A Hybrid Learning Framework for Imbalanced Classification
INTERNATIONAL JOURNAL OF INTELLIGENT INFORMATION TECHNOLOGIES(2022)
摘要
Class imbalance is a well-known and challenging algorithmic research topic among the machine learning community as traditional classifiers generally perform poorly on imbalanced problems, where data to be learned have skewed distributions between their classes. This paper presents a hybrid framework named PRUSBoost for learning imbalanced classification. It combines a selective data under-sampling procedure and a powerful boosting strategy to effectively enhance classification performance on imbalanced problems. Different from the simple random under sampling algorithm, this framework constructs the training data of the majority or negative class by using a newly developed partition based under sampling approach. Experiments on several datasets from different application domains that carry skewed class distributions have shown that the proposed framework provides a very competitive, consistent, and effective solution to imbalanced classification problems.
更多查看译文
关键词
Boosting, Data Sampling, Imbalanced Classification, Machine Learning, Novelty Detection
AI 理解论文
溯源树
样例
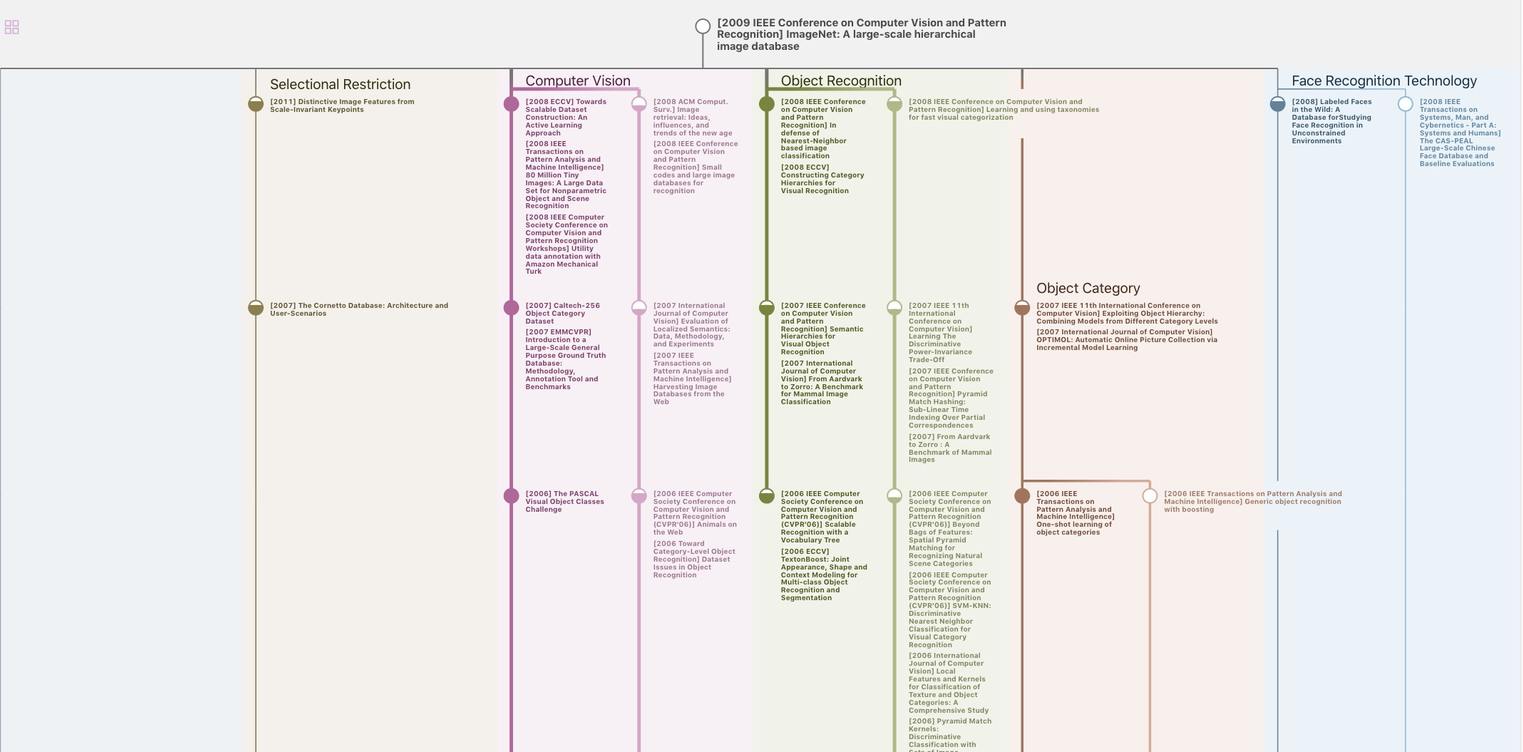
生成溯源树,研究论文发展脉络
Chat Paper
正在生成论文摘要