Generalized attention-based deep multi-instance learning
Multimedia Systems(2022)
摘要
Attention-based deep multi-instance learning (MIL) is an effective and interpretable model. Its interpretability is attributed to the learnability of its inner attention-based MIL pooling. Its main problem is to learn a unique instance-level target concept for weighting instances. Another implicative issue is to assume that the bag and instance concepts are located in the same semantic space. In this paper, we relax these constraints as: (i) There exist multiple instance concepts; (ii) The bag and instance concepts live in different semantic spaces. Upon the two relaxed constraints, we propose a two-level attention-based MIL pooling that first learns several instance concepts in a low-level semantic space and subsequently captures the bag concept in a high-level semantic space. To effectively capture different types of instance concepts, we also present a new similarity-based loss. The experimental results show that our method achieves higher or very comparable performance with state-of-the-art methods on benchmark data sets and surpasses them in terms of performance and interpretability on a synthetic data set.
更多查看译文
关键词
Multi-instance learning,Deep learning,Similarity-based loss,Attention-based pooling,Interpretability
AI 理解论文
溯源树
样例
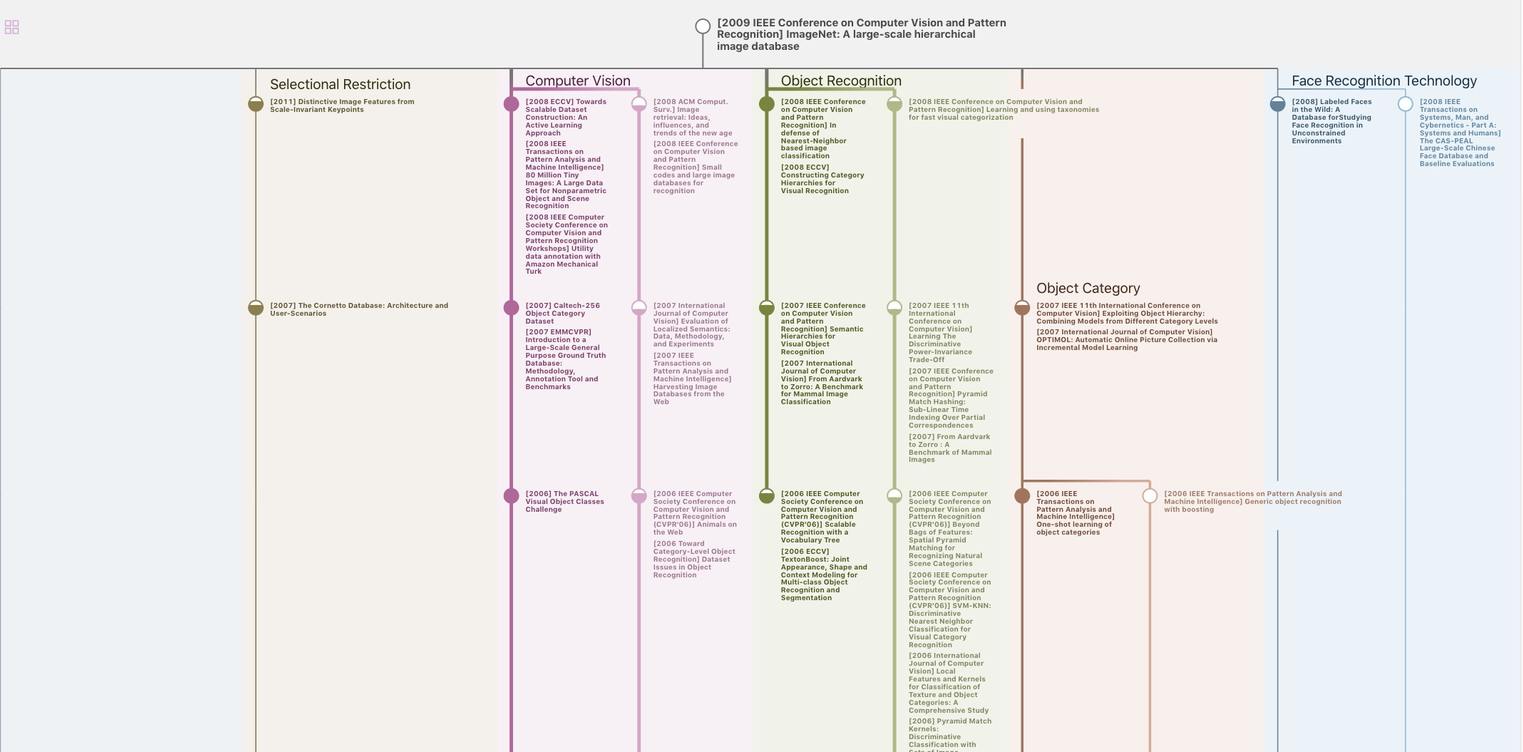
生成溯源树,研究论文发展脉络
Chat Paper
正在生成论文摘要