL is prone to approximation errors caused by out-of-distribution (OOD) data and particularly inefficient for pixel-based learning tasks compared with state-based input control methods. Several pioneer efforts have been made to solve this problem; some use pessimistic Q-values approximation for unseen observation while others train a model to simulate the environment to train a model on previously collected data to learn policies. However, these methods require accurate and time-consuming estimation of the Q-values or the environment models. Based on this observation, we present offline RL methods with augmented data (ORAD), a handy but non-trivial extension to offline RL algorithms. We show that simple data augmentations, e.g. random translation and random crop, significantly elevate the performance of the state-of-the-art offline RL algorithms. Besides, we find that regularization of the Q-values can also enhance performance. Extensive experiments on the pixel-based input control-Atari demonstrate the superiority of ORAD over SOTA offline RL methods considering both performance and data efficiency, and reveal that ORAD is more effective for the pixel-based control.
更多查看译文
关键词
Offline reinforcement learning,Q-value regularization,Q-value approximation,Data augmentation,Pixel-based control
AI 理解论文
溯源树
样例
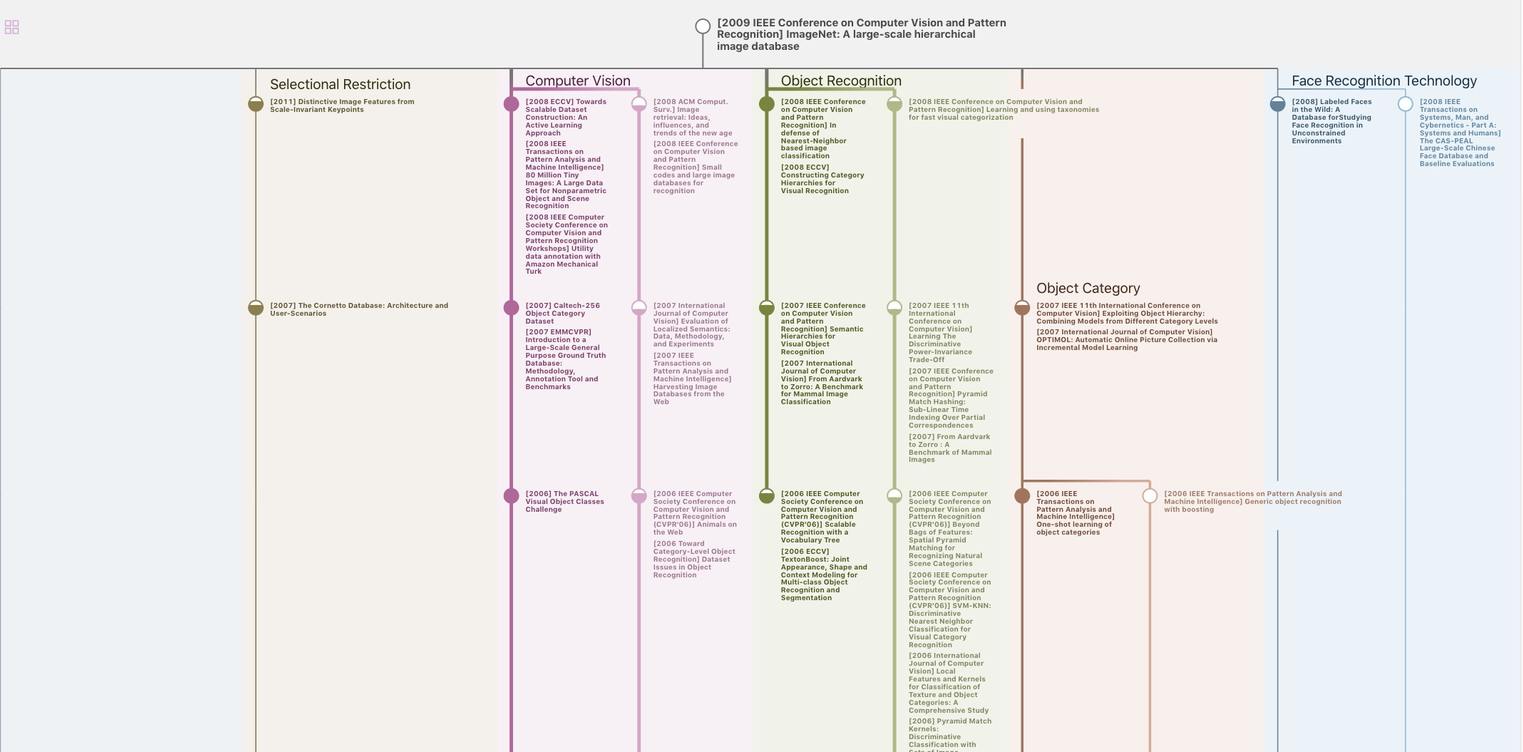
生成溯源树,研究论文发展脉络
Chat Paper
正在生成论文摘要