Roles of Drop Size Distribution and Turbulence in Autoconversion Based on Lagrangian Cloud Model Simulations
JOURNAL OF GEOPHYSICAL RESEARCH-ATMOSPHERES(2022)
摘要
The roles of the drop size distribution (DSD) and turbulence in the autoconversion rate A are investigated by analyzing Lagrangian cloud model (LCM) data for shallow cumulus clouds. The correlations of DSD and turbulence with other cloud parameters are estimated, and they are applied to parameterize their effects on A. A new parameterization of A based on this analysis is proposed: A = alpha q(c)(7/3) N-c(-1/3) H(R-c - R-c0) with alpha = alpha N-c(-X) (R-c - R-c0) (1 + b epsilon), where q(c) is the cloud water mixing, N-c ratio, R-c and are the number concentration and the volume mean radius of cloud droplets, R-c0 is the dissipation e rate, R-c0 is the threshold value R-c of, H is the Heaviside step function, and X, a, and b are constants. Here, N-c(-X) (R-c - R-c0) represents the effect of DSD via its correlation with N-c and R-c while A proportional to q(c)(7/3) N-c(-1/3) represents the effect of gravitational collisional growth for a given DSD and turbulence. The correlation between turbulence and DSD makes b larger than expected from turbulence-induced collision enhancement only. The effects of DSD and turbulence and their correlations with q(c) and N-c explain a wide range of exponent values of and in many existing parameterizations of A. The new parameterization is compared with the LCM data and applied to a bulk cloud model (BCM) while clarifying the difference between the cloud droplet mixing processes in the LCM and BCM. The importance of DSD and turbulence in raindrop formation in shallow cumulus clouds is shown by comparing the A results with and without these effects. Plain Language Summary One of the most important aspects of numerical weather prediction is the prediction of how much precipitation is generated from cloud droplets within a cloud; this process is referred to as autoconversion. Although autoconversion is known to be determined by the mass and number concentration of cloud droplets, it can also be affected by other factors, such as the drop size distribution (DSD) and turbulence. We clarify the effects of DSD and turbulence on autoconversion by analyzing the results of a realistic cloud field simulated by a new type of cloud model, in which cloud droplets are simulated as Lagrangian particles, and propose a parameterization to predict the autoconversion rate. It is found that the correlations of DSD and turbulence with other cloud parameters must be considered for the parameterization of their effects in autoconversion. We also apply the new parameterization to numerical weather prediction and show that the DSD and turbulence play an important role in raindrop formation in shallow cumulus clouds.
更多查看译文
AI 理解论文
溯源树
样例
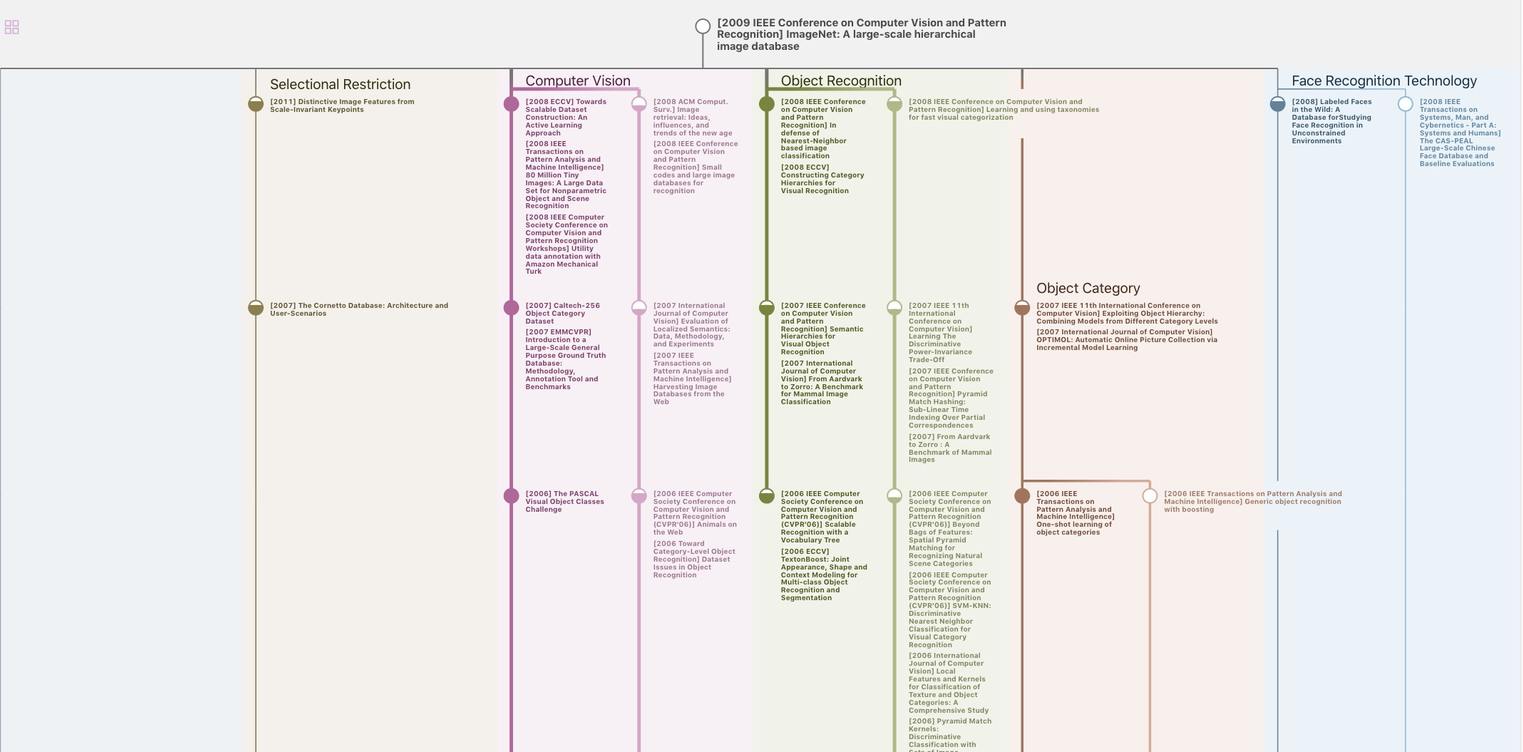
生成溯源树,研究论文发展脉络
Chat Paper
正在生成论文摘要