Learning deep latent space for unsupervised violence detection
MULTIMEDIA TOOLS AND APPLICATIONS(2022)
摘要
Numerous violent actions occur in the world every day, affecting victims mentally and physically. To reduce violence rates in society, an automatic system may be required to analyze human activities quickly and detect violent actions accurately. Violence detection is a complex machine vision problem involving insufficient violent datasets and wide variation in activities and environments. In this paper, an unsupervised framework is presented to discriminate between normal and violent actions overcoming these challenges. This is accomplished by analyzing the latent space of a double-stream convolutional AutoEncoder (AE). In the proposed framework, the input samples are processed to extract discriminative spatial and temporal information. A human detection approach is applied in the spatial stream to remove background environment and other noisy information from video segments. Since motion patterns in violent actions are entirely different from normal actions, movement information is processed with a novel Jerk feature in the temporal stream. This feature describes the long-term motion acceleration and is composed of 7 consecutive frames. Moreover, the classification stage is carried out with a one-class classifier using the latent space of AEs to identify the outliers as violent samples. Extensive experiments on Hockey and Movies datasets showed that the proposed framework surpassed the previous works in terms of accuracy and generality.
更多查看译文
关键词
Action recognition, Human behavior analysis, Human action recognition, Violence detection, Security and surveillance
AI 理解论文
溯源树
样例
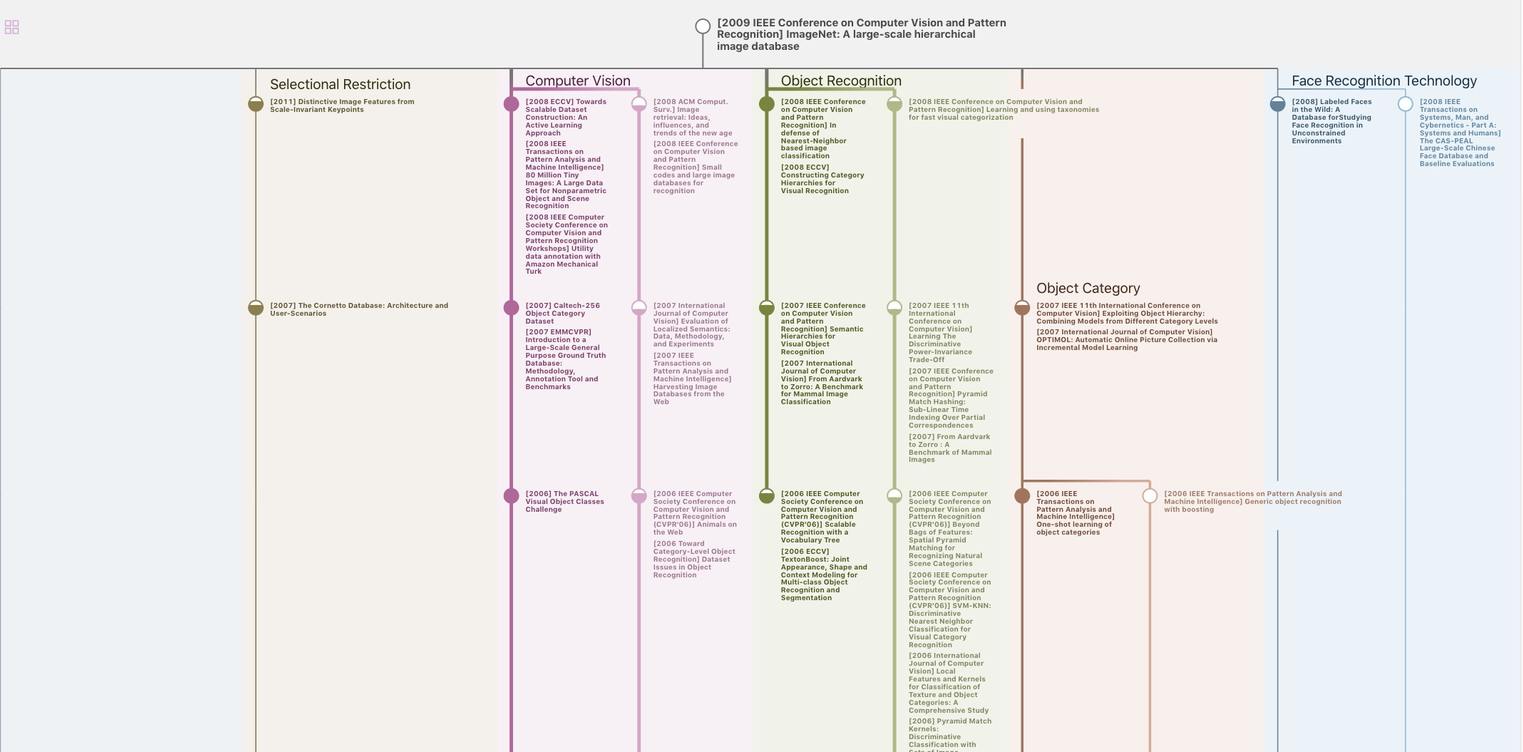
生成溯源树,研究论文发展脉络
Chat Paper
正在生成论文摘要