Machine Learning-Based Security Solutions for Critical Cyber-Physical Systems
2022 10th International Symposium on Digital Forensics and Security (ISDFS)(2022)
摘要
Cyber-Physical Systems(CPS) are complex critical infrastructure that assists society and provides efficient services to the people and governments. CPS uses many technologies including industrial control systems, smart grid, smart metering systems and the Industrial Internet of Things(IIoT). Extensive usage of ICT, giant physical components, and interconnected nature makes them extremely vulnerable to physical and cyber threats. A cyber-attack on a smart manufacturing system may halt the overall manufacturing process of the industry and reason to stop/reduce the production extensive time. Traditional security systems such as signature-based intrusion detection systems, firewalls and blacklisting are not effective due to high false alarm rates. Cyber-attacks such as DoS, DDoS, zero-day attacks and advanced persistent threats are advanced threats to CPS complex infrastructures. This paper discusses the current and future security challenges associated with CPS, datasets, and the impact of Machine Learning (ML) techniques proposed/used to detect and protect CPS from cyber-attacks. Numerous ML techniques such as unsupervised anomaly detection, Support Vector Machines (SVM), deep belief networks, recurrent neural networks and convolutional neural networks (CNN) have been proposed in the literature to mitigate risks for the critical CPS.
更多查看译文
关键词
Index Terms - Critical Cyber-physical systems,Cyber Attacks,Cybersecurity,Machine Learning,Deep Learning
AI 理解论文
溯源树
样例
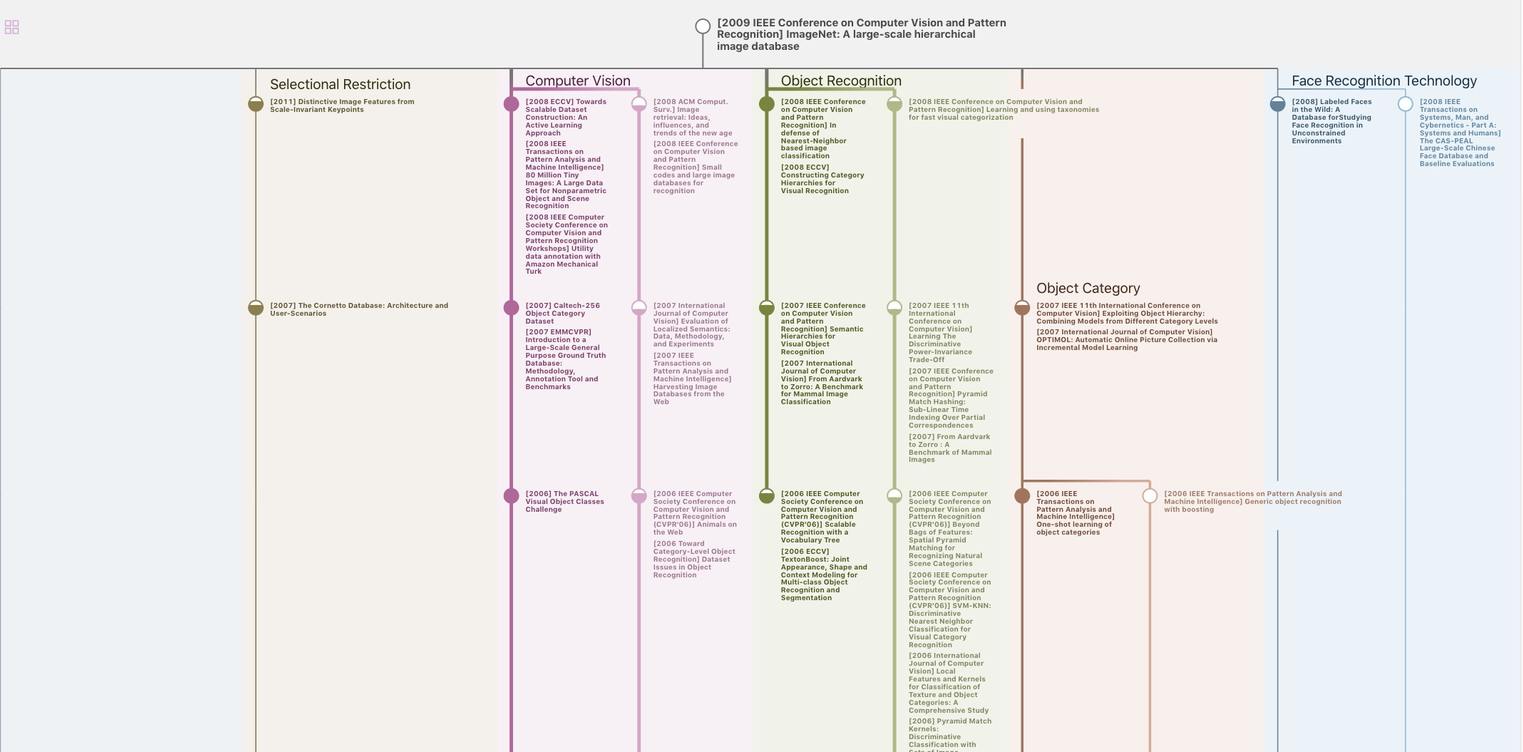
生成溯源树,研究论文发展脉络
Chat Paper
正在生成论文摘要