Generalized and Robust Least Squares Regression.
IEEE transactions on neural networks and learning systems(2024)
摘要
As a simple yet effective method, least squares regression (LSR) is extensively applied for data regression and classification. Combined with sparse representation, LSR can be extended to feature selection (FS) as well, in which l1 regularization is often applied in embedded FS algorithms. However, because the loss function is in the form of squared error, LSR and its variants are sensitive to noises, which significantly degrades the effectiveness and performance of classification and FS. To cope with the problem, we propose a generalized and robust LSR (GRLSR) for classification and FS, which is made up of arbitrary concave loss function and the l2,p -norm regularization term. Meanwhile, an iterative algorithm is applied to efficiently deal with the nonconvex minimization problem, in which an additional weight to suppress the effect of noises is added to each data point. The weights can be automatically assigned according to the error of the samples. When the error is large, the value of the corresponding weight is small. It is this mechanism that allows GRLSR to reduce the impact of noises and outliers. According to the different formulations of the concave loss function, four specific methods are proposed to clarify the essence of the framework. Comprehensive experiments on corrupted datasets have proven the advantage of the proposed method.
更多查看译文
关键词
Optimization,Robustness,Feature extraction,Sparse matrices,Noise measurement,Task analysis,Iterative methods,Classification,feature selection (FS),generalized framework,linear regression,robust
AI 理解论文
溯源树
样例
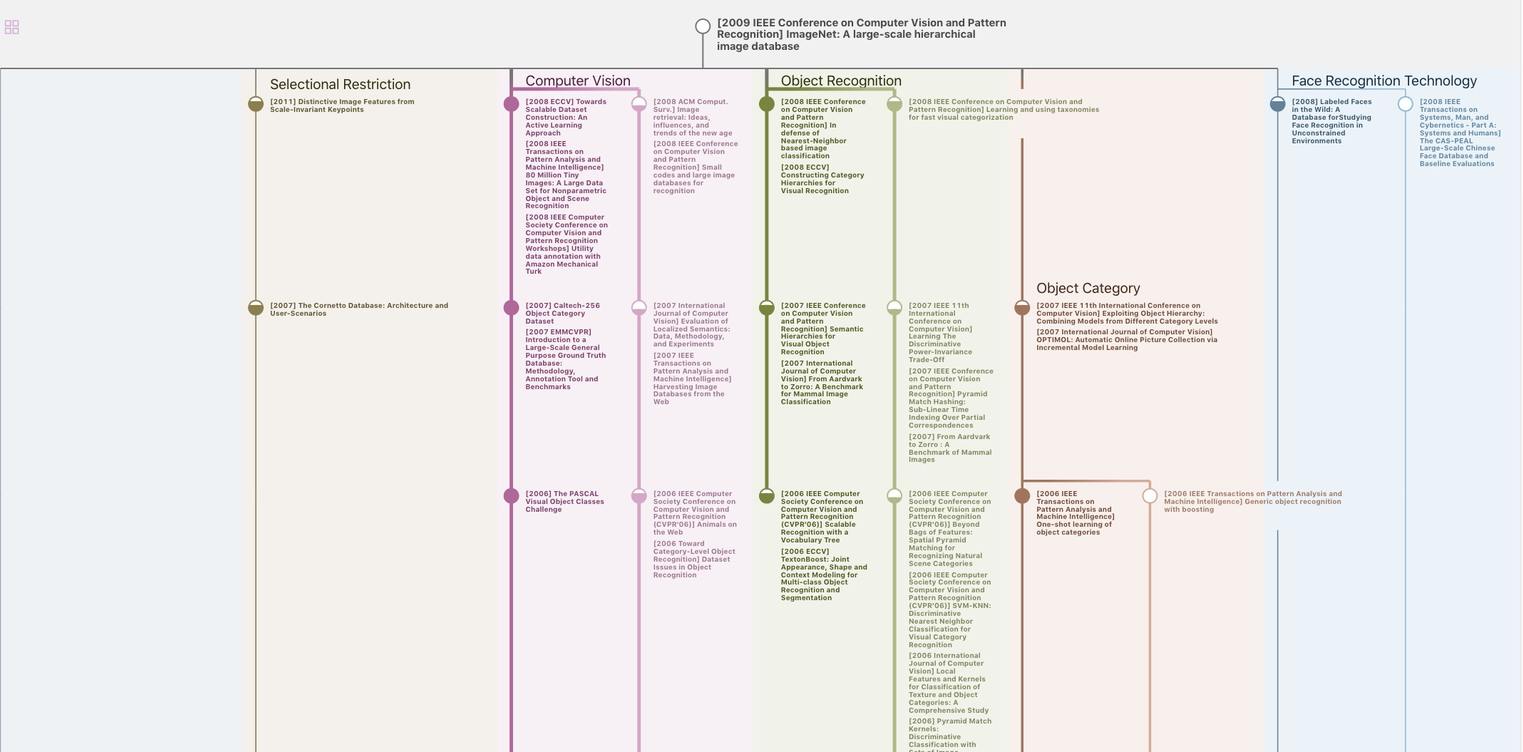
生成溯源树,研究论文发展脉络
Chat Paper
正在生成论文摘要