Semi-supervised Feature Selection Based on Pairwise Constraint-Guided Dual Space Latent Representation Learning and Double Sparse Graphs Discriminant
Applied intelligence(2022)
Abstract
Semi-supervised graph based sparse learning investigates both label related information and inherent characteristic in data when only a small number of labels are available. How to fully explore the structure information in data space and feature space and well utilize the label information in the partial labeled data is the crux to improve the performance of a learning model. In this study, the latent representation learning is introduced into data space and feature space (dual space) simultaneously in the framework of the semi-supervised graph lased sparse learning. A novel semi-supervised feature selection algorithm called semi-supervised feature selection based on pairwise constraint-guided dual space latent representation learning and double sparse graphs discriminant (PCDLRD) is proposed. Latent representation learning is firstly used in semi-supervised feature selection considering dual graph information, which aims to capture the complex internal correlation structure information in data space and feature space and well guide the process of feature selection. Furthermore, the latent representation learning with the pairwise constraints is defined, which embeds the pairwise constraints into the latent representation learning in data space so as to fully exploit the intrinsic structure of the data in the help of some supervised information. A double sparse graph discriminative model is also integrated into the framework to enhance the discriminative ability of the method, which uses both labeled and unlabeled data. In addition, a robust dual space latent representation learning model based on L2,1 − norm is established, which is more robust to outliers than the squared norm. A unified solution for the objective function is investigated and its convergence is proved theoretically and experimentally. Experimental results on twelve public datasets validate the viability of the proposed method and the effectiveness of the proposed method in classification tasks compared to the other six feature selection methods.
MoreTranslated text
Key words
Semi-supervised feature selection,Pairwise constraint,Dual space latent representation,Sparse graph discriminative
AI Read Science
Must-Reading Tree
Example
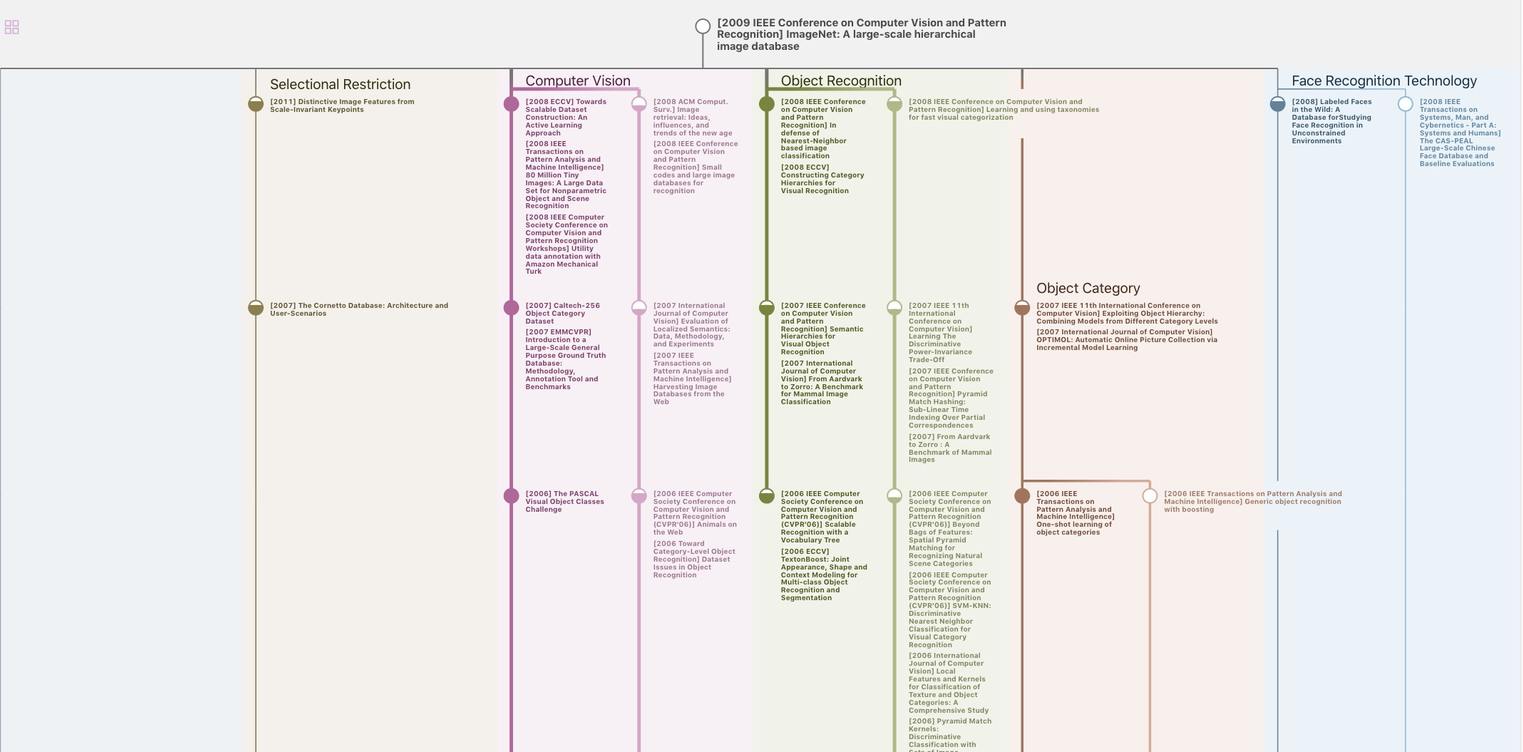
Generate MRT to find the research sequence of this paper
Chat Paper
Summary is being generated by the instructions you defined