Particle-balanced context-based filtering for hypothesis maintenance in sparse sensor coverage situations
2022 25th International Conference on Information Fusion (FUSION)(2022)
摘要
In this paper, a method for ensuring the maintenance of multiple hypotheses in the presence of context data is proposed. In many practical context-based tracking problems where particle filtering is used, the filtering distribution is distinctly multimodal. Several of the state hypotheses may be lost owing to resampling of a finite number of particles, when the target leaves sensor coverage for several timesteps. This is especially the case where there is no sensor coverage in areas of the state space where particle density is low, and tracking is confined to narrow pathways, such as narrow roads and alleyways. The approach followed in this paper is to cluster particles into hypotheses using expectation maximisation of a multivariate Gaussian mixture, and to ensure that the number of particles per cluster is maintained using modified resampling. When no measurements are received for extended periods, two criteria are used to modify resampling to ensure hypothesis maintenance. This first adjusts resampling probabilities such that each hypothesis or cluster has roughly the same number of particles. The second adjusts resampling probabilities such that each hypothesis or cluster has a number of particles proportional to the narrowest dimension of the cluster (minimum eigenvalue of the cluster). This ensures that the particle density of each hypothesis remains roughly the same over all the hypotheses. The particular application will dictate which criterion is the most suitable.
更多查看译文
关键词
Context based tracking,particle filtering,hypothesis maintenance,sparse sensor coverage
AI 理解论文
溯源树
样例
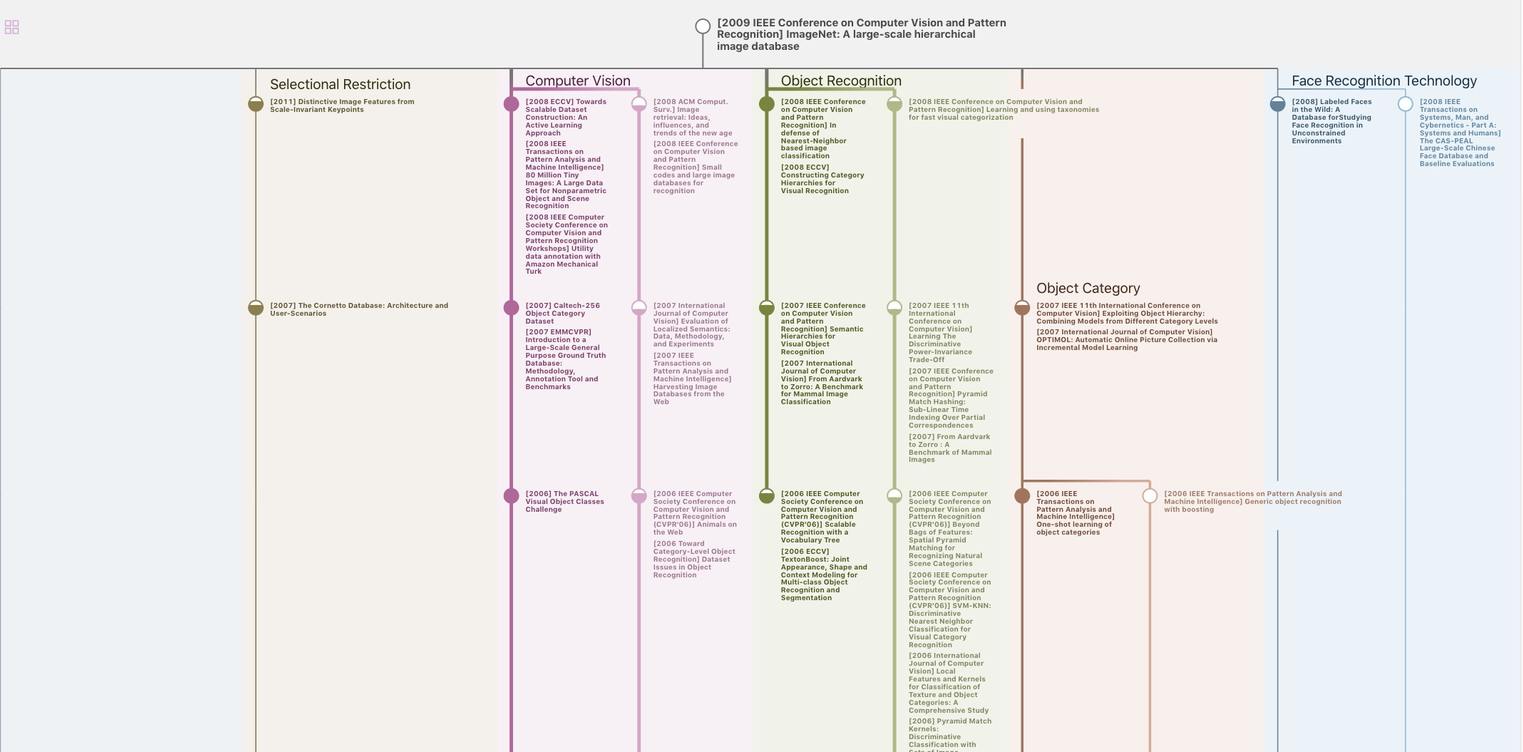
生成溯源树,研究论文发展脉络
Chat Paper
正在生成论文摘要