Refragmentation Through Machine Learning Classifier for Fast and Accurate Optical Proximity Correction
DTCO AND COMPUTATIONAL PATTERNING(2022)
摘要
MB-OPC contains a fragmentation step, in which each polygon edge is divided into a number of segments. Simple empirical rules dictate how the edges should be divided. Fragmentation strongly affects MB-OPC in its quality and runtime since OPC correction is performed segment by segment. Refragmentation using random forest classifier (RFC) is proposed. A segment and its surroundings are modeled using a number of features, which drive RFC to decide whether a segment should be further divided or not. It complements rule-based fragmentation in that small number of critical segments, which are not short enough and cause longer MB-OPC iterations, are quickly identified. When refragmentation is applied in standard rule-based fragmentation and MB-OPC flow, maximum EPE is reduced substantially (from 3.8nm to 2.4nm) with very marginal increase in the number of segments (7k to 7,093), yet OPC iterations are reduced (10 down to 8).
更多查看译文
关键词
Refragmentation, random forest classifier
AI 理解论文
溯源树
样例
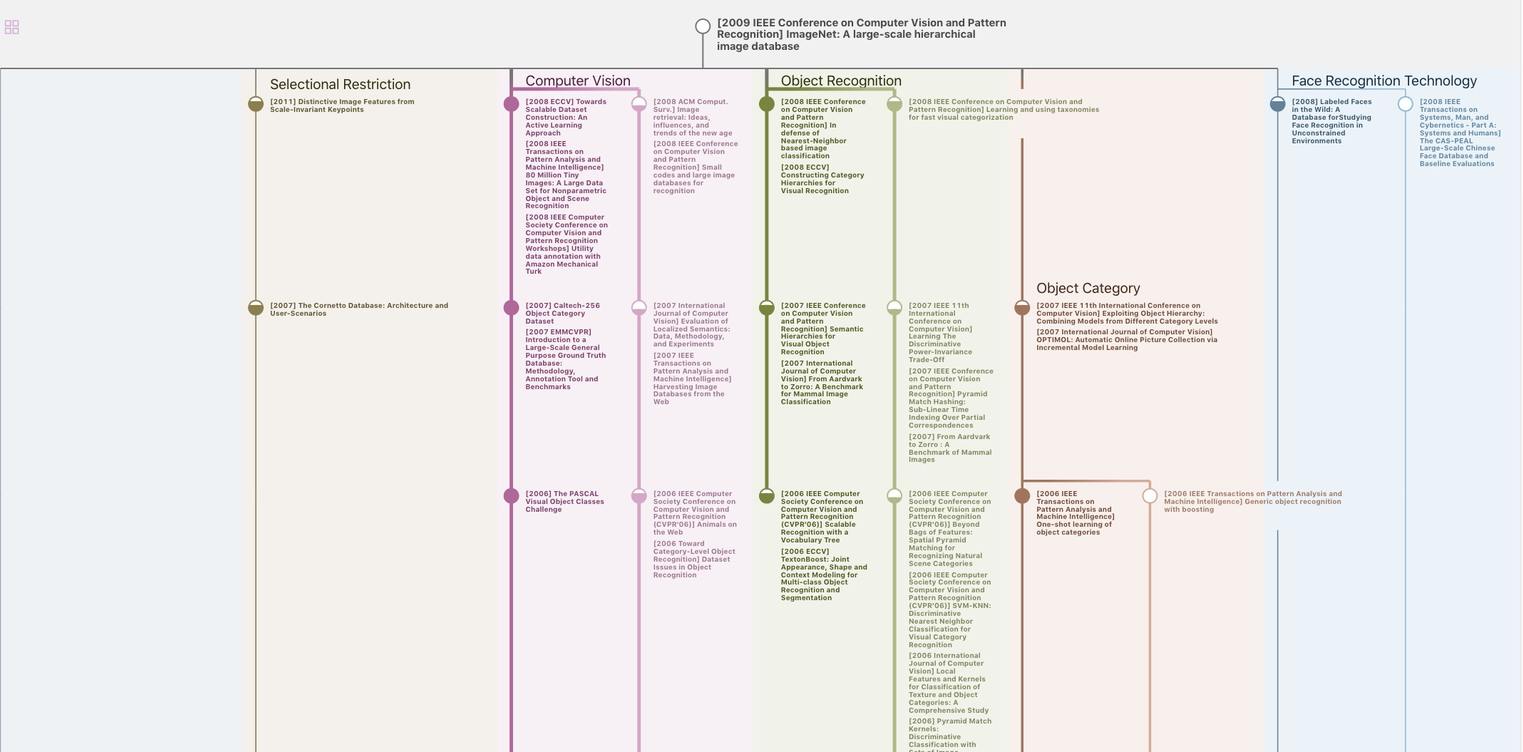
生成溯源树,研究论文发展脉络
Chat Paper
正在生成论文摘要